1. 引言
肝细胞癌(hepatocellular cancer, HCC)是人类癌症中癌症相关死亡的常见原因 [1]。与其他恶性肿瘤相比,HCC的发病率在男性中排名第五,在女性中排名第九,在全球癌症死亡人数中占第二位 [2]。HCC预后不良,1年和3年生存率分别为36%和17% [3]。虽然肝细胞癌在手术切除、化疗、射频消融、全身治疗、肝移植等方面取得了很大的进展,但由于大部分患者处于晚期,其预后仍然较差 [4]。显然,我们还需要探索新的方法来预测HCC的预后。
铁是所有哺乳动物血红蛋白合成,DNA合成和能量代谢所必需的营养素。铁代谢也是一个重要的生物过程,包括线粒体呼吸,代谢和解毒,DNA合成,抗氧化防御,氧传感和免疫防御 [5]。在肿瘤中发现了一种依赖于铁代谢新型的细胞死亡,称为铁死亡 [6] [7]。一些研究表明,铁代谢涉及多种类型的癌症,包括肝细胞癌,膀胱癌,乳腺癌,结肠直肠癌,胃癌,肺癌,黑色素瘤和胰腺癌 [8] [9]。细胞中的铁代谢主要包括由许多基因和蛋白质调节的摄入,利用和外排过程 [10] [11] [12]。铁代谢相关基因主要由铁摄入基因(TFRC和DMT1),利用基因(FTH1,FTL,HIF1A,HMOX1,SLC25A37和SLC25A38)和外排基因(FLVCR1和SLC40A1)组成 [13] [14]。
为了探讨铁代谢与HCC预后的关系,以及铁代谢相关基因是否可作为HCC预后的潜在生物标志物,指导临床用药。我们使用HCC患者的RNA微阵列和临床数据来开发预后模型作为预测HCC患者存活的独立生物标志物。
2. 材料和方法
1) 数据下载和处理
根据现有文献,获得了潜在的铁代谢相关基因,主要包括TFRC,DMT1,FTH1,FTL,HIF1A,HMOX1,SLC25A37,SLC25A38,FLVCR1和SLC40A1。从TCGA数据库下载由374个HCC和50个相邻正常组织组成的RNA微阵列和临床数据。374例HCC和50例癌旁正常组织铁代谢相关基因的差异表达用均值功能处理,均值表达水平用log2转化归一化。我们通过limma软件包分析肿瘤组织与正常组织中铁代谢相关基因的差异表达,阈值为错误发现率(false discovery rate, FDR) < 0.05和 |log2倍数变化(fold change, FC)| > 1。
2) 铁代谢相关基因的一致性聚类分析
在R语言中使用ConsensusClusterPlus进行一致性聚类分析将所有肝癌患者分为不同的组 [14]。然后采用Kaplan-Meier法计算各组间总生存期(OS)的差异。用卡方检验比较年龄、性别、年级、分期在不同群体间的分布。
3) 基于铁代谢相关基因的预后模型
通过单因素Cox回归分析铁代谢相关基因的表达情况,得到与生存显著相关的基因。然后,我们采用多变量Cox回归分析去除与生存高度相关的铁代谢相关基因。我们根据预后模型计算每个HCC患者的风险评分。风险评分的计算如下:风险评分 =
