1. 引言
球磨机负荷可靠检测是选矿过程节能降耗和高效稳定运行的必要条件。磨机负荷的检测通常采用磨机运行时外部响应信号振动及振声间接获得。目前磨机负荷参数检测系统实现方式包括嵌入式仪表或者软件工具包 2 [1] 。目前这些开发方式在矿石来料不稳定、多变情况下由于模式固定、适应性较差。为了有效解决球磨机负荷监测问题,目前国际研究热点是通过具有较高灵敏度和较强抗干扰性筒壁振动信号来监测球磨机运行。充分提取和利用这种非线性非平稳信号蕴含的大量丰富有价值信息,通过特征选择和优化技术提取振动和振声信号集合经验模态分解(EEMD) [2] 本征模态函数IMFs特征,构建基于IMFs特征空间的集成模型,并在过程漂移时自适应更新集成模型结构和参数,适应时变非线性磨矿过程复杂工况变化,是保证球磨机负荷集成模型泛化性、可信度和适应性的有效手段。因此,需要开发具有模块化结构和实验功能的球磨机信号分析和磨机负荷监测软件系统。
由于球磨机外部响应信号振动、振声变量时域特征难以提取,频域信息具有较大的冗余成分,国内外提出了基于频谱特征选择的磨机负荷检测方法,并对此进行了大量的研究。目前针对振动、振声信号的处理效果比较好的是多传感器信息融合的磨机负荷集成建模 [3] 。近年来,混合编程技术运用越来越成熟,可以集合两个或者多个软件的优点进行混合编程,大大提高软件的开发效率。MATLAB作为一种高级语言,具有极高的编程效率,但它也存在界面开发能力弱 [3] 、执行速度慢、算法和数据不利于保密以及依赖MATLAB编译环境不能独立运行等一些局限性。C#.NET开发的系统界面友好、执行速度快、易维护、可升级等特点,能有脱离编译环境而运行,而且算法和数据保密性强,但是在工程计算方面与MATLAB相比就相形见绌了 [4] 。因此实现MATLAB与C#的混合编程,结合两者的优点,必将提高软件的开发效率以及软件的性能,也可以工作人员带来很大的方便。
针对球磨机负荷难以直接测量问题,利用MATLAB与C#混合编程开发了一套球磨机信号分析和关键参数预报系统。利用Matlab的Deploytool工具将信号EEMD分解、IMF频谱变换、iPLS特征选择、关键参数模型训练和预测一系列m函数编译为C#.NET dll程序集,供C#.net调用,并由C#.NET完成前端界面开发和端口操作任务。该系统充分利用混合编程优势互补,提高了编程效率。
2. 球磨机信号分析和关键参数预报建模策略
