1. 引言
由于潜射武器通常都是长期贮存、一次使用的,所以贮存阶段是影响潜射武器安全性的主要阶段。在贮存阶段,由于水下环境恶劣,所以温度、湿度、振动等环境因素是影响潜射武器的安全性的主要因素。潜射武器的安全性不仅对武器本身具有重要影响,而且对潜艇的安全性具有重大影响。目前,对潜射武器的安全性控制主要集中在安全性分析和安全性评估,这两种方法对于潜射武器的安全控制具有重要作用,但是这两种方法都是对武器系统固有的安全因素进行分析评估,却难以对武器系统的安全性进行预测。如果能及时准确的预测潜射武器的安全性,及时采取安全性控制措施,将对提高潜射武器的安全性具有重要作用。
目前有关装备安全性预测方法主要包括神经网络方法[1] [2] 、系统仿真方法[3] ,贝叶斯网络方法[4] [5] 等。但是上述方法都没有利用环境因子进行安全性预测,而且上述方法的泛化能力有限,所以不能保证安全性预测的精度。
多核学习是近年来机器学习领域的研究热点,其在模式分类、模式回归及预测等方面具有广泛应用[6] 。局部多核学习方法(LMKL)[7] 是一种泛化能力较好的多核学习方法,本文采用LMKL对潜射武器环境因子的安全性进行预测。
2. 局部多核学习方法(LMKL)
核学习方法已经广泛应用于统计与机器学习领域,而多核学习由于具有良好的泛化能力,逐渐成为核学习领域的重要分支。
多核学习对于处理不规则数据、样本分布不平坦等实际问题具有很好的效果[6] 。多核学习方法是一种权系数与核函数的组合学习算法[7] ,其流程如图1所示。
为了提高回归函数的预测精度,Sarath[7] 等提出了一种局部多核学习方法(LMKL),每个核函数的加权值根据数据点确定,其加权核为:
(1)
其中
为输入
的门限函数:
(2)
其中
为
的参数,可以用梯度下降法求得,具体请参考文献[8] 。优化问题为:
(3)
(4)
最终回归函数为:
(5)
3. 潜射武器环境安全性预测模型
在潜射武器贮存阶段,环境因素是影响武器系统安全的重要因素,其重要性如下表所示[9] 。
从表1可以看出,贮存中最重要的环境因素是温度、湿度,其次是冲击。环境安全性预测模型选择影响武器系统安全性较大的因素作为输入,包括温度、湿度。模型的输出为武器系统安全性预测结果,具体判断规则如表2所示。
温度归一化模型:
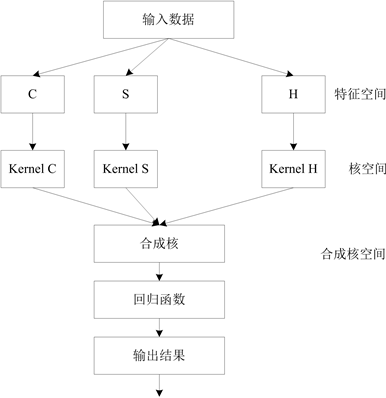
Figure 1. Multiple kernel leaning flow chart
图1. 多核学习流程图
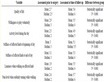
Table 1. Important environment factors to weapon system in storage stage
表1. 贮存阶段有关的重要环境因素
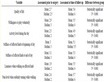
Table 2. Decision rules of environment safety predicting
表2. 环境安全性预测判断规则
(6)
式中:
为温度归一化值;
为实际温度,
为艇上最小温度,
为艇上最高温度,假定武器系统存贮最高温度35℃,最低温度5℃。
湿度
取值为实际相对湿度,假设武器系统安全性预测值为
,则环境安全性预测模型为:
(7)
式中:
为时间序列值;
为局部多核学习模型。
4. 仿真验证
根据武器系统使用环境要求以及上述分析结果,可得出如表3所示的安全性规则。
这种规则是无法直接用于LMKL的,需要对上表内容进行量化处理,使之成为可用于训练的量化样本。本文采用蒙特卡洛方法,根据上表内容生成虚拟样本,样本示例如表4所示。
本文采用4个如下的径向基核函数作为LMKL的核函数:
(8)
每一个核函数代表1种环境信息,核函数方差
。为了减少由于随机现象以及主观经验引起的不确定性,实验独立进行了30次,并与神经网络集成(NNE)[10] 、最小二乘SVM (LSSVM)做了试验对比。神经网络集成的集成规模为5,个体模型为RBF神经网络;支持向量机核函数为RBF核函数,惩罚因子C = 2000。仿真结果见表5。
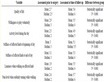
Table 3. Weapon system safety rules table of environment factors
表3. 武器系统环境安全性规则表
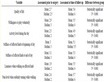
Table 4. Examples of training samples
表4. 训练样本示例
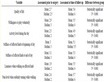
Table 5. Simulating experiment results
表5. 仿真实验结果
从表5可以得出,LKML与LSSVM的预测精度相当,但是LKML算法的支持向量数量最小,而且计算时间最短。从上述试验结果可以看出,本文提出的方法可以比较准确的根据环境信息对系统安全性进行预测,而且算法复杂性可以满足要求。
5. 结束语
从仿真结果可以看出,LMKL可以比较准确的预测潜射武器的安全性,而且且预测精度明显高于其他算法。但是,LMKL需要完善的训练样本才能保证预测的准确性。本文所述的安全性预测方法适用于长期贮存的武器系统。