1. 引言
乳腺癌是全球女性最常见的恶性肿瘤之一 [1] ,可根据雌激素受体(estrogen receptor, ER)、孕激素受体(progestogen receptor, PR)和人表皮生长因子受体2 (human epidermal growth factor receptor 2, HER-2)的表达水平分为不同亚型,表达ER、PR和HER-2的患者预后好,治疗上可选择干预激素产生的内分泌治疗药物及抑制HER-2的靶向药物等 [2] [3] [4] ;而ER、PR和HER-2均为阴性的三阴性乳腺癌(triple negative breast cancer, TNBC),对一些最有效的乳腺癌疗法不敏感,且恶性程度高,常发生于年轻女性,缺乏有效的靶向治疗药物 [5] [6] ,因此迫切需要寻找新的治疗靶点。近年来,肿瘤代谢逐渐成为研究的热点,如肿瘤糖代谢、线粒体代谢,谷氨酰胺、蛋氨酸代谢等,其中以有关肿瘤糖代谢的研究最为广泛 [7] [8] [9] 。正常细胞的葡萄糖代谢包括糖酵解和三羧酸循环,1分子葡萄糖在糖酵解的整个糖代谢过程中,仅净产生2分子ATP,正常细胞主要通过有氧呼吸获得能量,在有氧氧化中,1分子的葡萄糖净生成32或者38分子ATP,但肿瘤细胞与正常细胞不同,即使在有氧条件下,肿瘤细胞仍然进行高无氧糖酵解,因此抑制糖酵解具有抑制增殖和杀伤肿瘤细胞的作用 [10] [11] 。本研究拟通过生物信息学方法筛选乳腺癌糖酵解相关的高风险基因,为进一步研究乳腺癌预后标志物选择和治疗新靶点提供参考。
2. 材料与方法
2.1. 转录组数据及临床数据的下载
通过癌症基因组图谱(the cancer genome atlas, TCGA)数据库,检索收集乳腺癌肿瘤样本和正常样本的转录组数据及包含年龄、生存状态和肿瘤分期的临床数据,通过R4.0.3计算正常样本和肿瘤样本的数量,获取基因表达数据集文件及基因表型数据文件。
2.2. 基因富集分析
