1. 引言
室内装饰性的墙纸纹理具有色彩多样、图案丰富的特点,一般来说,墙纸都是有着不同风格的,如图1所示,既有巴洛克风格的墙纸,又有现代风格的墙纸。墙纸纹理一直以来在各种领域都得到了广泛的应用,例如书籍封皮、室内装修、路标商标等等。这引起了许多墙纸纹理设计公司、墙纸售卖者的广
泛关注。对于墙纸设计公司而言,他们需要辨别一幅特定的墙纸纹理是怎样一种设计风格;对于墙纸纹理售卖者来说,他们需要针对不同的房间场景,为客户推荐合适的纹理。不同的房间中的装饰墙纸也都具有独特的风格,适用于卧室,客厅,浴室等不同场景中的墙纸都有很大的区别。然而到目前为止,要判断一幅纹理图像的设计风格,或者分析其所适合装饰的房间不同风格的墙纸纹理,都需要人们用眼睛不断地观察然后判断。这个过程非常耗费人力,耗费时间,并且在人眼产生视觉疲劳的状态下,容易造成判断失误。从这个角度来讲,利用计算机视觉的方法寻找一个符合实际的并且有效的解决途径,使其既能够学习墙纸纹理的设计风格,又可以对墙纸比较适合装饰什么样的房间进行推荐,具有十分重要的意义。
2. 数据库
2.1. 数据采集
与墙纸风格相关的研究工作 [1] 目前比较少,同时具有风格标签和适宜装饰的房间的标签的数据集更是凤毛麟角。根据Cimpoi等人 [2] 在其工作中收集数据集DTD (Describing Textures Dataset)的经验,本文同样采取网站获取数据集的方法,通过网络进行了墙纸纹理数据的收集工作。我们定义了卧室(bedroom)、客厅(living room)、浴室(bathroom)、儿童房(children’s room)共四种类型的场景和现代风格(modern style)、巴洛克风格(baroque style)、花状(floral style)、奢华风格(luxury style)、清新风格(fresh style)、乡村风格(country style)六种墙纸纹理风格来搜集对应的墙纸。
在收集墙纸纹理图像的过程中,我们使用两种方式对符合条件的墙纸进行筛选:
1) 根据墙纸销售网站 [3] [4] [5] 上所提供的不同标签下的图像分别进行下载,可以为我们提供bedroom、living room、children’s room以及bathroom等场景中所有的墙纸图像;而wallpaper from the 70s [5] 网站有多个风格样式的墙纸图像可供我们下载;
2) 借助谷歌搜索引擎进行数据搜索。我们在搜索栏中输入不同的墙纸风格,或者不同的房间场景,搜索墙纸图像,根据引擎的搜索结果进行筛选。例如,输入“bathroom wallpaper”,搜索墙纸图片,然后选择分辨率比较清晰且大小合适的墙纸进行下载。
按照上述思路进行墙纸图像采集之后,最终总共六个风格,四种场景共计1300多张图像。
2.2. 数据处理
由于通过网站和搜索下载得到的墙纸图像复杂多样,于是造成了以下两个问题:
1) 个别的图像并不是特别符合对应类别的要求。例如,在浴室类别的所有图像中,我们发现,其中混杂了并不是特别符合浴室场景的墙纸图像;
2) 来源不同的图像,其尺寸、分辨率均不同,对后续的模型训练造成一定的困扰。
对于这样的情况,我们采取了以下措施:
a) 手工筛掉不符合条件的图像,保留符合要求的和分辨率高的;
b) 通过程序实现图像尺寸的裁剪,使得所有图像保持一致大小。
经过上述处理,在最终获得的墙纸数据集中,六个风格描述的墙纸类别下分别含有100幅~200幅不等数量的墙纸,四个房间类别下墙纸图像也均包含100幅。图像尺寸最终裁剪为390 × 300。具体见图2与图3。图2为数据集中四种不同场景的墙纸示例,从左到右,从上往下依次是适用于浴室、卧室、儿童房和客厅的墙纸纹理图像。图3为数据集中六种不同风格的墙纸示例,从左到右,从上往下依次是巴洛克风格、花状风格、清新风格、奢华风格、现代风格和乡村风格的墙纸纹理图像。

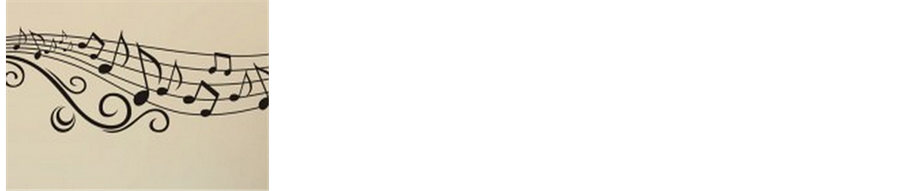