(vi为基因的平均表达水平,ci为基因的回归系数)。根据预后模型,绘制Kaplan-Meier生存曲线,通过对数秩检验对高危组和低危组进行评估。我们通过生成OS相关ROC曲线来确定预测模型的准确性。独立预后分析是我们预测预后模型是否可以作为肝癌患者的独立预后因素。
4) 肿瘤浸润免疫细胞的预后模型
CIBERSORT是一种分析工具,可使用基因表达数据估算肿瘤活检的浸润性免疫组成。在这项研究中,我们通过CIBERSORT方法量化肝细胞癌样本中免疫细胞的分数。由CIBERSORT产生的免疫细胞群的推断部分的结果被认为是准确的,阈值P < 0.05 [15]。
5) 通过Oncomine数据库、The Human Protein Atlas和Kaplan-Meier plotter验证铁代谢相关基因
Oncomine数据库,癌症微阵列数据库和基于web的数据挖掘平台,用于挖掘癌症基因信息 [16]。Oncomine数据库用于常见癌症类型及其各自正常组织的差异表达分类,以及临床和病理分析。人类病理学地图集允许为癌症患者生成个性化的基因组规模的代谢模型,以鉴定参与肿瘤生长的关键基因。在这项研究中,我们利用Oncomine数据库和人类蛋白质图谱来探索HCC组织和肝组织中铁代谢相关基因的蛋白质表达。通过Kaplan-Meier绘图仪在线工具验证RBP在HCC中的预后价值。
6) 验证预后模型
我们的预后模型由国际癌症基因组联盟(ICGC)数据库进行外部验证。每个HCC患者的风险评分通过相同的公式计算。ICGC数据库主要包含116名日本HCC患者和邻近的正常组织。
7) 统计分析
所有统计分析均使用Perl语言和R语言进行。绘制Kaplan-Meier曲线以显示高风险组和低风险组之间的存活差异,并进行对数秩检验以确定差异的显着性。所有比较统计学显著性定义为P < 0.05。
3. 结果
1) 铁代谢相关基因表达差异
生成热图以显示肿瘤组织和正常组织之间m6A甲基化相关基因的表达水平(图1)。肿瘤组织中SLC11A2、FLVCR1、FTH1、TFRC、SLC25A38和FTL的表达水平显著高于正常组织。肿瘤组织中HMOX1的表达水平显著降低。
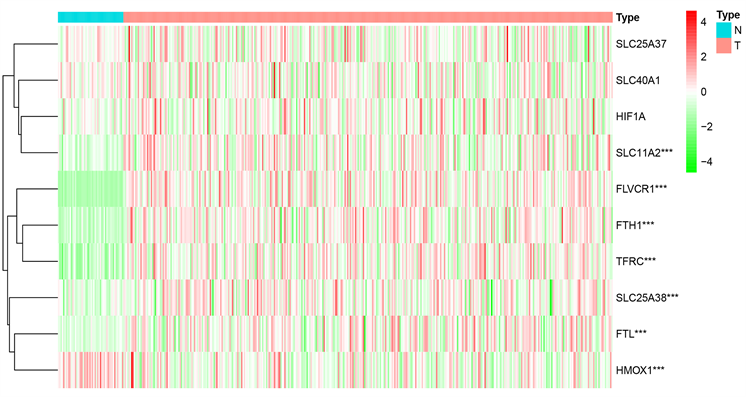
Figure 1. Heatmap of differentially expressed iron metabolism-related genes between HCC and normal tissues
图1. HCC和正常组织之间差异表达的铁代谢相关基因的热图
2) 铁代谢相关基因的一致性聚类分析
一致性聚类分析显示,最合适的选择是将HCC患者分为两类(图2(A)~(D))。评价聚类与临床病理特征的关系。组1和组2之间的肿瘤T状态和分期存在显著差异(图2(E))。此外,Kaplan-Meier曲线显示,组1的5年OS显著长于组2 (P = 0.006) (图2(F))。
3) 基于铁代谢相关基因的预后模型的建立和验证
共鉴定出4个铁代谢相关基因(FLVCR1,FTL,HIF1A,HMOX1)构建预后模型。我们基于4个铁代谢相关基因的预后模型使用以下公式形成:风险评分 = (FLVCR1*0.16713的平均表达) + (FTL*2.52E−05的平均表达) + (平均表达) HIF1A*0.014361) + (HMOX1*0.002315的平均表达)。