基因集富集分析(gene-set enrichment analysis, GSEA)数据库中搜索下载糖酵解相关的基因集,将基因表达数据集文件、基因表型数据文件及基因集文件导入GSEA软件,进行基因富集分析,得到基因富集显著的基因集。
2.3. 筛选乳腺癌糖酵解基因
提取糖酵解基因集表达量,按照P < 0.05,对筛选出的基因集在正常样品和肿瘤样品中进行差异分析,得到差异基因的表达量,其与TCGA数据库下载的临床数据合并,对基因表达数据和生存数据进行相关性分析,找到乳腺癌糖酵解相关的高风险基因。
2.4. 糖酵解预后模型构建
对筛选出的乳腺癌相关的糖酵解基因构建比例风险回归模型(proportional hazards model,简称COX模型),计算出每个临床样本的风险值,以风险值的中位值为界,将高于中位值和低于中位值的病人分别划分为高、低风险两组,采用Kaplan-Meier法计算生存率,并通过R语言Survival程序包绘制出高低风险组的生存曲线图。
2.5. 模型验证
根据风险值,利用R程序包SurvivalROC绘制ROC曲线图,以评估预后模型的性能。通过单因素和多因素分析评估高风险基因的预后。
2.6. GEPIA数据库单基因生存分析
利用基因表达谱数据动态分析(gene expression profiling interactive analysis, GEPIA)数据库,对筛选出的乳腺癌糖酵解高风险基因分别做单基因生存分析。
2.7. 高风险基因在三阴性乳腺癌中的预后分析
从基因表达综合数据库(gene expression omnibus, GEO)下载三阴性乳腺癌数据集GSE58812,对筛选出的5个高风险基因做预后分析
3. 结果
3.1. 转录组数据及临床数据的下载
从TCGA数据库下载乳腺癌正常样本和肿瘤样本转录组数据,下载到正常样本文件111个,肿瘤样本文件1053个。下载临床数据文件,获取每个临床样本患者的生存时间、生存状态(存活或死亡)等基本信息。
