1. 前言
细胞凋亡蛋白质是一类有着特殊功能的蛋白质,在生物体的生长发育和维持体内平衡中扮演着重要的角色 [1] 。这些蛋白质对于了解细胞凋亡的过程和机理具有重要作用。细胞凋亡与许多疾病相关,如自身免疫疾病、肿瘤、神经退行性病变等 [2] 。细胞凋亡蛋白质的功能与其亚细胞位置紧密相关 [3] ,从生物信息学的角度预测蛋白质在细胞中的位置能更好地了解它们的功能。
mRNA是RNA分子中的一大家族,它将DNA中的遗传信息传递到核糖体中,在核糖体上作为蛋白质的合成模板,决定肽链中氨基酸的排列顺序。研究表明mRNA的二级结构对研究其功能具有重要的作用 [4] 。本文将细胞凋亡蛋白质所对应的mRNA的二级结构信息挑选出来,并与蛋白质一级序列信息相结合,利用生物信息学的方法统计分析了结构和序列信息,将有利于更深刻地了解不同亚细胞位置中细胞凋亡蛋白质的特性。
本文以细胞凋亡蛋白质的mRNA序列,mRNA二级结构,氨基酸序列作为研究对象,统计分析了三阅读框3-mer mRNA序列频数信息,mRNA二级结构-序列模式信息,氨基酸的物理化学性质,氨基酸黏性和进化信息,用支持向量机的方法基于Jackknife检验和独立检验对不同亚细胞位置的细胞凋亡蛋白质进行预测。
2. 数据集
本文所采用的细胞凋亡蛋白质数据均来源于Uniprot数据库(Release 2015_12 http://www.Uniprot.org/)。根据关键词apoptosis挑选出1128个细胞凋亡蛋白质,其中多定位的蛋白质有555个,有明确唯一单一定位信息的蛋白质有572个。去掉蛋白质数量过少的亚细胞位置,在RefSeq数据库中查找到选定的单一定位的蛋白质的mRNA序列,同时去掉mRNA序列长度大于10,000 nt的蛋白质。最终采用的数据集共包含蛋白质331个,分别位于四个亚细胞位置:细胞核,细胞膜,细胞质和线粒体,见图1。数据集中每个蛋白质都有对应的mRNA序列。

Figure 1. Apoptosis protein data distribution diagram
图1. 凋亡蛋白质数据分布图
3. 特征选取
3.1. 三阅读框下3-mer mRNA序列信息
mRNA由DNA转录而来,携带着重要的遗传信息,其中最基本的信息就是序列信息。mRNA序列中包含了不同大小、形状和化学性质的四种碱基:胞嘧啶(C)、鸟嘌呤(G)、腺嘌呤(A)和尿嘧啶(U),3-mer序列信息是指序列中任意三个相邻的碱基。对于一条序列,由起始位点开始统计三联体,有三种阅读框(由W1,W2,W3表示),取一个细胞凋亡蛋白质(图1)对应的mRNA作为例子:
ATGTCGGGACCCGTGCCAAGCAGGGCCAGAGTTTACACAGATGTTAATACACACAGACCT......
第一个阅读框(W1),3-mer序列信息:ATG TCG GGA CCC GTG CCA AGC AGG GCC ......
第二个阅读框(W2),3-mer序列信息:TGT CGG GAC CCG TGC CAA GCA GGG CCA ......
第三个阅读框(W3),3-mer序列信息:GTC GGG ACC CGT GCC AAG CAG GGC CAG ......