根据风险评分的中位数水平,HCC患者分为低风险组和高风险组。Kaplan-Meier生存曲线显示,低风险组的生存率高于高风险组(HR = 1.329,95%CI = 1.210~1.461,P < 0.001) (图3(A))。此外,我们通过构建ROC曲线评估了OS相关预后模型的准确性,风险评分的AUC显著高于其他临床病理参数(图3(B))。最后,我们通过预后模型对HCC患者进行排序以分析生存分布。我们可以根据风险评分确定HCC患者的死亡率。此外,随着风险评分的增加,患者的死亡率上升(图3(C),图3(D))。
我们通过相同的公式计算了ICGC数据门户项目肝癌RIKEN,JP (LIRI-JP)中每位HCC患者的风险评分作为独立的外部验证。HCC患者根据风险评分的中位数分为高危组和低危组。Kaplan-Meier生存曲线显示了我们的预后模型的预后价值(P < 0.001) (图3(E))。此外,ROC曲线还显示OS相关预后模型预测HCC患者预后的良好能力(图3(F))。随着风险评分的增加,患者的死亡率上升(图3(G),图3(H))。因此,这些验证结果证实了我们的预后模型的预测预后能力。
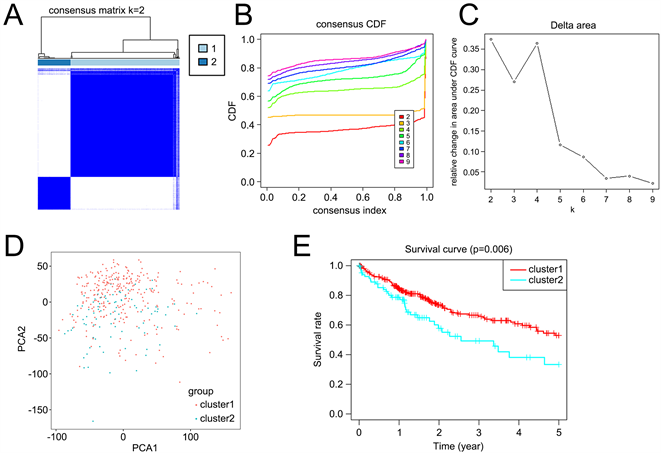
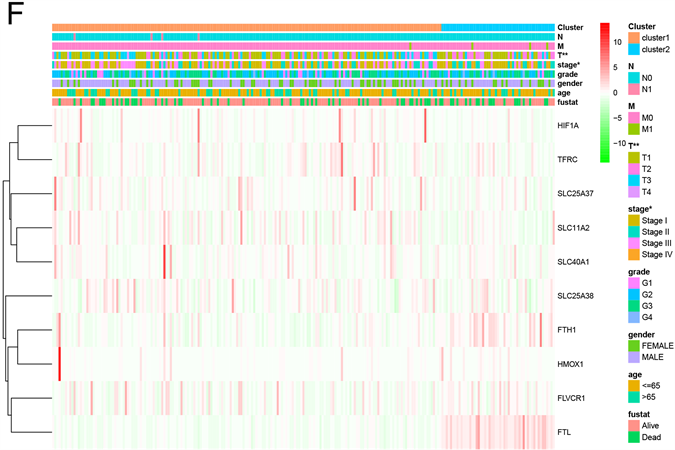
Figure 2. Differential overall survival and grade of HCC patients in the two different clusters. (A) The HCC patients divided into two distinct clusters, k = 2; (B) Consensus clustering cumulative distribution function for k = 2~9; (C) Relative change in area under cumulative distribution function curve for k = 2~9; (D) The principal components analysis of the iron metabolism-related genes; (E) Kaplan-Meier curve of cluster 1 and cluster 2; (F) Heatmap of expression of iron metabolism-related genes and clinicopathological features between cluster 1 and cluster 2
图2. 两组不同肝癌患者的总体生存率和分级差异。(A) HCC患者分为两个不同的聚类,k = 2;(B) 一致聚类累积分布函数k = 2~9;(C) 累积分布函数曲线下面积的相对变化k = 2~9;(D) 铁代谢相关基因的主成分分析;(E) 簇1和簇2的Kaplan-Meier曲线;(F) 簇1和簇2之间铁代谢相关基因表达和临床病理特征的热图