针对磨机筒体振动和振声信号组成复杂、信息存在冗余性和互补性、现有球磨机负荷测量模型大多假设振动信号具有线性平稳性,同时传统单模型结构的稳定性和泛化性有限,本文提出基于集合经验模态分解(Ensemble Empirical Mode Decomposition, EEMD)和区间偏最小二乘(Interval Partial Least Squares, iPLS)本征模态频谱特征提取的球磨机负荷参数集成建模方法。筒体振动和振声信号采用非线性、非平稳EEMD自适应分解技术获取反映球磨机负荷参数变化的本征模态函数(IMFs),利用区间偏最小二乘iPLS 方法去掉本征模态函数频谱中不相关和冗余成分,提取本征模态函数频谱特征,融合时域电流信号,建立磨机负荷参数随机向量泛函连接网络RVFL(Random Vector Functional Link)集成模型,通过对多个RVFL成员模型输出加权平均获得磨矿浓度、填充率和料球比三个球磨机负荷参数估计值。
2.1. 信号分解模块
集合经验模态分解是在经验模态分解(EMD)的基础上发展起来的一种信号处理方式,它是EMD方法的改进。它能够自适应的将信号分解为不同时间尺度的IMF集合,每个IMF中只包含一种振荡形式,代表了信号的本征波动模式,这些波动模式的产生与球磨机的负荷密切相关。
基于EEMD的球磨机振动信号分解步骤如下:
首先在振动信号
中加入振幅为
的白噪声得到:
(1)
寻找筒体振动信号
的极大值点和极小值点;
采用样条插值法拟合
的上下包络线,计算上下包络线的均值曲线
,得到振动信号
与均值曲线的差值
(2)
以
为原始信号,重复步骤(2)和(3)得到
(3)
反复进行k次,直到得到第一个IMF分量
,定义第一个振动信号IMF分量为
。
(4)
原始振动信号
减去第一阶IMF分量得到剩余部分
,令
,重复步骤(2),(3)和(4),得到第二阶IMF分量
。
重复步骤(2)~(4)n次可得到多个IMF分量,当
小于给定的某个值或成为一个单调函数的时候则整个过程停止。得到第一次筛分结果
(5)
随机产生新的白噪声
,返回步骤(1);
将上述步骤(1)~(7)重复N次,得各阶IMF集合
(6)
相应阶的IMF平均值作为振动信号该阶的IMF结果:
(7)
则原振动信号
可表示为IMF与振动信号分解残差的形式:
(8)
按照上述分解步骤,最终球磨机的振动信号
会被分解为一组时间尺度不同的本征模态函数
。
振声信号
的EEMD分解方法与振动信号基本相同,最终可以得到振声信号的本征模态函数
,振声信号可以表示为:
(9)
其中
为振声信号分解的残差。
2.2. 特征选择模块
振动和振声信号EEMD分解得到的本征模态函数时域波形特征并不明显,本文通过Welch法变换为频域功率谱后能够清晰的显示出IMF频域特征,但是一系列IMF频谱空间高维特性,导致后续的磨机负荷模型复杂度增加,泛化性降低。为此,本文采用偏最小二乘法(iPLS) [5] 对信号的频谱进行特征选择,以降低输入空间维数。基于iPLS的振动信号IMF频谱特征选的步骤如下:
1) 将全频谱
分为
个等宽的子区间,
;
2) 采用非线性迭代偏最小二乘算法(NIPALS)在每个子区间
分别建立磨机负荷参数
局部偏最小二乘回归模型
。PLS算法表示为
(10)
其中矩阵
,
分别表示IMF频谱区间和输出磨机负荷参数的负荷向量。
是权值矩阵,
为内部模型对角系数矩阵,
。第i个磨机负荷参数,在IMF的第
个特征频段iPLS模型输出
为
(11)
3) 分析各IMF频谱子区间模型,采用交叉校验均方根误差(RMSECV),比较各子区间局部模型精度。均方根误差(RMSE)和相关系数(r)作为模型性能的测度。RMSE定义如下:
(12)
其中
为磨机负荷参数实验室测量值,N为样本个数。RMSECV为交叉校验的均方根误差。取精度最高的局部模型所在区间为入选频段。相关系数r定义如下:
(13)
其中N为样品数目,
为交互验证预测值的均值;
为实际测量值的均值。通过上述方法对振声IMF频谱进行特征选择,找到各IMF中与磨机负荷密切相关且富含信息量最多的特征子频段。
2.3 基于IMF频谱特征的集成建模方法
鉴于模型简单、训练速度快、泛化性能好特点,采用随机向量功能连接网络 [6] 建立振动、振声IMF特征频谱和磨机负荷参数之间的非线性模型。设
为振动和振声IMF特征选择结果,作为非线性模型的输入变量
,以磨机负荷参数作为模型的输出变量
,隐含层节点为Hn,激励函数为g(x)的单隐层前馈神经网络(Single-hidden Layer Feed-forword Neural Network,SLFN)统一模型为
(14)
其中
是连接第
个隐含层节点的输入权值,
是第
个隐含层节点的偏差,
是隐含层节点的输出权值,
表示
和
的内积。激励函数
可以为Sine,Sigmoid等。式(14)可以简化为
(15)
其中
(16)
,
17)
如果隐含层节点足够多,SLFN就能在输入权值随机产生时逼近任何连续的函数。设输入为
,激励函数为
,隐含层节点数为Hn,则RVFL模型建模步骤如下:
1) 随机产生子模型输入权值
和阀值
;
2) 计算子模型隐含层输出矩阵
;
3) 计算子模型的输出权值
,
,其中
为输出矩阵
的Moore-Penrose的广义逆矩阵。
集成模型的预报值为各子模型的加权和,表示为
(18)
其中,
表示集成模型对第
个磨机负荷参数的预报值,
为成员模型个数,
表示第
个子模型的权值,
表示第
个子模型对第
个负荷参数的预报结果。权值
的大小表示子模型在整个集成模型的贡献多少,本文中取
即所有成员模型的贡献率全部相同。
3. 系统的总体设计
