1. 引言
轴承是一种精密的机械元件,也是各种机械设备中举足轻重的基础件。滚动轴承作为机械设备中应用最广泛的标准部件之一,其本身精度高,对使用环境要求也相对较高,而且也是最易损坏并导致事故发生的部件[1] 。轴承监测是保证产品尤其是高精度产品质量既能符合国家标准和企业内控标准,又可以很好地满足用户需求的手段,不但重要而且非常必要[2] 。据统计,在使用滚动轴承的旋转机械设备中,约有30%的机械故障是与滚动轴承损伤有关的[3] [4] 。所以,对滚动轴承的状态监测和故障诊断迫在眉睫。轴承的故障诊断可采用温度诊断技术、油样分析技术、油膜电阻诊断技术、声发射诊断技术和振动诊断技术等,而从适用、实用、有效的观点看,振动诊断法是目前最有效的滚动轴承状态监测与故障诊断方法[5] -[8] 。本文基于滚动轴承的振动信号数据,通过建立识别神经网络对轴承的运行状态进行监测及故障诊断。
2. 实验系统及信号
一个完整的滚动轴承故障诊断系统应该包括数据采集和故障诊断两部分。其中数据采集部分用于采集和存储滚动轴承的振动信号;而故障诊断部分用于连续或者间断地处理振动信号,实现故障诊断,并将诊断结果显示出来。在本实验中,主要采用了压电式加速度传感器来测量振动信号,将传感器安装在合适的测点位置;由于传感器测得的振动信号比较微弱,因此传感器测得的信号需接入电荷放大器进行放大;放大器输出的信号是模拟信号,计算机无法识别,因此振动信号还需A/D卡进行模数转换,得到计算机可以识别和处理的数字信号,本文所涉及实验中采用的是PCI-1711研华数据采集卡。
3. 振动信号数据的特征提取
在设计好滚动轴承状态的监测装置后,基于所采用的装置采集滚动轴承的振动信号,再利用MATLAB对采集到的信号进行数据处理和分析。首先通过零均值化进行数据预处理,结果如图1所示。
3.1. 滚动轴承时域特征值提取
在时域内分别抽取如下七种特征值:均方根值、峰值、峰值因子、峭度因子、脉冲因子、裕度系数和波形因子。各特征参数的计算公式及物理意义如下:
1) 均方根值:其与轴承表面波纹等缺陷引起的无规则振动波形的异常有较好的相关性;
(1)
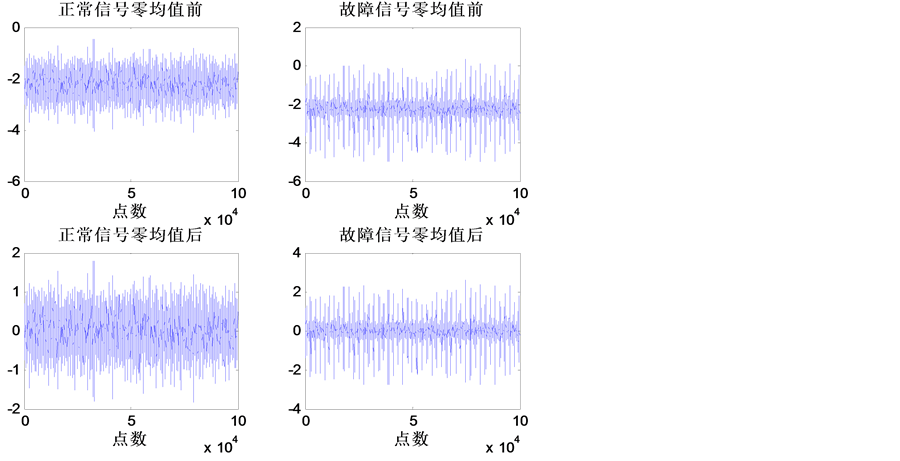
Figure 1. Normal and fault bearing signals zero mean contrast figure
图1. 正常信号和故障信号零均值前后对比图
2) 峰值:峰值反映了信号偏离平均值的程度;
(2)
3) 峰值因子:适用于点蚀类故障的诊断;
(3)
4) 峭度系数:表示轴承振动信号振幅的规则性,当过大时则意味着有故障出现;
(4)
5) 脉冲因子:反映了振动脉冲的频率;
(5)
6) 裕度系数:反映信号冲击程度的一个指标,对轴承的冲击故障较为敏感;
(6)
7) 波形因子:可用来判定滚动轴承的损伤类型。
(7)