4) 预后模型,临床病理参数与肿瘤浸润免疫细胞的关系
单变量和多变量Cox回归分析显示预后模型可以作为HCC患者的独立预后因素(图4(A),图4(B))。此外,预后模型与临床病理特征显着相关,如生存状态(p = 0.001),分期(p = 0.002),分级(p = 0.001)和肿瘤T状态(p = 0.002) (图4(C)~(F))。
接下来我们用CIBERSORT算法评估了肝癌微环境中肿瘤浸润免疫细胞与预后模型的关系。CIBERSORT分析显示,肝癌高危组和低危组22种免疫细胞类型的组成差异显著(图5(A),图5(B))。巨噬细胞M0在高危组更为富集,而T细胞CD8、NK细胞活化在低危组更为富集(图5(C))。
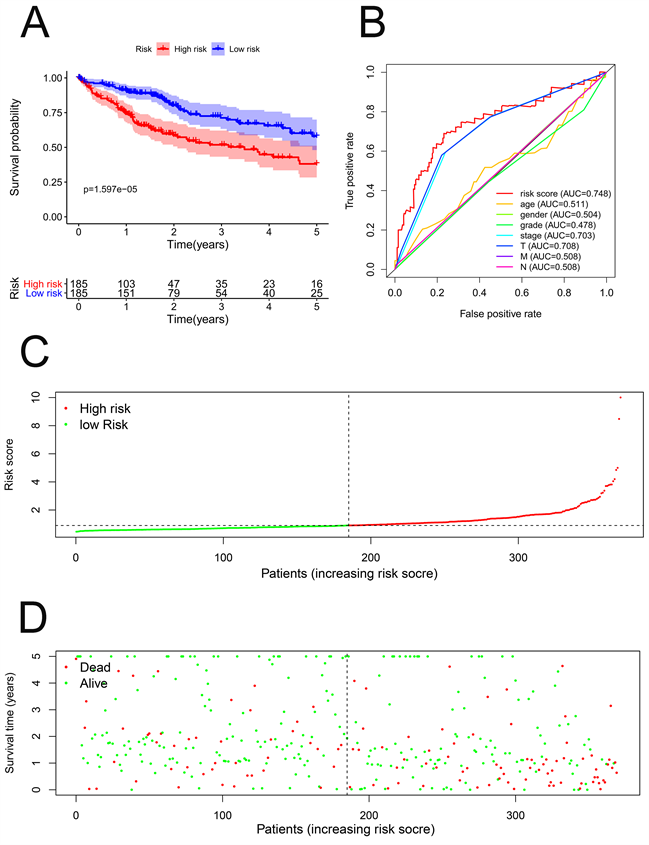
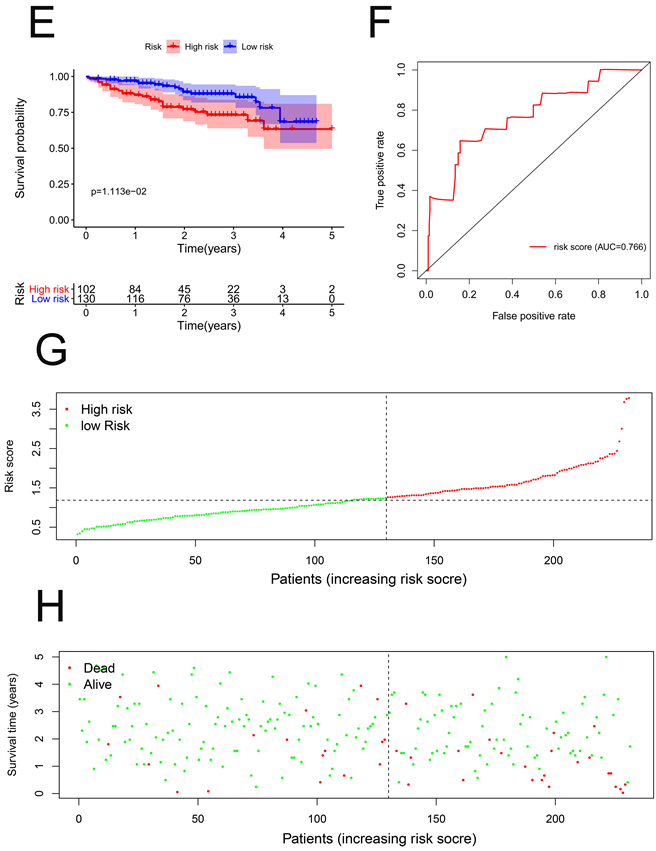
Figure 3. Prognostic model of hepatocellular carcinoma patients in TCGA and ICGC cohort. (A) Kaplan-Meier curve of high-risk and low-risk HCC patients in TCGA cohort; (B) ROC curve of OS-related prognostic model in TCGA cohort; (C) Risk score distribution of HCC patients with different risks in TCGA cohort; (D) Scatterplots of HCC patients with different survival status in TCGA cohort; (E) Kaplan-Meier curve of high-risk and low-risk HCC patients in ICGC cohort; (F) ROC curve of OS-related prognostic model in ICGC cohort; (G) Risk score distribution of HCC patients with different risks in ICGC cohort; (H) Scatterplots of HCC patients with different survival status in ICGC cohort
图3. TCGA和ICGC队列中肝细胞癌患者的预后模型。(A) TCGA队列中高危和低危HCC患者的Kaplan-Meier曲线;(B) TCGA队列中OS相关预后模型的ROC曲线;(C) TCGA队列中不同风险HCC患者的风险评分分布;(D) TCGA队列中不同生存状态HCC患者的散点图;(E) ICGC队列中高危和低危HCC患者的Kaplan-Meier曲线;(F) ICGC队列中OS相关预后模型的ROC曲线;(G) ICGC队列中不同风险HCC患者的风险评分分布;(H) ICGC队列中不同生存状态HCC患者的散点图