任一序列的三阅读框3-mer序列频数表示为公式(1) (2)
(1)
(2)
其中
表示第k个阅读框中第i个三联体出现的频数。
3.2. mRNA二级结构–序列模式信息
研究表明,mRNA的功能与其结构密切相关,为了使mRNA结构信息可以作为机器学习的特征参数,我们使用RNAfold软件 [5] 来预测mRNA的二级结构,二级结构的预测结果是以点或括号“ . 或 ( ) ”表示的。“( )”表示配对的碱基,形成茎结构;“.”表示不配对的碱基,形成单链或环结构。在本文中,为了计算方便对“(”和“)”不加以区分 [4] 。给出任一条mRNA序列,结构–序列模式信息可由二级结构预测图和相邻的三个碱基中中间的碱基表示。64种三联体可约化为32种(4 × 23)组合,即:A(((、A((.、A(..、A...、A.((、A.(.、A..(、A.((、U(((、U((.、U(..、U...、U.((、U.(.、U..(、U.((、G(((、G((.、G(..、G...、G.((、G.(.、G..(、G.((、C(((、C((.、C(..、C...、C.((、C.(.、C..(、C.((。我们将这种三联体结构片段的频数作为结构–序列模式的特征参数。
3.3. 物理化学性质
AAIndex database [8] 由AAindex1、AAindex2两部分组成,其中的AAindex1是氨基酸指数(amino acid indexes)表,这些指数是将氨基酸不同物理化学和生物学性质量化后的数据,目前共搜集了544种氨基酸指数。本文采用了其中九种物理化学性质 [6] [7] ,分别是Hydrophilicity value (亲水性值)、Mean polarity (平均极性)、Isoelectric point (氨基酸等电点)、Refractivity (折射率)、Average flexibility indices (平均灵活性指标)、Average volume of buried residue (埋藏残基的平均体积)、Electron-ion interaction potential values (电子离子相互作用势值)、Transfer free energy to surface (转换到表面的自由能)、Consensus normalized hydrophobicity (标准化后的疏水性),利用同一蛋白质序列中不同距离的氨基酸残基之间存在的相互作用,获得更多的预测信息。九种物理化学性质见表1,这些信息均来自AAIndex database [8] 。首先,用标准化后的氨基酸指数(amino acid indexes)表示序列中的每一个氨基酸残基,氨基酸指数同样提取于AAIndex database,利用公式(3)对第i种氨基酸指数进行标准化。这种方式已被Chou等人用于物理化学性质的标准化 [9] - [11] 。
(3)
(4)
(5)
标准化后,每一种特性变成了一组新的20个数字。对于第i种特性,任一条蛋白质序列可以表示为
,其中L为序列长度,
(
)是序列中第k个氨基酸残基的第i种特性的标准化值,然后利用等式(6)计算自相关函数的值。
(6)
(7)
其中,T是一个常数。
通过计算得到的每一种特性的特征向量,都包含该条序列中不同距离的氨基酸残基间的相互作用关系。物理化学特性参数向量可表示为公式(8):
(8)
3.4. 氨基酸黏性信息Stickiness of AAs
在生物体中存在着大量的蛋白质,其中功能性蛋白质的两两相互作用 [12] [13] 是很少的,而非功能性的、随机的蛋白质相互作用有很多。某些细胞特性有助于减少非功能性蛋白质的相互作用。基于蛋白质相互作用面和蛋白质表面溶剂可及性的氨基酸残基频数,氨基酸黏性(stickiness)可以被定义为 [14] :
(9)
其中fAA(interface)代表蛋白质相互作用界面(interface)的氨基酸频数,fAA(surface)代表蛋白质表面(surface)的氨基酸的频数。Levy通过大量的分析发现蛋白质表面黏性的变化可能与它的亚细胞位置有关 [14] 。通过公式统计氨基酸黏性值,结果见表2 [14] 。
Petersen [15] 等人开发了网站Net Surf P (http:// www.cbs.dtu.dk/ ser vic es/NetSurfP-1.1/),通过网站提交任务可预测蛋白质的表面位置信息,相对表面溶剂可及性(RSA),绝对表面溶剂可及性,RSA的z-fit值,α螺旋概率,β折叠概率和无规卷曲概率。利用这些预测结果,每条蛋白质序列可以构建成一个向量(10):