动力设备在各种负荷状态下的运行状态叫做工况。对于滚动轴承,在相同的负荷状态下,不同的转速也属于不同的工况,所以在对信号的处理和分析中,应在相同的转速下,提取特征值。在本实验中,正常轴承和故障轴承在zs = 20 rpm, zs = 25 rpm, zs = 30 rpm三种工况下采集振动信号,每种工况下又分成3组数据,得2*3*3 = 18组数据。以滚动轴承转速为20 rpm(20转/每分钟)时的状态数据为例说明,时域特征结果见表1。
正常轴承和故障轴承zs = 20时分别提取3组特征,根据表1得到时域特征值曲线对比图如图2,由图可知在相同的工况下,时域特征值的重复性好,不同工况下,峰值、峭度因子、峰值因子、脉冲因子和裕度因子特征值在幅值上差别很大,尤其是峭度因子和裕度因子在正常状态下和故障状态下区别非常明显。
3.2. 滚动轴承频域特征值提取
对信号进行时域分析时,有时一些信号的时域参数相同,但并不能说明信号就完全相同。因为信号不仅随时间变化,还与频率、相位等信息有关,这就需要进一步分析信号的频率结构,并在频域中对信号进行描述。动态信号从时间域变换到频域主要通过傅立叶级数和傅立叶变换实现,其中周期信号靠傅立叶级数,非周期信号靠傅立叶变换。
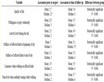
Table 1. Rolling bearing vibration signal time domain features
表1. 滚动轴承振动信号时域特征值
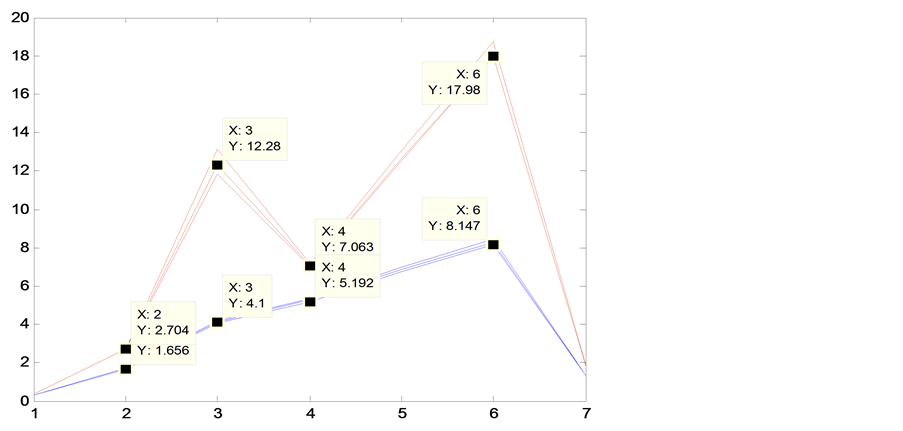
Figure 2. Normal and fault bearing signals time domain features contrast figure (zs = 20 rpm)
图2. zs = 20时正常信号和故障信号的时域特征值对比图(红色——故障信号、蓝色——正常信号)
在本实验中,利用傅里叶变换提取频域特征。傅里叶变换(FFT)是一种频域分析方法,当信号被分解成各次谐波以后,我们就可以从频域来分析处理问题。信号的频域特性是信号的内在本质,而信号的时域波形只是信号的外在形式。显然,从本质上分析处理问题将会更深入,得到的分析结果也将会更全面、更具有优越性。
在频域中,首先提取如下三种特种值:重心频率(zxpl),频率方差(plfc),均方频率(jfpl)。
根据表2得正常轴承和故障轴承振动信号频域特征对比图如图3,从图中可以明显看出故障轴承信号的频域特征校时域信号相反,在幅值上均小于正常轴承信号的特征值。
由图2、图3可以得出:当转速为20 rpm时,正常轴承信号和故障轴承信号所提取的时、频域特征值对比非常明显。为了验证选取的特征值是否具有普适性,分别在转速为20 rpm、25 rpm和30 rpm状态下采集信号,并分别求取这三种状态下信号的频率特征,其对比结果如图4所示,正常轴承在不同的转速下频域特征值有很好的重复性,故障轴承在不同的转速下频率特征值表现出幅值差异很大,无规律。