5) 铁代谢相关基因在肿瘤数据库、人类蛋白质图谱和Kaplan-Meier绘图仪中的表达
利用Oncomine数据库分析肝癌组织中FLVCR1、FTL、HIF1A、HMOX1的表达水平。在Wurmbach肝(222906_at)、Chen肝(IMAGE:1575419)、Roessler肝(200989_at)中,不同肝细胞癌中FLVCR1、FTL、HIF1A的表达水平均高于正常组(图6(A)~(C))。然而,HMOX1在肿瘤中的表达水平没有意义。此外,我们通过人类蛋白质图谱数据库验证了FLVCR1、FTL、HIF1A、HMOX1的组织学水平,结果显示,FLVCR1、FTL、HIF1A、HMOX1在肝癌组织中上调,在正常组织中下调(图6(D)~(G))。用Kaplan Meier-plotter检测FLVCR1、FTL、HIF1A、HMOX1的预后意义。结果显示,FLVCR1、FTL、HIF1A、HMOX1与HCC患者的OS密切相关(图7(A)~(D))。
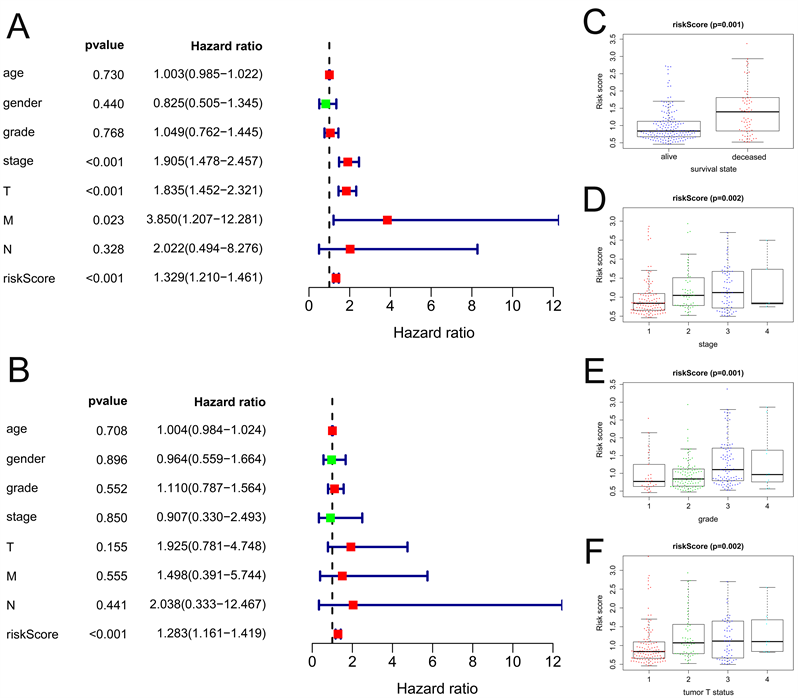
Figure 4. The relationships between prognostic model, clinicopathological parameters. (A) Univariate factor independent prognostic analysis; (B) Multivariate factor independent prognostic analysis; (C) The relationships between prognostic model and survival state; (D) The relationships between prognostic model and stage; (E) The relationships between prognostic model and grade; (F) The relationships between prognostic model and tumor T status
图4. 预后模型与临床病理参数之间的关系。(A) 单因素独立预后分析;(B) 多因素独立预后分析;(C) 预后模型与生存状态的关系;(D) 预后模型与分期的关系;(E) 预后模型与分级的关系;(F) 预后模型与肿瘤T状态的关系
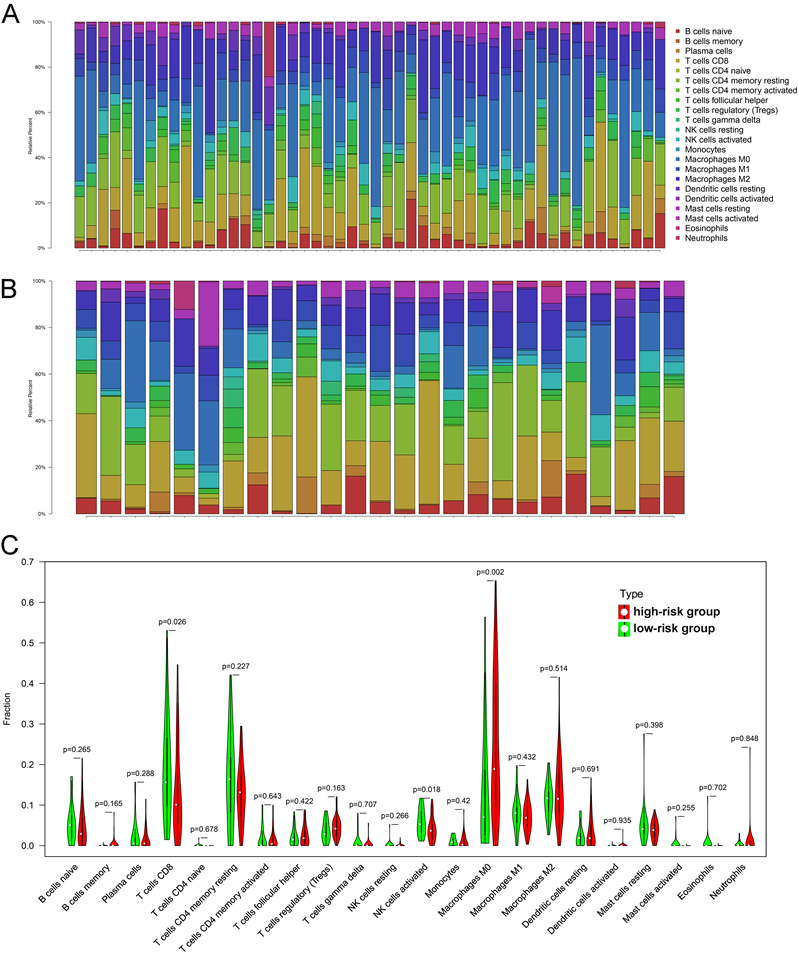
Figure 5. The relationships between prognostic model and tumor-infiltrating immune cells. (A) 22 immune cells in High-risk group; (B) 22 immune cells in low-risk group; (C) Violin plot displays the differentially infiltrated immune cells between High-risk group and low-risk group