Figure 2. Illustration of wallpapers for four different scenes
图2. 四种场景的墙纸图片示例
3. 模型构建
3.1. 特征提取
Gabor特征:
Gabor [6] [7] 特征是被认为是一种符合人类视觉感知的特征。给定一幅大小为P × Q的图像I(x, y),它的Gabor小波变换可以通过卷积形式定义:
(1)
其中,s和t用来表示滤波器的尺寸,x和y用来表示像素在图像中的位置。我们采用了4个尺度6个方向的滤波器提取了墙纸纹理的Gabor特征,最终的特征维度是48维。
ScatNet提取的特征:
ScatNet (Scattering Network) [8] 是信号或者图像的一种散射变换的形式,这种特征变换可以应用于语音信号、多重分形和图像的分类问题上。论文 [9] [10] [11] [12] 介绍了如何利用ScatNet特征进行应用。在使用ScatNet对一幅2D的图像进行特征变换时,ScatNet依赖于三种数据结构,并对其进行相互嵌入:层数、小波族函数和滤波器组。
1) 层
2D图像x的前三个散射层是按照式(2)、式(3)和式(4)定义的:
(2)
(3)
(4)
其中,公式中的符号*代表空间域的卷积操作,
是平均滑动窗口,而
是以
次幂放大后的小波,并且按照
进行旋转变换后得到的;输出变量S是一个元胞数组,它包含着来自不同层的散射变换后的元素:
就对应着上述三个公式中的
变量。而散射层是matlab的一种变换结构,它具有两个域:
A. 信号(signal)
散射层的信号是一个二维的元胞数组矩阵,这里面融合了散射变换所需要的参数,式(5)中用变量p对该参数进行索引:
(5)
B. meta是包含j和θ的序列结构,它们都对应于每个p,如式(6)所示:
(6)
2) 族函数
ScatNet所使用的全部都是线性运算器。一个标准的族群是按照下式的规范建立的:
(7)
scat.m文件中是根据式(8)进行层层迭代的:
(8)
第m个运算符
是要在第m个内部层
上进行计算的。运算符输出第m个外部层
和一个剩余层V。第二行公式是对剩余层V进行了一个复杂的系数变换,然后将结果存储于第
个内部层
中,以便后续进行分解。
3) 滤波器组
散射变换运算中的滤波器可以作为小波家族函数输出的第二个参数,具体见式(9):
(9)
经过以上描述,将2D墙纸图像送入ScatNet网络后,可以得到对应的特征表示。由于经过多个滤波器后再连接的特征维度非常高,本文在实验中进一步执行了降维操作。本部分采用的是PCA (Principal Component Analysis)降维,PCA又名主成分分析,是人们常用的降维手段。其主要原理在于求解高维数据的协方差矩阵并求解特征值及特征向量,然后按照特征值降序排列的顺序,取前k个特征向量,将输入样本X降维至k维,降维后的样本数据表示为
。PCA降维在计算过程中一般不需要手动确定降维后的维数,即k的值,而是参考累计贡献率变量来决定最终降维后的数据。
经过ScatNet变换后的图像特征再次经过PCA降维后,风格类墙纸纹理的特征维度由2329362维降至30维,适用于不同室内房间的墙纸维度降至292维。
3.2. 模型训练
墙纸纹理的风格描述既可以看作墙纸的一种标签,为此,我们构建了相应的模型,使其既能够为墙纸纹理图像自动添加风格描述,又可以为其推荐所适于装饰的房间。图4展示了本文对墙纸纹理展开风格分析的整体架构。
该架构描述了我们预测墙纸纹理风格的步骤和方法,总体的思路是在收集的数据的基础上通过训练不同的分类模型,为测试图像自动预测风格语义并推荐适用的应用场景。本文用两种方式实现图4中所示的训练模型;
1) 传统方法
传统的机器学习方法对数据集进行分类操作,往往是将特征提取和分类器训练分离开来,即“特征 + 分类器”模式;在本文,墙纸纹理的图像特征采取上述Gabor特征和ScatNet两种特征对其进行表示;分类器将采用最常用的Support Vector Machine, 即SVM分类器。