3.2. GSEA分析结果
利用GSEA数据库,检索到5个糖酵解相关的基因集,分别为 “BIOCARTA_GLYCOLYSIS_PATHWAY”、“HALLMARK_GLYCOLYSIS”、 “KEGG_GLYCOLYSIS_GLUCONEOGENESIS”、“REACTOME_GLYCOLYSIS”、 “REACTOME_REGULATION_OF_GLYCOLYSIS_BY_FRUCTOSE_2_6_BISPHOSPHATE_METABOLISM”,利用GSEA对这些基因集进行富集分析,筛选出P < 0.05的基因集2个“HALLMARK_GLYCOLYSIS”(q = 0.0085, P = 0.005)、“REACTOME_GLYCOLYSIS”(q = 0.0134, P = 0.007) (见图1)。图中横坐标是根据表达信息与表型的关联进行排序的基因,纵坐标是富集评分(Enrichment Score, ES),HALLMARK_GLYCOLYSIS、REACTOME_GLYCOLYSIS两个基因集下所有基因均在排序列表的顶部富集,说明该基因集在乳腺肿瘤中是上调趋势。
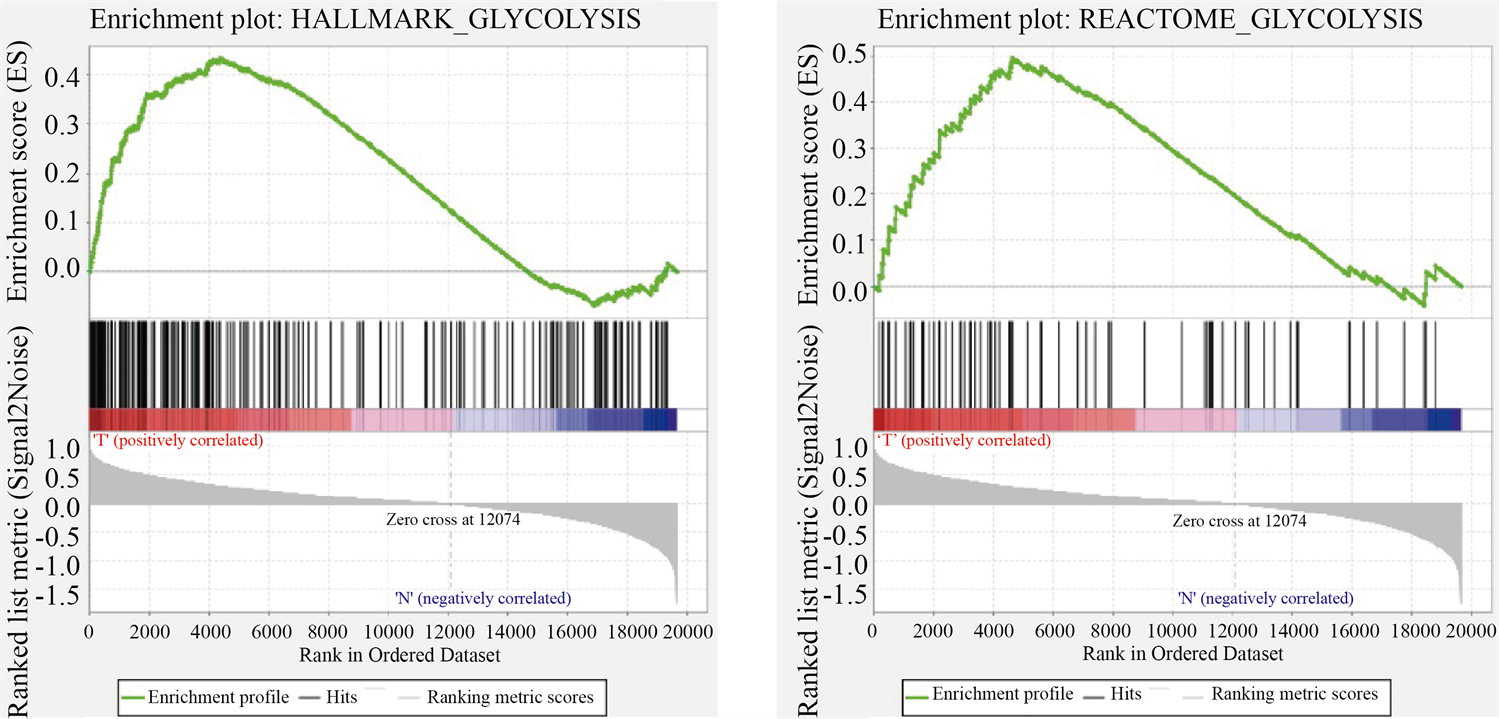
Figure 1. Enrichment of two gene sets with major variations between BC tissues and noncancerous tissues
图1. 乳腺肿瘤组织和正常组织之间2个主要富集差异的基因集
3.3. 糖酵解相关基因结果
对通过GSEA富集分析筛选出的2个基因集,在正常组织和肿瘤组织中进行差异分析,得到两者差异表达的糖酵解基因256个。
3.4. 糖酵解预后模型构建