图5. 预后模型与肿瘤浸润性免疫细胞之间的关系。(A) 高危组22个免疫细胞;(B) 低危组22个免疫细胞;(C) 小提琴图显示高风险组和低风险组之间差异浸润的免疫细胞
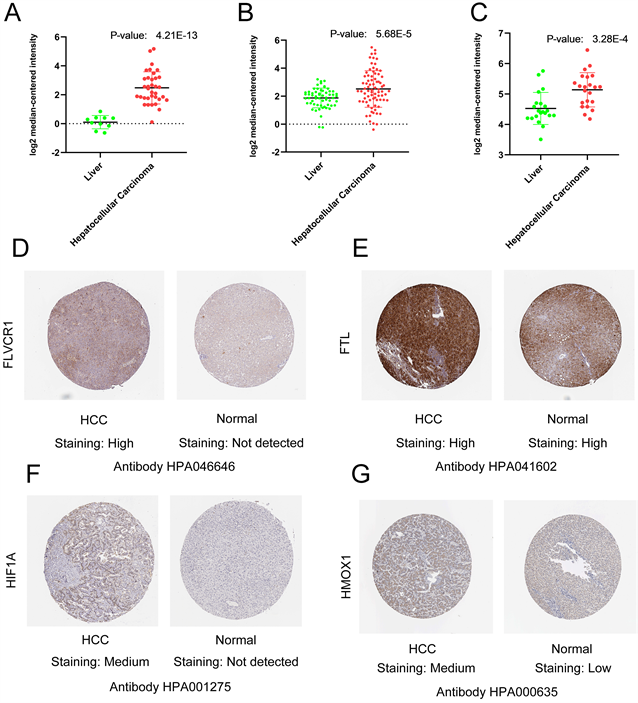
Figure 6. The expression level of FLVCR1, FTL, HIF1A, HMOX1 in the Oncomine database, and The Human Protein Atlas. (A) Expression level of FLVCR1 in HCC and Liver in Oncomine database; (B) Expression level of FTL in HCC and Liver in Oncomine database; (C) Expression level of HIF1A in HCC and Liver in Oncomine database; (D) Immunohistochemistry results of FLVCR1 in HCC (Staining: High; Intensity: Strong; Quantity: 75%~25%; Location: Cytoplasmic/membra) and in normal tissue (Staining: Not detected; Intensity: Negative; Quantity: None; Location: None); (E) Immunohistochemistry results of FTL in HCC (Staining: High; Intensity: Strong; Quantity: >75%; Location: Cytoplasmic/membranous) and in normal tissue (Staining: High; Intensity: Strong; Quantity: >75%; Location: Cytoplasmic/membra); (F) Immunohistochemistry results of HIF1A in HCC (Staining: Medium; Intensity: Moderate; Quantity: >75%; Location: Cytoplasmic/membranous/nuclear) and in normal tissue (Staining: Not detected; Intensity: Negative; Quantity: None; Location: None); (G) Immunohistochemistry results of HMOX1 in HCC (Staining: Medium; Intensity: Moderate; Quantity: 75%~25%; Location: Cytoplasmic/membranous) and in normal tissue (Staining: Low; Intensity: Weak; Quantity: 75%~25%; Location: Cytoplasmic/membranous)