系统整体根据需求以功能模块的形式进行开发,模块之间功能相对独立,数据和信息以模块间通信的交换的方式实现,便于管理和开发。根据球磨机信号分析和关键参数预报系统的功能设计,系统可以划分为数据导入模块、信号处理模块、特征选择模块、负荷建模与参数预报模块等。球磨机信号分析和关键参数预报系统功能树如图1所示。
4. 系统功能的实现
系统功能的实现如下:
1) 通过数据导入模块采集到的离线数据导入到系统,然后转换成MATLAB和C#.NET的中间数据类型(MWArray),以供下一步的调用;
2) 信号分解模块将振动、振声时域数据采用集合经验模态分解方法分解为一系列本征模态函数,并将每个IMF时域分量转化为相应的IMF频谱;
3) 特征选择模块利用区间偏最小二乘方法选择与磨矿浓度、料球比和填充率密切相关的IMF及其频谱特征,组成磨机负荷模型输入特征变量;
4) 负荷建模与参数预报模块包括负荷建模和参数预报,负荷建模建立基于特征选择的输入和磨机负荷参数之间的数学模型,参数预报根据前面建立的磨机负荷模型预报磨机负荷参数的变化。
5. 仿真实验和系统测试
5.1. 系统主界面
运行球磨机信号分析和关键参数预报系统,系统主界面如图2所示。系统分为四部分:数据导入模块、信号分解模块、特征选择模块以及参数预报模块。
5.2. 数据导入功能
数据导入模块是人机交互的接口,通过数据导入模块用户可以将采集到的离线数据导入到系统中,实现对数据一系列处理的开端。如图3所示,用户通过右侧的浏览按钮可以自由的选择要处理的振动数据和振声数据原始数据文件,原始数据文件可以是txt文本文件、mat文件格式。如果是TXT文件格式,
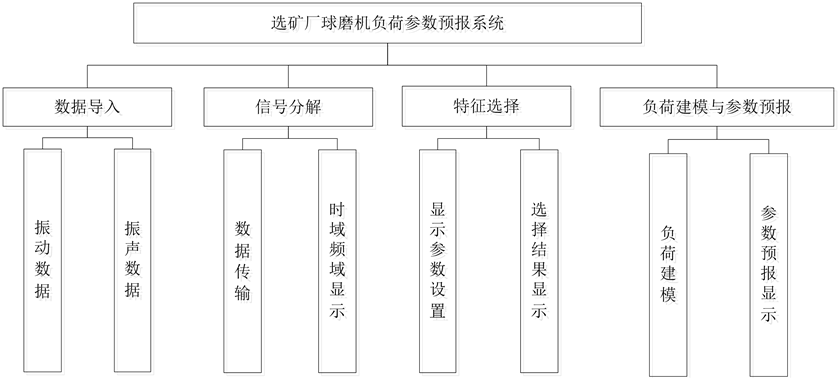
Figure 1. Mill load parameter forecast system function tree
图1. 磨机负荷参数预报系统功能树
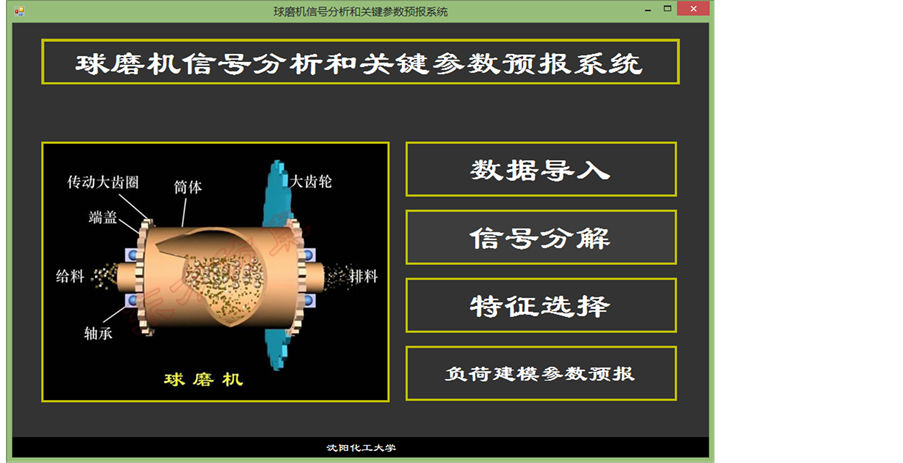
Figure 2. Main interface of signal analysis and key parameter forecast system of ball mill
图2. 球磨机信号分析和关键参数预报系统主界面
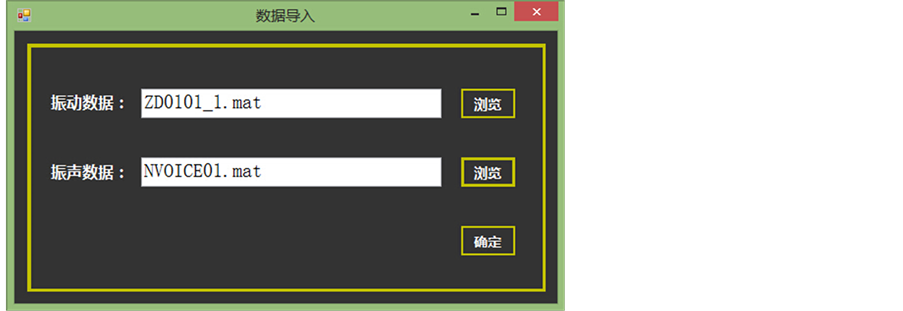
Figure 3. Data import module interface
图3. 数据导入模块功能界面
在后台将其转化为mat数据文件格式。如果是mat文件格式,则直接调用。
5.3. 信号分解和频谱变换功能
