1. 引言
在过去的一段时间里,具有不可积约束的欠驱动系统的控制越来越受到人们的关注 [1] 。由于Brockett条件的限制,这样的系统不是局部线性可控的,并且不能通过任何光滑(甚至连续)的时不变控制律渐近稳定 [2] [3] 。为了克服这一困难,提出了许多新的非线性方法。所提出的具有不可积约束的欠驱动系统的稳定解包括光滑时变控制律、不连续或分段光滑控制律和混合控制律 [4] [5] [6] 。以往的工作大多集中在具有速度不可积约束的非完整系统上。在许多实际应用场景比如具有欠驱动系统由于两轮自平衡机器人,其具有内在的的不稳定性,通过对其进行主动控制保持其直立位置,其轮子被独立驱动以提供需要机器人保持平衡的力(力矩),各种线性和非线性控制系统被设计出来。欠驱动船舶是一种重要的民事和军事工具,其控制问题近年来显得尤为重要。 [7] 中提出了一种连续反馈控制律,保证位置变量指数收敛到其期望值,并且方向变量收敛到某个常数值。在 [8] 中,提出了一种不连续反馈控制律,在初始状态的某些假设下,提供所有状态到其期望值的指数收敛。 [9] 中,提出了一种时变反馈控制律,在吸引池大小未知的情况下,实现所需平衡点的局部指数(相对于给定膨胀)稳定性。 [10] 中,提出了一种时变反馈控制律,以确保运动船舶模型的半全局实际指数稳定性,其中喘振和偏航速度作为控制输入。基于另一简化模型。
上述关于欠驱动水面舰艇稳定性的文献综述表明,实现全局指数收敛于所需设定点的控制律,如 [11] [12] [13] [14] [15] 中提出的控制律甚至不能保证所需设点的局部渐近稳定性;实现闭环系统全局一致渐近稳定的控制律,如 [16] [17] [18] [19] [20] 中提出的控制律是时变的,并且只产生对所需设定点的渐近收敛。对这种欠驱动系统,传统的渐进稳定控制方法设计起来变得越来越困难,本文采用有限时间控制策略,对水面船舶进行有效控制。
2. 控制对象运动数学模型
本文的研究对象为欠驱动水面船舶,其运动示意图如图1所示。对于多数欠驱动水面航行器,通常忽略其在垂直面内的运动情况,即只考虑其在纵向、横向和转向运动,本文针对欠驱动水面船舶控制跟踪问题,旨在找到稳定、响应时间快,控制精度高的解决方法,借助于fossen [19] 所建立的船舶水平面运动方程,假设以下条件成立:
1) 船体的惯性主轴和船体坐标系重合:
2) 船体的几何重心为船体坐标系原点;
3) 船体及所载重货物质量均匀分布;
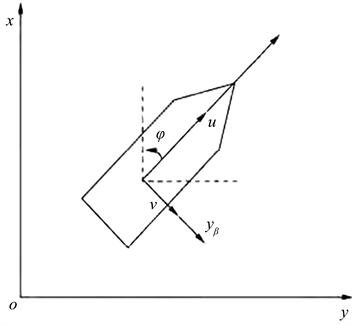
Figure 1. Schematic diagram of the navigation coordinate system of surface ships
图1. 水面船舶航行坐标系示意图
(1)
(1)式中,u,v和r分别为船体坐标系中纵向、横向和角速度;x,y,φ为惯性系中船舶的位置和方向。
,
是水面船舶的惯性和附加质量系数。
,
为水面船舶的水动力系数。可用的控制输入为螺旋桨推力
和转向力矩
。侧向一般不配备单独的推进器。
假设有如下的参考轨迹
满足
(2)
令
,
其中T为如下可逆矩阵
由数学知识可知,
均为正数。故以上的坐标变换为可逆变换。
对参考轨迹做相同的变换,有
, (3)
,
令
则有如下的跟踪误差动力学方程
(4)
3. 控制器设计策略
考虑误差系统(4),则如下的控制律
(5)
以及未知有上界的扰动自适应估计律
(6)
则可以所讨论的被控对象扰动条件下跟踪误差的有限时间收敛。
(5)式中,
均为正数。
(6)式中,
为自适应系数,
定义为
(7)
其中
,
,
为任意小的正数,
为
的估计值,
,
为最大估计值。
证明:由(4)可知,当
时,
有界,且
有限时间收敛。由式(5)可知,
为渐进收敛,由(4)知道
也为有限时间收敛量。把
看做输入变量,由输入状态稳定理论,可知
有界且可以在有限时间内收敛于0。
因为
,
李雅普诺夫函数选取如下
对时间求导并有
,
,利用式(6),有
(8)
由(8)可知,
是有界的,由Babalat引理 [20] ,可知
是有限时间收敛的。证明完毕。
4. 仿真结果
水面船舶的系统参数为
螺旋桨推进器的推力为
,最大扭矩为
。
参考轨迹设置如下。
初始条件为
其他控制参数选择为
在MATLAB-SIMULINK程序中按照设定的增益运行仿真程序,误差的收敛情况如下图2。
5. 结果分析
由仿真结果可以看出,所设计的控制算法可以实现对船舶位置和航向良好的同步跟踪控制,所设计的有限时间控制器对环境干扰具有一定的鲁棒性能,但对横向常值扰动的抗干扰能力较弱。今后的工作将重视解决这一问题。