图6. 肿瘤数据库和人类蛋白质图谱中FLVCR1、FTL、HIF1A、HMOX1的表达水平。(A) 肿瘤数据库中肝癌和肝脏中FLVCR1的表达水平;(B) 肿瘤数据库中肝细胞癌和肝脏中FTL的表达水平;(C) 肿瘤数据库中肝癌和肝脏中HIF1A的表达水平;(D) 肝癌中FLVCR1的免疫组化结果(染色:高;强度:强;数量:75%~25%;位置:细胞质/膜)和正常组织(染色:未检测到;强度:阴性;数量:无;地点:无);(E) 肝癌中FTL的免疫组化结果(染色:高;强度:强;数量:>75%;位置:细胞质/膜)和正常组织(染色:高;强度:强;数量:>75%;位置:细胞质/膜);(F) HCC中HIF1A的免疫组织化学结果(染色:培养基;强度:中等;数量:>75%;位置:细胞质/膜质/核)和正常组织(染色:未检测到;强度:阴性;数量:无;地点:无);(G) HCC中HMOX1的免疫组织化学结果(染色:培养基;强度:中等;数量:75%~25%;位置:细胞质/膜)和正常组织(染色:低;强度:弱;数量:75%~25%;位置:细胞质/膜质)
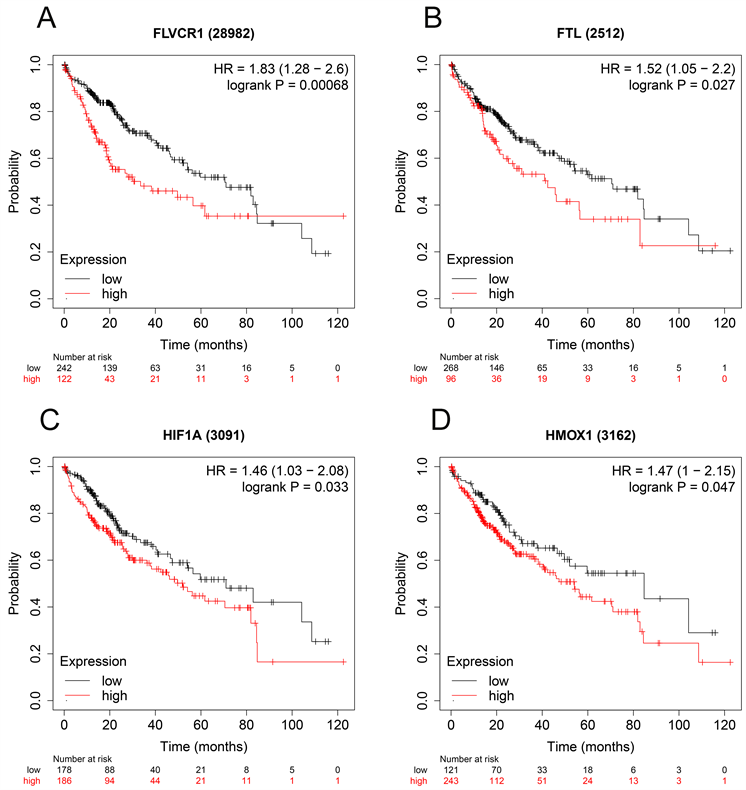
Figure 7. Validation the prognostic value of FLVCR1, FTL, HIF1A, HMOX1 in HCC by Kaplan Meier-plotter. (A) FLVCR1; (B) FTL; (C) HIF1A; (D) HMOX1
图7. 通过Kaplan-Meier绘图仪验证FLVCR1、FTL、HIF1A、HMOX1在肝癌中的预后价值。(A) FLVCR1;(B) FTL;(C) HIF1A;(D) HMOX1
4. 讨论
肝癌的发生、发展和治疗是一个复杂的调控网络。与单一的临床病理参数相比,收集多种生物标志物并建立预后模型是预测肿瘤预后更有效的方法。目前,铁代谢在包括肝细胞癌在内的多种癌症中起着重要作用。铁代谢在癌症的发生、发展中普遍存在。基于铁代谢相关基因的预后模型可能比单一的临床病理参数更精确。
本研究旨在探讨铁代谢相关基因与肝癌患者预后的关系。我们从TCGA数据库中获得了铁代谢相关基因的RNA表达谱。然后,我们确定了4个铁代谢相关基因(FLVCR1、FTL、HIF1A、HMOX1),并通过Cox回归分析构建了预后模型。最后,以ICGC队列为例,用同一公式对预测模型进行了验证。预后模型可作为肝癌患者独立的预后生物标志物。
