1. 引言
工件识别是工业流水线上必不可少的步骤,其目的在于获得工件的分类、定位信息 [1] ,以便用机器人进行分拣作业。近年来,为了提高生产效率,采用机器人替代人工劳动力越发受到重视,工件识别是分拣作业的重要步骤之一,如何提高工件识别的准确性显得尤为重要。
目前主流的工件识别方法主要有两类:一类是基于模板的匹配算法;另一类是基于学习的分类器设计的算法,以神经网络和支持向量机为代表 [2] - [5] 。前者实现简单,适用于分类对象已知的情况;后者原理复杂,训练成本高,分类结果可控度不高,适用于分类规则不明显的情况。
本文的分拣系统具有工件种类少,但是识别准确率要求高的特点。假设实验流水线仅包含图1所示的两种工件,为了降低实现的复杂度并且提高结果的准确度,本文采用模板匹配算法 [6] [7] 。模板匹配算法的重点在于需要预先建立模板库,再将待识别的工件图像与模板进行匹配即可得出结果,其整体流程如图2所示,将图像进行预处理之后根据匹配策略得出匹配结果。

Figure 2. The flow chart of template matching
图2. 模板匹配流程图
目前的模板匹配策略主要有两类:基于像素点灰度相关的匹配和基于特征的匹配。其中前者计算精度高、抗干扰能力强,但是对于相似的两个工件,识别结果会出现偏差,难以取舍;后者基于工件的个性,能够区分出相似的工件,且计算速度比较高,但是由于使用的是由数据集映射得到的特征集数据,存在特征集的优选问题。本文提出了一种结合两者的二次识别算法,在保证匹配准确性的基础上,又尽可能地提高了识别的速度。
2. 模板库的建立
工件的摆放姿态会影响机器臂的抓取动作,因此每种姿态需要单独建立模板,虽然本文的实验生产线只有两类工件,但是每类工件在生产线传送带上分别含有图3和图4所示的5种和3种稳定摆放姿态,最终本文建立的模板库如图12所示包含八种模板,依次按1~8编号。
3. 一次识别
一次识别流程如图5所示,主要步骤包括了高斯滤波、二值化、保留最大连通域、归一化和一次识别。
3.1. 高斯滤波
高斯滤波器是一种线性平滑滤波器,能够有效地去除服从正态分布的高斯噪声,其原理是根据高斯函数的形状选择权值作为该像素点对目标像素的贡献权值。本文涉及的系统主要是高斯噪声,所以采用高斯滤波对图像进行去噪处理。
3.2. 二值化
图像二值化的目的在于将目标和背景区分开来,有定阈值分割、局部阈值分割方法等。由于本文的系统保持采集图像的光照条件基本相同,可以采用定阈值分割方法。采用OpenCV库的定阈值函数cvThreshold()即可实现。图6所示为原始工件图像。图7所示结果为经过高斯滤波和二值化的图像。

Figure 3. Five stances of the first work piece
图3. 工件1五种姿态

Figure 4. Three stances of the second work piece
图4. 工件2三种姿态

Figure 5. The flow chart of one-time recognition
图5. 一次识别流程图

Figure 7. The image after Gaussian filter and binarization
图7. 高斯滤波、二值化后的图像
3.3. 保留最大连通域 [8]
经过去噪以及二值化的图像,仍然会存在一些较小的杂质,会影响后期识别,需要去除。本文采用了OpenCV库提供的漫水填充算法cvFloodFill()标记出各连通区域,采用排序算法找出最大的连通域并保留,结果见图8。
3.4. 角度归一化和大小归一化
二值化后的工件图像的大小和角度不尽相同,会给后期识别匹配工作造成不必要的困扰,因此必须进行图像归一化的预处理。
本文截取图像的最小外接矩形,将该矩形的中心与质心相对比,并结合水平轴与其逆时针旋转碰到的该矩形的第一条边的夹角计算得出旋转角度,之后利用空间变换的方法将图像进行旋转实现和模板图像一样水平且质心靠左。
角度归一化后,删除全白行和全白列实现目标区域的分割,采用双线性插值算法将图像大小归一化为110*60,结果见图9。
3.5. 一次识别
一次识别采用基于像素点的匹配算法,计算匹配系数进行初步筛选。其基本思路为将待匹配图像按模板顺序与每个模板进行像素点灰度值的比较,计算相似率 [9] ,即为一次识别的匹配系数R1:
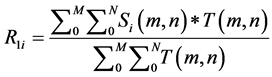
其中,i表示模板的编号,S表示模板图像,T表示待匹配图像,M、N为图像的宽和高。
本文随机采集了1000幅图像,对其进行一次识别,结果如表1所示。一次识别的准确率已经高达97.5%,但是仍然存在误判的情况。
分析一次识别匹配系数可以发现,部分相似的工件容易混淆。以本该识别为2号模板的某工件图像为例,该一次识别结果误判为模板3,仔细分析表2所示的一次识别匹配系数可以发现,该工件图像与模板2、3的匹配系数分别为0.88、0.89,非常靠近,也就是说该图像匹配结果为模板2的可能性也非常高,并且还有可能为模板4。针对这种匹配系数相近的情况,本文引入二次识别加以区分。