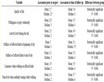
Table 1. The 9 physicochemical properties
表1. 9种物理化学性质
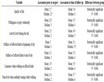
Table 2. The stickiness index of amino acids
表2. 氨基酸黏性
(10)
L为序列长度,A为蛋白质序列中的氨基酸残基,Sr为RSA,Sa为绝对溶剂可及性,Z为RSA的z-fit值。根据表2,用氨基酸黏性值S代替公式(10)中的Aj,得到向量(11)。
(11)
然后利用自相关方程(12)计算各个预测信息,特征参数向量表示为公式(13):
(12)
(13)
3.5. 进化信息(PSSM)
本文通过本地运行PSI-BLAST [16] 程序,用细胞凋亡蛋白质数据集中的每条序列与nr数据库(released on 04 2016)中的序列进行比对和评价。设置E-value值为0.001,经过三次迭代搜索,获得数据集中每条蛋白质序列的同源序列,构建位置特异性得分矩阵(position-specific scoring matrix, PSSM),提取蛋白质进化的保守信息 [17] 。首先将矩阵以行为单位利用公式(14)进行标准化,
(14)
其中
是由PSI-BLAST直接得到的得分,
是二十种氨基酸的平均值,
是标准差,L为序列长度。
对于一条序列长度为L的蛋白质P,标准化后的PSSM矩阵(15)可以表示为:
(15)
为了利用该序列顺序的信息,采用自相关函数(16)来得到特征参数向量(17)
(16)
(17)
4. SVM算法
4.1. 支持向量机(Support Vector Machine, SVM)
支持向量机(Support Vector Machine, SVM)是一种用于解决分类和回归问题的机器分类算法。基本原理是将低维空间向量集映射到高维空间,通过选用适当的核函数和寻找最优分类面,使得不同类别样本之间的间隔最大化,从而有效地解决非线性分类问题。
本文使用支持向量机的C-SVC (C-Support Vector Classifier,C-支持向量分类器)类型,径向基核心函数,采用台湾大学林智仁(Lin Chih-Jen)教授开发的libsvm 3.21软件包 [18] ,通过搜寻最优C和γ值来训练细胞凋亡蛋白质中按照亚细胞位置类别作为标记的特征参数数据集,进行Jackknife检验 [19] [20] 和独立检验的预测。
4.2. 预测性能评估
本文采用了Jackknife检验和独立检验,Jackknife检验被认为是较严格和客观的统计检验方法;而独立检验则反映了算法对新序列的预测能力。在Jackknife检验中,假设细胞凋亡蛋白质数据集共有N条蛋白质序列,把其中的每条蛋白质依次作为待测样本,剩下的N-1条蛋白质作为测试集测试,并给出这条细胞凋亡蛋白质的分类。在独立检验中,本文随机选取80%的数据作为训练集,用Jackknife检验训练出模型,将其余20%的数据作为测试集来检验模型的预测能力。本文采用的评价算法性能的指标为:敏感性 (Sensitivity, Sn)、特异性(Specificity, Sp)、预测成功率(Accuracy, Acc)、总体成功率(Overall accuracy, OA) 和评价综合预测结果的相关性系数(Mathew’s Correlation Coefficient, MCC),定义如下:
(18)
(19)
(20)
(21)
(22)
其中,TPi表示第i类亚细胞位置中预测正确的细胞凋亡蛋白质条数;TNi表示非第i类亚细胞位置中的细胞凋亡蛋白质被正确的识别为非i类的蛋白质条数;FPi表示非第i类亚细胞位置中的细胞凋亡蛋白质被错误的识别为第i类的蛋白质条数;FNi表示第i类亚细胞位置中细胞凋亡蛋白质被错误的识别为非i类的蛋白质条数。
5. 结果与讨论
5.1. 预测结果比较
本文分别从mRNA和氨基酸角度提取了不同的信息对细胞凋亡蛋白质的亚细胞位置进行预测。mRNA方面分别利用了三阅读框下3-mer mRNA序列信息和mRNA二级结构–序列信息。根据三阅读框下3-mer mRNA序列信息可以构建一个192维的特征向量,mRNA二级结构–序列信息可以构造成32维的特征向量,去掉部分特异性不强的信息,得到一个23维特征向量,将这两个特征融合后进行预测。基于Jackknife检验预测结果见表3。由表3可以看出,采用mRNA单一信息时总体预测成功率分别达到63.44%和58.61%,而将mRNA序列与结构信息融合后总体预测成功率达到65.56%,比三阅读框下3-mer mRNA序列信息提高了2.12%,比mRNA二级结构–序列信息提高了6.95%。结果表明,融合序列与结构信息后预测成功率有了提高,融合序列与结构信息能够更充分的反映出mRNA的特性。