将差异表达的糖酵解基因和生存数据合并,再得到乳腺肿瘤糖酵解相关基因预后模型,该模型纳入基因为PGK1、SDC1、NUP43、IL13RA1、CACNA1H (表1)。Kaplan-Meier生存分析结果(图2)显示,乳腺癌患者高风险组与低风险组生存差异显著(P < 0.05)。
3.5. 模型验证
3.5.1. ROC曲线图
根据风险值绘制5年ROC曲线(图3),曲线下方的面积被称为AUC (Area under Curve),AUC值越高,也就是曲线下方面积越大,说明模型预测病人生存准确率越高。本次利用生物信息学对乳腺癌转录组数据分析,得到的AUC = 0.72,预测准确率较高。
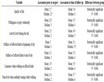
Table 1. High-risk genes associated with glycolysis in breast cancer
表1. 乳腺癌糖酵解相关高风险基因
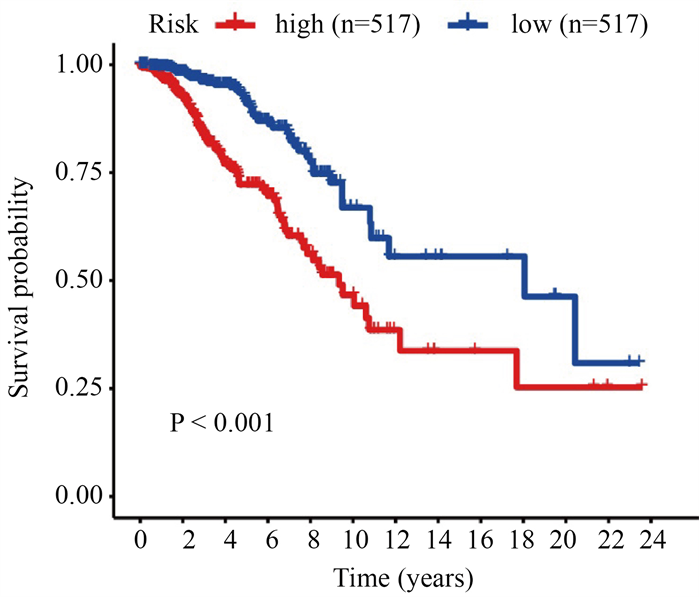
Figure 2. Survival analysis of high-risk and low-risk in breast cancer patients
图2. 乳腺癌患者高风险组与低风险组生存分析
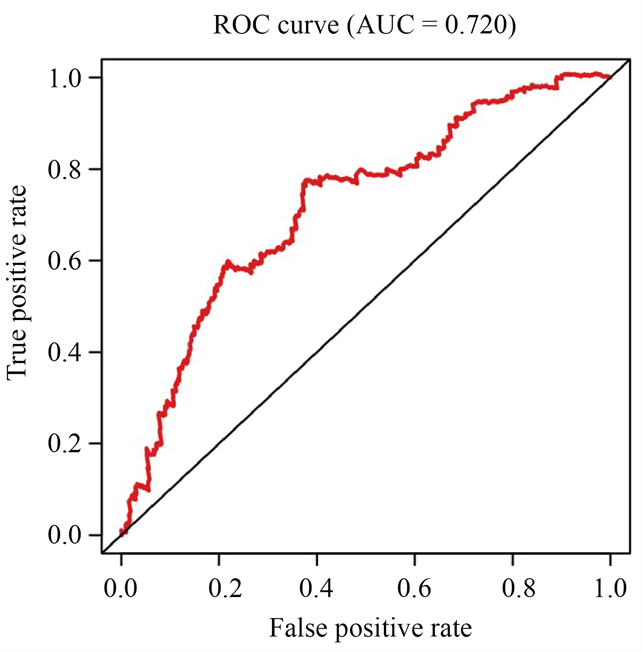
Figure 3. Time-dependence ROC curve according to the 5-year survival of the area under the AUC value
图3. 根据5年生存曲线下面积的时间依赖性ROC曲线
3.5.2. 独立预后分析
我们将年龄(>60岁),分期(T、M、N)和风险评分定义为独立的预测指标,通过单因素和多因素分析显示,这些变量与患者生存相关(P < 0.05),见图4。
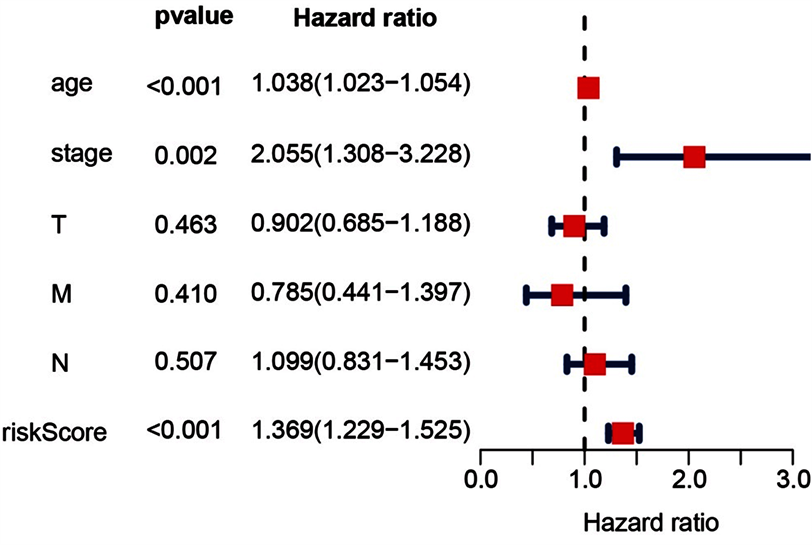