在不同的工况下(zs = 20、zs = 25和zs = 30),所得到的滚动轴承信号频域特征是不同的,虽然在zs = 20时故障轴承频域幅值的差异非常明显。当zs = 25和zs = 30时,情况发生了改变,不仅幅值差异很大,而且变化趋势也有所不同。说明了不同的工况,提取的频域特征不具有重复性和变化趋势一致性,根据
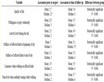
Table 2. Rolling bearing vibration signal frequency domain features
表2. 滚动轴承振动信号频域特征值一
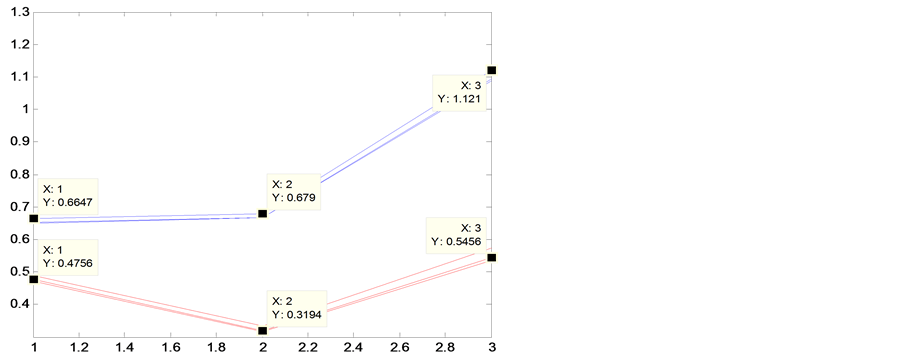
Figure 3. Normal and fault bearing signals frequency domain features contrast figure (zs = 20 rpm)
图3. zs = 20时正常信号和故障信号的频域特征对比图(红色——故障信号、蓝色——正常信号)
特征值选取原则,重心频率、频率方差、均方频率都不可以作为模式识别的特征量,重新选取频域特征。
为了得到的特征值在相同工况下有很好的重复性且变化趋势具有一致性,不同工况下又有很好的差异性,本实验中,对三种工况采集到的数据分别分段提取FFT谱的分段最大值均值特征(将采集信号的FFT谱按能量特征分成9段),得到的特征值如表3所示。
正常状态和故障状态,分别各提取zs = 20, zs = 25, zs = 30转速下的一组特征,根据表3中分段FFT频谱最大值均值得到的正常状态和故障状态特征值曲线对比图如图5,从图中可以看到在不同转速下信号有较好的重复性,而且正常信号和故障信号之间的D3、D7、D8又具有比较明显的差异性。
由图5可知,相同工况下选取的九个特征值中,根据有效特征值的选取原则:1) 同状态信号重复性好;2) 不同状态信号差异性好,处于横坐标为3、7、8上的特征值可以选作模式识别的特征值。
对时域和频域特征值的比较筛选,得到八组特征值用于模式识别的特征值,整理数据如表4。
4. 模式识别
4.1. 特征值归一化
当所有样本的输入信号都为正值时,与第一隐含层神经元相连的权值只能同时增加或减小,从而导致学习速度很慢。为了避免出现这种情况,加快网络学习速度,需要对输入信号进行归一化,使得所有
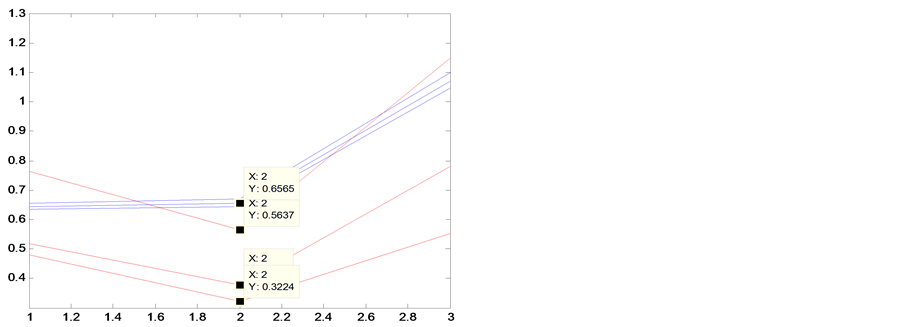
Figure 4. Normal and fault bearing signals frequency domain features contrast figure at three different speeds
图4. 三种不同转速下正常信号和故障信号的频域特征对比图(红色——故障信号、蓝色——正常信号)
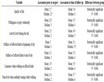
Table 3. Rolling bearing vibration signal frequency domain features