Figure 8. The image after maximum connected domain retention
图8. 保留最大连通域后的图像

Figure 9. The image after normalization
图9. 归一化后的图像
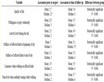
Table 1. The results of one-time recognition random test
表1. 一次识别随机测试结果
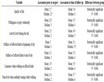
Table 2. The first coefficient of one-time recognition error
表2. 错误范例一次识别匹配系数
4. 二次识别
4.1. 基本原理
二次识别采用基于特征的匹配算法,重点区分一次识别匹配系数相近的工件图像。其主要原理是对模板库中的每个模板提取一定数量的特征,构成特征组合 [10] ,将待匹配模板的特征组合区域与模板进行比较计算相似率,即为二次识别的匹配系数R2。
二次识别的主要流程包含模板特征的提取和工件图像的识别两部分,其中模板特征的提取主要流程如图10所示,先提取特征组合,然后给每个特征分配匹配系数xj,最后将特征信息存储入库。
4.2. 特征提取
由于本文采集的是工件的静态图像,不存在空间上的转换关系,并且经过图像预处理之后,图像只有黑白两种灰度,因此采用特征区域坐标、面积、特征灰度值作为特征信息。
提取的特征数量必须保证足够与其他模板相区分,针对本系统的模板库,选定了三个特征,平均分配每个特征的匹配系数为0.33。若是增加模板,可适当增加特征数量以保证各模板的区分度。
选取模板特征时,需根据一次识别的结果对相似模板进行分析,突出相似模板之间的差异性,以表2显示的一次识别误判为例,该工件原图如图11所示,由于拍摄角度的问题,该工件在1区呈现为黑色的情况,导致跟模板3混淆。但是通过观察可以发现2区与3区依然保持着模板2的灰度特征,因此确定了图12所示的模板2、模板3的2、3区的特征用以区分彼此。

Figure 11. The binary image of one-time recognition error
图11. 一次识别错误范例工件二值化图像
模板1 模板2 模板3 模板4
模板5 模板6 模板7 模板8
Figure 12. The template library
图12. 模板库示意图
其余模板特征选取过程以此类推,最终本文八个模板的特征组合提取如图12中的红色矩形框标出区域所示。
4.3. 二次识别
工件图像的识别如图13所示,提取待匹配图像的特征区域信息,与模板进行比较,计算匹配率,其基本步骤包括:
1) 将一次识别匹配系数进行排序,若第一位的匹配系数大于0.8,且与第二位相差0.1以上则直接输出一次识别结果,否则提取与第一位匹配系数之差小于0.1的所有模板编号,转至步骤2。
2) 依次将待匹配图像与步骤1中提取的编号对应的模板图像的特征组合区域进行特征信息对比。
3) 计算二次识别匹配系数:
(i表示模板编号;ni,j表示待匹配图像与i模板的j特征是否匹配,匹配为1,否则为0;
)。
4) 综合考虑一次识别和二次识别,得出最终匹配结果:
。
4.4. 实验结果
为了验证二次识别的有效性,本文对上文提到的一次识别错误范例进行了二次识别,最终得到了如表3所示的结果。
由表3可知,经过二次识别之后,匹配结果正确,并且与其他模板的匹配率很低,不易混淆。待匹配图像与模板3和4的匹配系数分别为0.89和1.13,与模板2的匹配系数分别相差了0.99和0.75,有明

Figure 13. The flow chart of two-time recognition
图13. 二次识别流程图
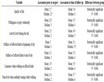
Table 3. The second coefficient of one-time recognition error
表3. 错误范例二次识别匹配系数
显的区分度。本文随机采集了1000幅工件图像进行测试,最终识别率高达100%,由此可见二次识别弥补了一次识别不易区分相似工件的缺点,达到了本系统的既定目标。
5. 结论
本文针对工件识别问题提出了基于模板匹配的二次识别的方法,经实验证明取得了非常好的效果。一次识别根据有效区域像素点灰度值计算匹配率,体现了待匹配图像与模板的整体匹配度,然而由于拍摄角度的影响,存在待匹配图像与多个模板整体相似的情况,会出现误判。二次识别则根据特征区域的有效信息计算匹配率,针对一次识别匹配率相近的情况重点区分,去除误判。本文的方法相较基于像素点的模板匹配算法,准确率明显提高;相较基于特征的模板匹配算法,模板库的特征优选问题得以改善,弥补了两种模板匹配算法各自的缺点,实现了系统的目标。
本文的方法简单,识别率高,主要适用于工件种类较少的分拣作业场合。
NOTES
*通讯作者。