Figure 4. Forest plot of multivariate COX regression analysis
图4. 多因素COX回归分析的森林图
3.6. 单基因生存分析结果
采用GEPIA数据库对基因PGK1、SDC1、NUP43、IL13RA1、CACNA1H的表达水平对肿瘤患者总生存期(overall survival, OS)做的单基因生存曲线显示5个基因在乳腺癌中的表达水平均与病人预后呈负相关(图5)。
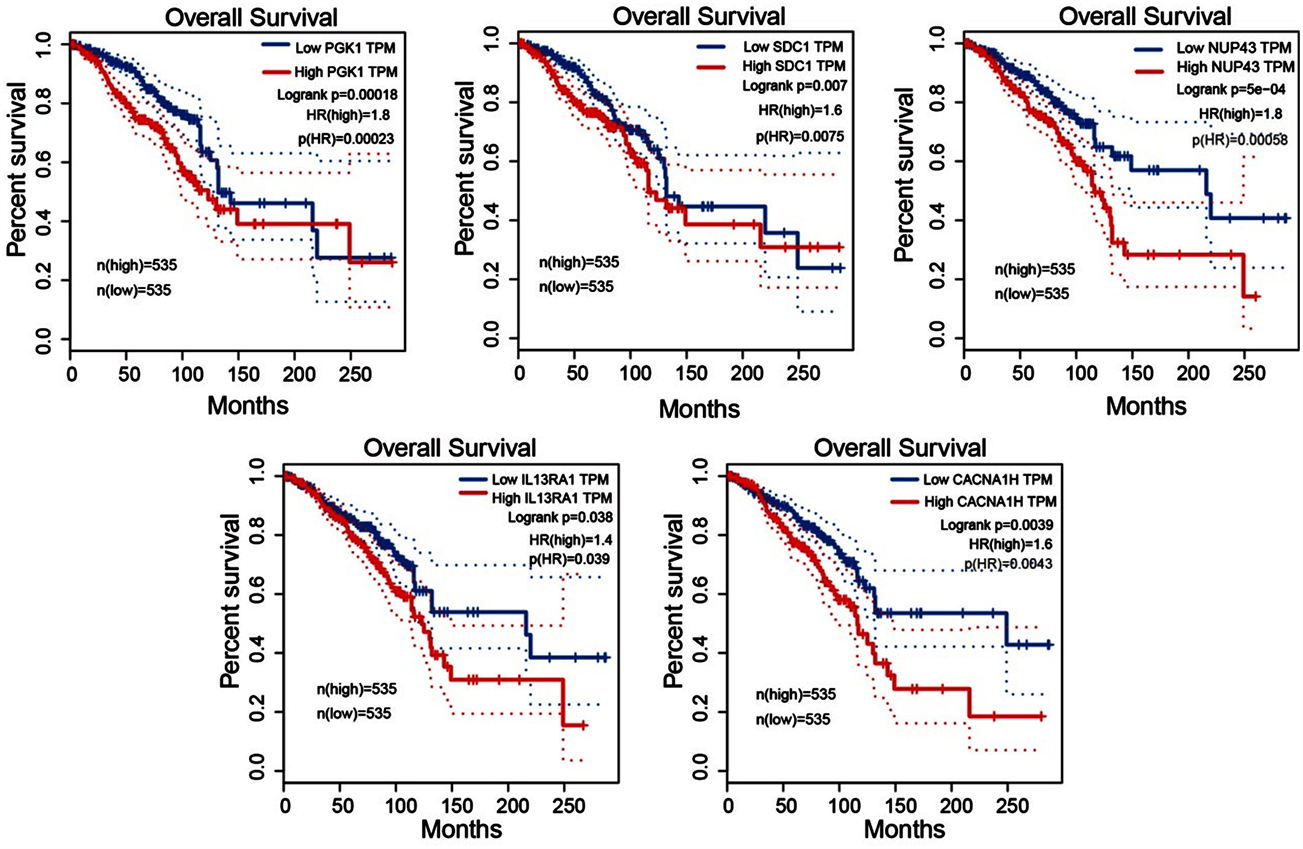
Figure 5. Single gene survival analysis
图5. 单基因生存曲线
3.7. 糖酵解高风险基因表达与TNBC患者预后的关系
根据PGK1、SDC1、NUP43、IL13RA1、CACNA1H基因表达情况,我们将GSE58812数据集分为高表达组和低表达组,结果显示(图6),PGK1、CACNA1H、SDC3高表达组预后较差,差异具有统计学意义(P < 0.05),且与TCGA-BC数据分析结果一致;而基因NUP43、IL13RA1的表达对三阴性乳腺癌患者的预后无显著影响(P > 0.05)。
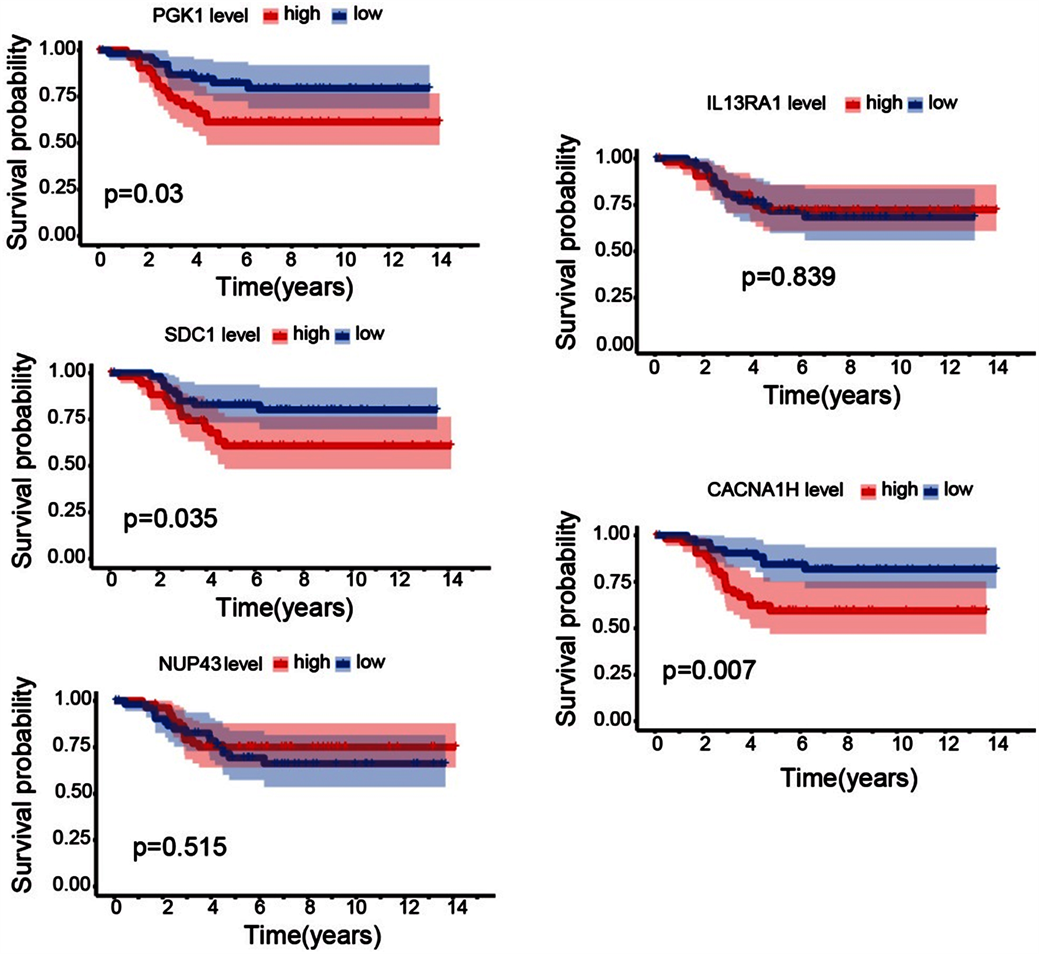
Figure 6. The correlation analysis of genes expression level and prognosis of triple negative breast cancer
图6. 基因表达水平与三阴性乳腺癌预后相关性分析
4. 讨论
20世纪20年代,德国诺贝尔奖获得者Warburg发现了肿瘤代谢特征,即肿瘤细胞即使在有氧条件下,依然依赖糖酵解供给能量而抑制线粒体能量代谢,这种现象称为“瓦博格效应” [12] 。在这一代谢特征的启发下,许多关于的研究取得了很多成果 [13] 。