表3. 滚动轴承信号频域特征值二
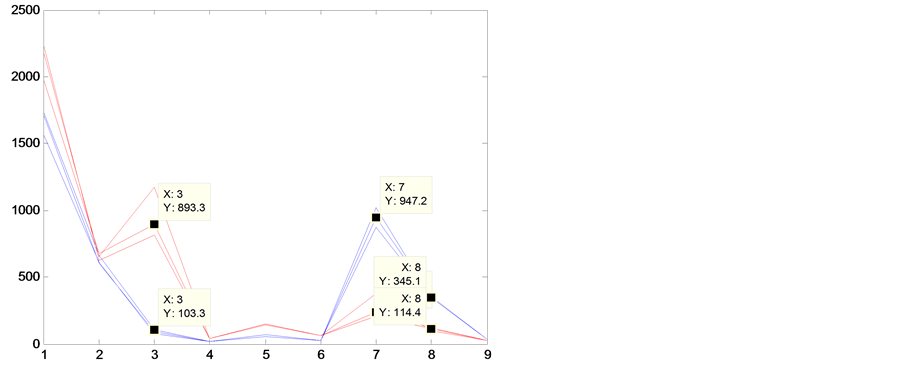
Figure 5. Normal and fault bearing signals frequency domain features contrast figure (zs = 20 rpm)
图5. zs = 20时正常信号和故障信号的频域特征对比图(红色——故障信号、蓝色——正常信号)
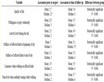
Table 4. Rolling bearing vibration signal features
表4. 信号有效特征值对比
样本的输入信号其均值接近于0或与其均方差相比很小。归一化就是要把数据通过某种算法处理后,限制在一定范围内。首先归一化是为了后面数据处理的方便,其次是保正程序运行时收敛加快。归一化的具体作用是归纳统一样本的统计分布性。
神经网络是以样本在事件中的统计分布几率来进行训练(概率计算)和预测的,归一化是统一在(0, 1)之间的统计概率分布,所以在本实验中,对提取的轴承信号特征值采用线性函数转换,其表达式为:
,其中x、y分别为转换前、后的值,MaxValue、MinValue分别为样本的最大值和最小值。归一化后的特征值数据如表5所示。
4.2. BP神经网络
BP(Back Propagation)神经网络,通过误差反向传播来不断调整网络的权值和阈值的学习过程,由信息的正向传播和误差的反向传播两个过程组成。输入层各神经元负责接收来自外界的输入信息,并传递给中间层各神经元;中间层是内部信息处理层,负责信息变换,根据信息变换能力的需求,中间层可以设计为单隐层或者多隐层结构;最后一个隐层传递到输出层各神经元的信息,经进一步处理后,完成一次学习的正向传播处理过程,由输出层向外界输出信息处理结果。当实际输出与期望输出不符时,进入误差的反向传播阶段。误差通过输出层,按误差梯度下降的方式修正各层权值,向隐层、输入层逐层反
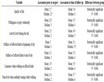
Table 5. Rolling bearing vibration signal normalized features
表5. 归一化后的特征值
传。周而复始的信息正向传播和误差反向传播过程,是各层权值不断调整的过程,也是神经网络学习训练的过程,此过程一直进行到网络输出的误差减少到可以接受的程度,或者预先设定的学习次数为止。
4.3. BP神经网络的建立
BP网络是由一个输入层,一个或多个隐层以及一个输出层组成,上下层之间实现全连接,而每层神经元之间无连接,输入的神经元可以根据需要求解的问题和数据表示方式确定。在故障诊断中,输入层神经元个数一般等于每个样本中包含的特征值的个数,输出层的维数可根据设计者的要求确定。在正向传播进程中,输入信息从输入层经隐层加权处理传向输出层,经功能函数运算后得到的输出值与期望值进行比较,若有误差,则误差反向传播,沿原先的连接通道返回,通过逐层修改各层的权重系数,减小误差。随着这种误差逆向传播修正的不断进行,网络对输入模式响应的正确率也不断上升。