信号分解和频谱变换模块如图4所示,用户可以设置信号分解的次数以及要加入的噪声比。设置完成后点击“执行信号分解”按钮进行信号EEMD分解,分解完成后以mat文件格式保存振动和振声时域IMF数据。同时完成频谱变换,各IMF函数的频谱也以mat文件格式保存。对于振动、振声时频域数据也可以图形显示。选择EEMD分解的IMF系列函数,设置显示的时频域范围,完成振动、振声信号的时域和频域波形显示。波形显示功能,用户还可以通过鼠标对曲线进行放大、缩小、左右移动等操作。本实验选择球磨机信号采集系统在不同球、料、水配比下采集26组振动、振声信号中的第一组进行测试,设置噪声比Nstd = 0.2,分解次数NE = 1,选择振动时域频域显示范围缩减到10,000,振声时域显示范围0~10000,振声频域显示范围0~4001,EEMD分解的振动、振声时频结果显示如图4、图5、图6和图7所示。
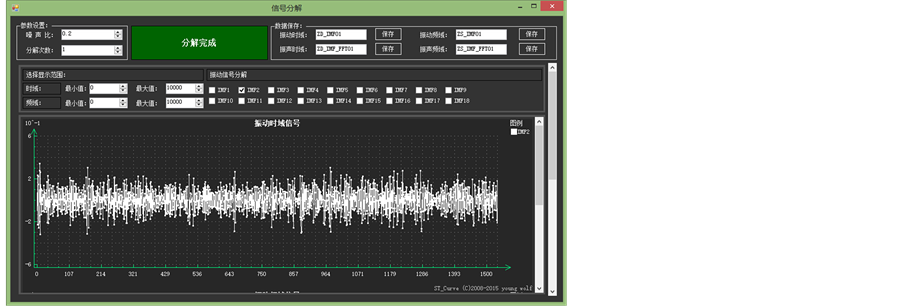
Figure 4. Vibration time domain decomposition results
图4. 振动时域分解结果显示
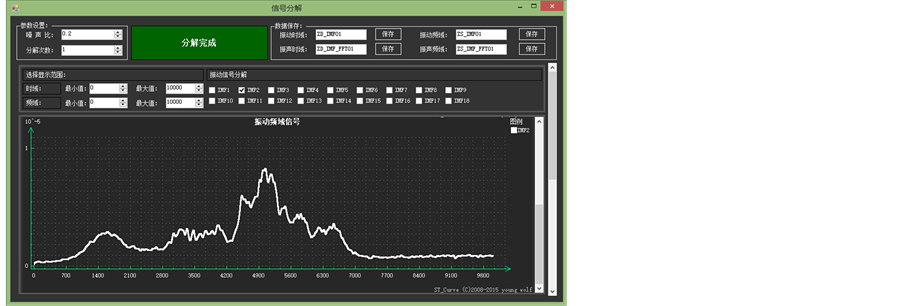
Figure 5. Vibration time frequency conversion result
图5. 振动时频转换结果显示
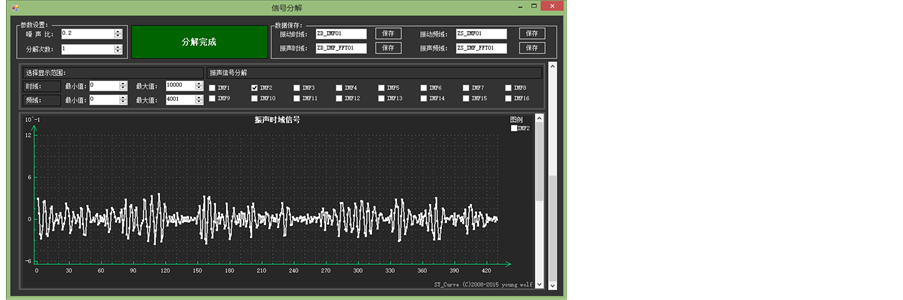
Figure 6. Acoustic time domain decomposition results
图6. 振声信号时域分解结果显示
5.4. 特征选择模块
特征选择模块如图8和图9所示,功能界面分为数据组合、参数设置以及图形显示三个部分。数据组合部分,用户可以根据信号分解的结果剔除误差太大的数据组,然后重新组合,组合完成后按钮变成绿色;参数设置部分,用户可以选取自变量(振声或者振动)和因变量(磨矿浓度、料球比和填充率),设置区间个数、区间组合个数(2,3,4)以及交叉校验样本个数,设置完成后点击“进行特征选择”按钮进行特征
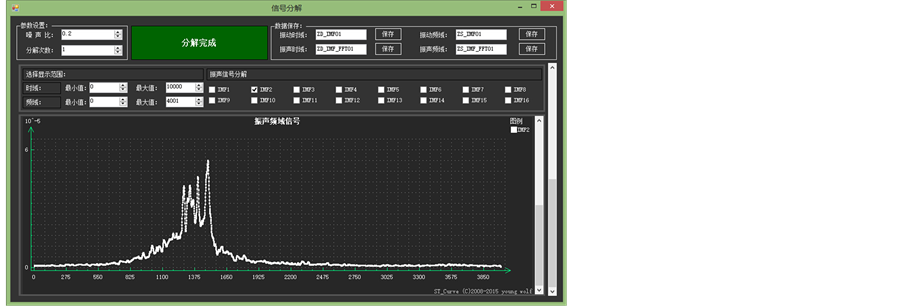
Figure 7. Acoustic frequency transform results
图7. 振声信号时频转换结果显示
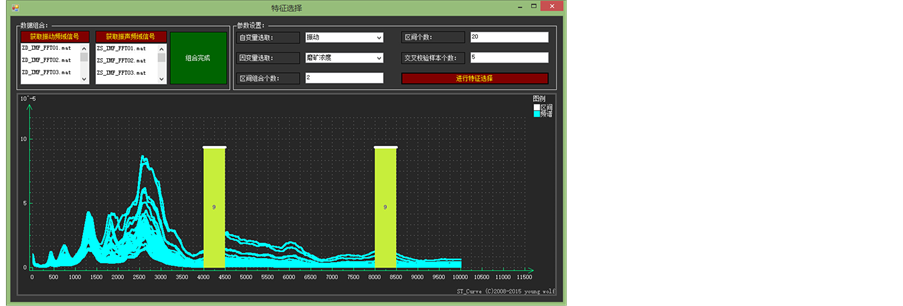
Figure 8. Vibration-grinding concentration characteristic selection
图8. 振动–磨矿浓度特征选择结果显示
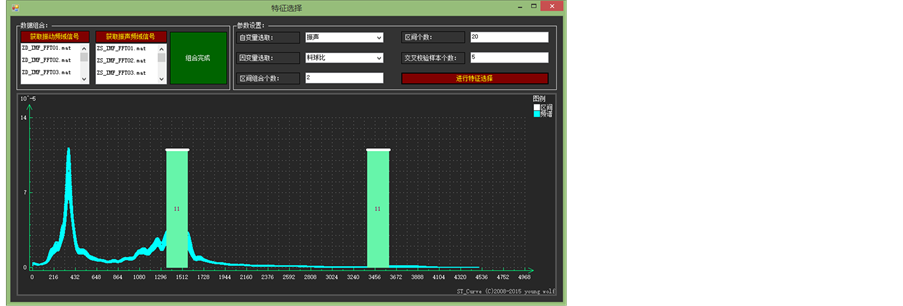
Figure 9. Acoustic-material ball ratio feature selection