有关研究发现,体外乳腺癌模型中存在糖酵解应激反应,在细胞分裂和死亡过程中,p53基因参与其中,而它是通过癌细胞中的“有氧糖酵解”这一步骤来进行调节的,有氧糖酵解在其生长过程中发挥了非常重要的作用 [14] 。为进一步了解乳腺肿瘤中糖酵解相关基因表达,我们利用TCGA数据库对111个正常样本和1053个乳腺肿瘤样本进行了糖酵解相关基因的差异分析,发现GSEA富集分析中的两个基因集HALLMARK_GLYCOLYSIS、REACTOME_GLYCOLYSIS在乳腺肿瘤中呈上调趋势,说明在肿瘤组织中糖酵解活跃,符合“瓦博格效应”。ROC曲线显示,用此糖酵解相关基因模型预测病人生存率准确性较高。进一步将筛选出的基因在GEPIA数据库做单基因预后分析,结果显示这5个基因表达与患者预后呈负相关,有统计学意义。说明PGK1、SDC1、NUP43、IL13RA1、CACNA1H为乳腺肿瘤高风险基因。最后我们用分析了GEO数据库中三阴性乳腺癌患者的上述5个基因的表达和预后的相关性,进一步证实PGK1、SDC1、IL13RA1基因表达与TNBC患者预后成负相关。
磷酸甘油酸激酶(PGK)是糖酵解过程中催化ATP形成的主要酶,它的两种亚型PGK1和PGK2在人类中具有相似的结构和功能 [15] [16] 。PGK1和丙酮酸激酶M2 (PKM2)是癌细胞有氧糖酵解过程中控制ATP产生的两种酶 [17] [18] ,缺氧条件下PGK1介导糖酵解为肿瘤细胞产生ATP也与各种肿瘤的发生发展显著相关 [19] 。因此,PGK1是一个非常有潜在应用价值的肿瘤治疗靶点。Syndecan1 (CD138)是SDC1基因编码的Syndecan家族四个成员中的第一个成员 [20] 。它由三个结构域组成,其中胞外结构域在细胞和基质的相互作用中起着关键作用,并作为趋化因子和生长因子的供受体,参与细胞增殖、迁移等功能 [21] 。多数研究认为SDC1在乳腺癌中高表达与乳腺癌的侵袭性和不良预后相关,抑制SDC1表达或功能可以治疗乳腺癌或延缓其进展 [22] [23] [24] [25] ;这些研究结果均与本文中我们通过生物信息学方法得到的结果是一致的。