其中隐层的设计:隐层的神经元个数选择是一个较为复杂的问题,往往需要设计者多次试验来确定,因而不存在一个理想的解析式来表示。隐层单元的个数与问题的要求、输入/输出单元的数目都有着直接关系。隐层单元个数太多会导致学习时间过长、误差不一定最佳,也会导致容错性差、不能识别以前没有见到的样本等问题,因此一定存在一个最佳的隐层单元数。在本实验中,主要参照以下三个公式确定最佳隐层单元数:
(8)
其中
是输入层神经元数,
是隐层神经元数;
(9)
其中,m是输出神经元数,n是输入神经元数,a是[l, 10]之间的常数;
(10)
其中n是输入神经元数。
三层BP神经网络的网络结构图如图6。
4.4. 神经网络训练及结果
神经网络训练程序如下:
net=newff(minmax(x),[21,2],{'tansig','logsig'},'traingdx');
net.trainParam.epochs = 300;
net.trainParam.lr = 0.1;
net.trainParam.goal = 0.0001;
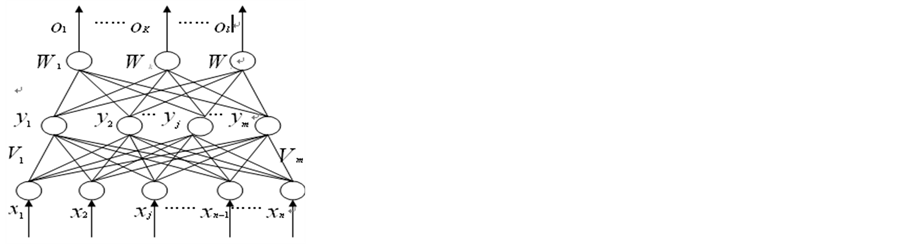
Figure 6. BP network structure of three layers
图6. 三层BP神经网络的网络结构示意图
net=train(net,x,t);
y=sim(net,x);
error=y-t res(i)=norm(error);
程序训练得到结果为:
error =
−0.0166 −0.0186 −0.0157 0.0069 0.0118 0.0076 0.0031 0.0030 0.0034 −0.0010 −0.0026 −0.0011
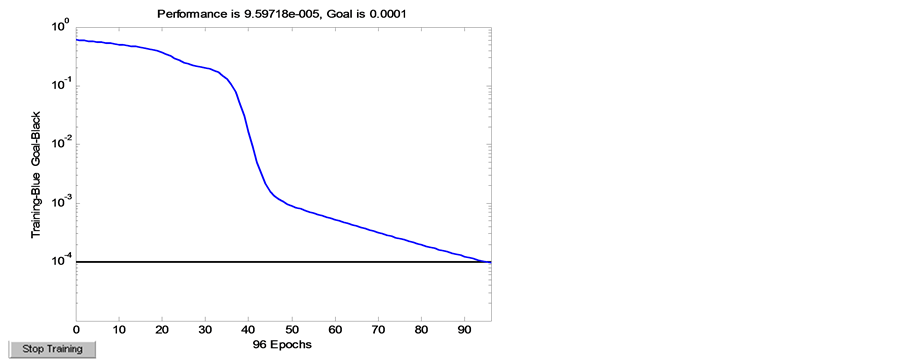
可见网络训练收敛速度快,稳定性好。使用验证样本对已经训练好的网路进行仿真,比较仿真结果与目标结果,并通过比较计算训练样本、验证样本平均误差评价此BP神经网路的有效性。而通过识别结果得知,所建立的BP网络识别结果准确,达到了预期的目标。
5. 总结
从对滚动轴承故障监测的实际出发,以及滚动轴承状态监测的重要性和发展前景,设计选择监测装置,并通过选用的监测装置采集正常轴承和故障轴承的诊断信号数据,对获得的数据提取时域特征和频域特征,通过正常信号和故障信号的特征值对比图,根据特征值的选取原则,提取有效特征值,如果不加选择地选取过多故障特征作为神经网络的输入,不仅不能提高系统正确诊断率,反而会降低诊断率。以神经网络理论为基础,结合滚动轴承故障诊断的特点,设计出适合于滚动轴承故障诊断的BP神经网络,将时域特征和频域特征进行不同组合作为BP神经网络的输入,对BP神经网络进行训练及验证,运用训练网络对未知轴承状态进行监测,实验证明该方法能够对轴承运行状态进行有效的实时检测和诊断。