CIBERSORT分析显示,T细胞CD8、NK细胞活化在低危组更为集中,巨噬细胞M0在高危组更为富集,而巨噬细胞M0在高危组更为富集。CD8+T细胞是抗肿瘤免疫的主要效应细胞,它们在肿瘤部位的存在与良好的预后相关 [17] [18]。一些研究表明,浸润人类转移性黑色素瘤的CD8+T细胞的相对丰度与免疫治疗的临床反应密切相关 [19] [20]。肿瘤微环境中CD8+T细胞的数量和质量在决定启动免疫治疗后是否会产生针对肿瘤的强大免疫应答方面起着重要作用 [18]。同时,NK细胞是天然免疫系统的关键组成部分,具有强大的抗肿瘤和抗转移活性 [21]。但巨噬细胞M0与肝癌的关系尚不清楚。这些结果表明,预后模型中的低危患者可能对免疫治疗敏感。
FLVCR血红素转运体1 (FLVCR heme transporter 1, FLVCR1)是一种跨膜蛋白,参与细胞内血红素的运输 [22]。一项研究表明,长非编码RNA FLVCR1-AS1通过分泌miR-573上调E2F转录因子3的表达,促进肺癌的增殖和侵袭 [23]。此外,一些研究表明,在乳腺癌中,LncRNA FLVCR1-AS1通过miR-381-3p/CTNNB1轴促进增殖、迁移和激活Wnt/β-catenin途径 [24]。此外,LncRNA FLVCR1-AS1介导miR-513/YAP1信号,以促进卵巢癌细胞的进展、迁移、侵袭和EMT过程 [25]。铁蛋白轻链(ferritin light chain, FTL)是铁代谢的关键蛋白,与癌症患者的生存密切相关。一些研究表明,FTL可能在前列腺癌、肺癌、肾癌和卵巢癌等多种癌症中发挥重要的生物标志物作用 [26] [27] [28]。HIF1A (缺氧诱导因子1亚单位α)是血管生成的重要组成部分,作为对肿瘤缺氧的反应而被激活,并促进肿瘤存活 [29]。先前的研究表明hsa-circ- 0000211通过调节miR-622/HIF1-A网络促进肺腺癌细胞的迁移和侵袭 [30]。HMOX1 (血红素加氧酶1)是一种催化反应的酶,可降解若干重要蛋白质(如血红蛋白、肌红蛋白和细胞色素p450)中所含的血红素基团 [31]。先前的研究表明HMOX1在乳腺癌中具有抗肿瘤作用 [32]。另一项研究报道HMOX1抑制小鼠乳腺癌中Notch1途径介导的肿瘤转移 [33]。此外,一项研究表明,HMOX1通过黑色素瘤中的B-Raf-ERK信号通路促进细胞增殖 [34]。
在我们的研究中,我们的基于铁代谢相关基因的肝癌患者预后模型得到了ICGC队列的验证。此外,我们还探讨了预后模式、临床病理参数与肿瘤浸润免疫细胞的关系。此外,利用人类蛋白质图谱Oncomine数据库对4个铁代谢相关基因的表达水平进行了检测。此外,CIBERSORT结果表明,预后模型中的低危患者可能对免疫治疗敏感。铁代谢是一个动态的、连续的过程。目前尚不清楚是否分析铁代谢相关基因的变化足以反映铁代谢活性。因此,我们的工作存在一些局限性。首先,铁代谢相关基因与肝癌的关系还没有进一步的实验研究。第二,缺乏大量的临床研究来证实我们的结果。
总之,我们的研究构建了一个基于铁代谢相关基因的肝癌患者预后模型。我们的预后模型在临床上显示出巨大的潜力。然而,这些铁代谢相关基因是否有助于免疫治疗靶点的选择和个体化治疗尚需进一步研究。
NOTES
*通讯作者。