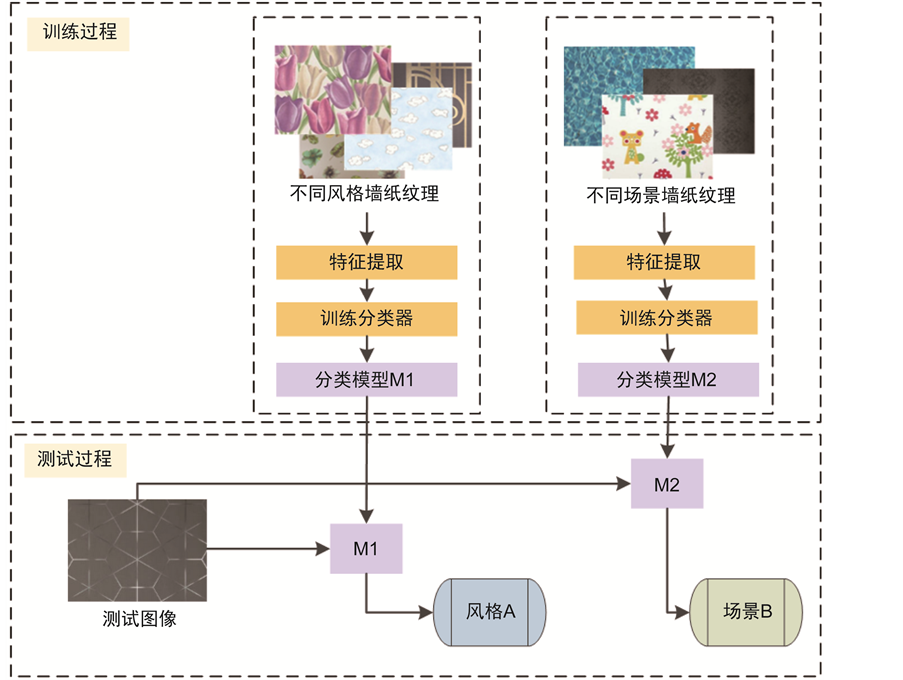
Figure 4. The flow chart of wall paper texture style analysis and scene recommendation
图4. 墙纸纹理的风格分析及场景推荐流程图
在训练过程中,我们将不同风格的墙纸和适用于不同室内场景的墙纸作为两个数据集分别进行特征提取和SVM训练。
2) 深度卷积神经网络(Deep Convolutional Neural Network)
对于分类问题,深度卷积神经网络一直展示着其巨大的优势。于是在本次实验中,本文采用微调的办法在规模较小的墙纸数据集上优化分类效果,争取取得更优的模型。在本次实验中,我们选择了经典的AlexNet网络模型,并且选择了2012年ImageNet大规模数据竞赛最优的模型 [12] ,在此基础上,用墙纸纹理的分类任务对模型进行微调。
4. 实验结果分析
在学习墙纸纹理的风格时,我们训练一个分类模型M1;而为了更进一步为墙纸纹理推荐其所适用于的室内场景,我们训练另外一个分类模型M2。在上述两个分类器中训练的结果如表1和表2所示。
如图5所示,当得到一幅纹理图像的时候,我们的模型可以为其自动标注风格,并且推荐合适的房间场景。而从表1和表2又可以看出,该应用具有较大的实际意义,同时也在小规模的数据集上取得了不错的训练结果,但训练结果仍然有待提高。分析原因如下:1) 同一幅墙纸纹理可以同时具有多个风格,即多个风格标签,因此墙纸纹理数据集的准备过程非常有必要为每一幅纹理增加多个风格标签;2) 颜色对墙纸纹理是十分重要的特性,也是影响人们选择的重要因素,后续的工作可以采用一些对颜色更敏感的特征对墙纸进行表示;3) 不同风格的墙纸纹理之间,其纹理脉络或者基元会“迷惑”模型的学习原因,使得分类准确率无法提高;4) 总体来讲,数据量有待扩大。墙纸纹理的风格(或者称为不同的模式)是需要提供大量的图像供机器进行学习,机器才有可能抓取不同风格的墙纸图像的关键特点加以区分。
5. 结束语
本文以室内装饰性的墙纸为切入点,使用特征学习与SVM分类器构建了墙纸纹理的风格学习和应用场景推荐系统,实现了针对输入墙纸纹理图像进行风格分析预测,并对该墙纸纹理所适用的场景进行了
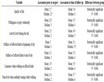
Table 1. The classification results of styles with different features
表1. 不同特征针对风格类实验的分类结果
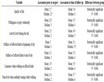
Table 2. The classification results of scenes with different features
表2. 不同特征针对室内场景类实验的分类结果

Figure 5. Illustration of style prediction and scene recommendation
图5. 墙纸纹理风格预测与场景推荐效果示例
推荐。该应用在商业和设计行业具有较大的是非常有意义的。本文中提出的方法可以有效的对墙纸纹理的风格和适用的场景进行有效的预测和推荐,但实验部分的结果仍有待提高。分析问题例如对于同一幅墙纸纹理的多种风格标签的整理与采集、多风格的墙纸纹理的分析和预测、建立虚拟的视觉场景使人们能够直观地观察到自己所选的纹理在某个室内场景的装饰效果等等。此外,进一步提高模型的准确程度也是后续工作需要解决的问题。
基金项目
青岛农业大学高层次人才启动资金项目编号1117006基于图像的棉花品级自动分类研究;
国家自然科学基金项目编号61271405符合视觉感知机理的自然纹理生成模式研究;
教育部博士点专项基金项目编号20120132110018基于视觉感知特征创意的纹理生成模型研究。