CACNA1H基因编码T型电压依赖钙通道Cav3.2孔洞的α1亚基 [26] ,Viet等通过生物信息学分析发现口腔鳞癌患者的CACNA1H甲基化水平与患者5年生存率呈负相关 [27] ,说明CACNA1H改变可能与肿瘤发展及预后相关,但其在乳腺肿瘤中的生物学作用未见研究报道。
Nup43蛋白由NUP43基因编码,是Nup106-107复合体的稳定成分,位于有丝分裂的着丝点,调控有丝分裂进程和染色体分离 [28] 。IL-13Rα1和IL-13Rα2是白介素13 (IL-13)的两个结合受体链 [29] ,IL-13Rα2是研究广泛的细胞表面靶点之一,多项研究表明其在肿瘤组织中的表达水平高于正常组织,且与肿瘤不良预后相关 [30] [31] [32] 。但在本研究分析中发现NUP43和IL13RA1与乳腺肿瘤预后相关,与三阴性乳腺癌患者预后相关性不显著。考虑乳腺肿瘤具有异质性,NUP43和IL13RA1基因表达可能与非三阴性乳腺癌患者预后相关性更大。
本研究通过一系列生物信息学方法,得到与乳腺癌糖酵解相关的包含5个高风险基因的预后模型,并通过ROC曲线和单因素及多因素分析对模型进行评估,其中5个乳腺肿瘤糖酵解相关高风险基因分别为PGK1、SDC1、NUP43、IL13RA1、CACNA1H。目前有文献报道PGK1、SDC1基因与乳腺癌的预后呈负相关 [33] [34] ,我们也做了单基因的生存分析,与其报道一致,说明我们研究方法的可信性。并且本研究进一步分析得出这些高风险基因中的PGK1、SDC1、CACNA1H的表达同样影响三阴性乳腺癌患者预后。因此,PGK1、SDC1、CACNA1H或可作为乳腺癌特别是三阴性乳腺癌治疗药物开发的重要潜在靶点,或一种新的预后生物标志物,均有较大深入研究价值和应用前景。
NOTES
*通讯作者Email: qdjingl@163.com