图9. 振声–料球比特征选择结果显示
提取;界面下方的图形显示模块则是将特征选择的结果显示在这个模块。本实验针对磨机负荷参数磨矿浓度进行振动信号第2个IMF以及料球比进行振声第二个IMF进行iPLS频谱特征提取,设置交叉校验样本个数为5,将IMF平均划分为20个子区间,在每个子区间上建立磨机负荷参数PLS模型 [7] ,计算子区间PLS模型的预报值与真值之间的RMSECV,设置区间组合个数为2,以RMSECV最小的两个子区间作为特征频段。
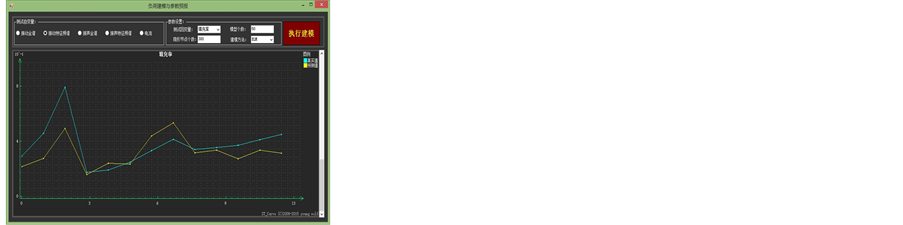
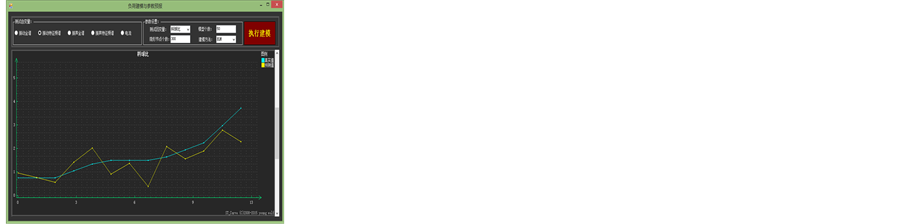
(a) 磨矿浓度 (b) 料球比 (c) 填充率
Figure 10. Forecast Results of ball mill load parameters
图10. 磨机负荷参数预测结果
5.5. 参数预报模块
球磨机负荷参数预报模块如图10所示。左上方的测试自变量选择模块,用户可以根据需要选择要建模的自变量(振动全谱、振声全谱、振动特征频谱和振声特征频谱);界面右上方的参数设置模块,用户可以设置建模的模型个数,设置后点击“执行负荷建模”按钮,对所选的自变量进行建模分析;界面最下方是图形显示模块,建模后的磨机负荷参数真实值与预测值的对比曲线在此模块上显示。本实验设RVFL隐含层节点数为300,建立50个磨机负荷多传感器信息的预报子模型进行集成,即为多传感器信息磨机负荷集成模型。图10(a)、图10(b)和图10(c)分别为磨机负荷参数磨矿浓度、填充率和料球比的预测结果,预测值接近实测值,满足工程需求。
6. 结束语
针对球磨机负荷难以直接测量问题,采用MATLAB和C#混合编程方式,开发了一套球磨机信号分析和负荷参数预报系统。该系统利用MATLAB丰富的函数资源和C#强大的可视化编程优势,实现振动振声信号的集合经验模态分解的多传感器信息融合的磨机负荷集成建模,以及以振动振声信号和电流信号相融合为子模型输入,以磨矿浓度、填充率和料球比作为输出建立的多传感器信息的RVFL集成模型,搭建快捷、方便、人机界面友好的磨机负荷预报软件。
系统测试结果表明该系统具有信号分解、特征选择、模型训练和参数预报功能,能够有效选择料球比、磨矿浓度和填充率密切相关的筒体振动和振声信号IMF频谱特征,基于谱特征的磨机负荷参数预报系统泛化性能良好。该软件可给研究人员提供更强的实用性,亦可用于磨矿过程的监测,保证磨矿过程的安全平稳生产,提高磨矿效率和质量。
基金项目
国家自然科学基金项目(61203102, 61573364),辽宁省教育厅科学研究项目(L2013158, L2013272)。