氨基酸方面选用了物理化学性质,氨基酸黏性信息,进化信息三种特性。比较发现在物理化学性质中当T = 50时预测效果最佳,在氨基酸黏性特性中同样是当κ = 50时预测效果最佳,进化信息中当λ = 1时预测效果最佳。之后,将氨基酸的三种特性选取最优变量融合称为AA hybrid,并对AA hybrid进行预测,预测结果见表4。从表4可以看出,采用物理化学性质总体预测成功率达到68.88%,氨基酸黏性信息总体预测成功率达到70.69%,进化信息比前两者更高,达到71%。将三种特性混合后总体预测成功率提高到77.34%,比单一氨基酸特性最高提高了8.46%。
由表3,表4可以观察出,多特征融合后的总体预测成功率都要高于单特征的总体预测成功率,说明多特征融合可以更加全面的刻画细胞凋亡蛋白质。
将mRNA的特性与氨基酸的特性全部融合(hybrid),利用融合后的特性进行预测。预测结果见表5所示。观察发现,同样是基于Jackknife检验,融合mRNA信息后预测效果更佳,比单独采用mRNA特性总体预测成功率提高了16.62%,比只用氨基酸特性的总体预测成功率提高了4.84%,这就表明mRNA对于细胞凋亡蛋白亚细胞定位预测具有一定作用。
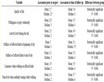
Table 3. Prediction performance of mRNA feature parameter
表3. mRNA特性参数预测结果
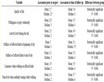
Table 4. Prediction performance of AAs feature parameter
表4. 氨基酸特性参数预测结果
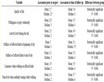
Table 5. Hybrid feature under Jackknife test
表5. 融合特征Jackknife检验
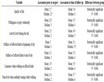
Table 6. The predictive accuracies for the 262 dataset and the dent dataset
表6. 262数据集和独立测试集预测结果
为了进一步评估算法对新序列的预测能力,本文进行了独立检验,分别从来自四个亚细胞位置的331条序列中随机挑选20%,共69条序列构成独立测试集,命名为Ident数据集。剩余的262条序列构成262数据集。采用融合特征(hybrid)作为输入参数输入SVM,262数据集的jackknife检验和Ident数据集的独立检验预测结果列于表6。观察表6发现,混合特征对262数据集具有较好的预测能力,总体预测成功率达到80.15%。对于独立测试集预测成功率达78.26%,反映出融合特征对未知细胞凋亡蛋白质也具有良好的预测能力。
5.2. 讨论
研究发现不同亚细胞位置细胞凋亡蛋白质的mRNA序列和结构具有一定的特异性。只采用氨基酸信息进行蛋白质亚细胞定位预测特征略显单一,将mRNA序列信息与二级结构信息融合后取得了更优的预测成功率。mRNA局部二级结构与其功能密切相关,结构-序列模式中三联体参数从短片段的结构和碱基种类出发考虑mRNA执行功能时的局域性,揭示出mRNA与细胞凋亡蛋白质亚细胞位置的关系。采用支持向量机的方法在Jackknife检验下取得了良好的预测结果,进行独立测试后发现算法对新序列也有较好的预测能力,这也说明所选择的特征参数能够比较有效的区分不同亚细胞位置中的细胞凋亡蛋白质。本文综合考虑了氨基酸的物理化学特性,氨基酸的黏性,进化信息,mRNA的序列信息和mRNA的二级结构信息,如果将更多的细胞凋亡蛋白质亚细胞位置的特征信息融合,更有效的提取序列中的蕴含的结构与功能信息,将对进一步提高细胞凋亡蛋白质的亚细胞定位预测有所帮助,也可能对进一步研究细胞凋亡蛋白质的功能提供一些理论依据。
致谢
感谢国家自然科学基金(61361015)和教育部第46批留学回国人员科研启动基金的支持。
*通讯作者。