1. 引言
短期负荷预测一般是预测未来1小时至1周的负荷,对电力系统的运行和生产有着重要的意义。目前,用于短期负荷预测的方法很多,总体分为传统和现代智能两类方法。传统的短期负荷预测方法有回归分析法、时间序列法等。实践表明,传统方法在应用中存在局限性,如负荷预测的输入和输出间呈非线性关系,就很难找到理想的预测模型[1] 。因此,现代智能短期负荷预测方法应运而生,如神经网络法、 小波分析法、支持向量机、灰色系统预测法等。
电力负荷具有不稳定性、非线性,且表现为以天、周、年为周期发生波动,大周期中套有小周期这些特殊的周期性。小波变换能将其呈周期性变化的子负荷序列分离出来,这样更有利于具有周期性的短期负荷预测精度的提高。小波分析在电力系统短期负荷预测中已得到广泛应用。
GBR算法是Friedman提出的将Boosting算法扩展以解决回归问题的预测算法,在多个领域有着广泛应用,如生物研究、搜索排名和机器学习等。它对数据具有很强的鲁棒性,能够很好地处理不干净的、含噪声的数据,支持不同的损失函数,并对非线性数据有很强的预测能力。国内外研究人员将GBR算法应用于太阳能领域[2] 的预测、汽车保险损失成本预测[3] 、碳钢的土壤腐蚀规律研究[4] 等。但在电力系统负荷预测领域应用还很少。
本文将小波变换与梯度Boosting回归相结合进行短期负荷预测。利用小波变换将负荷序列及气象序列(温度、湿度、气压、风速)分别分解到不同的频段上,选取输入特征向量对每个负荷分量建立基于相应气象因素影响的WGBR模型进行预测,最后综合得到负荷序列的最终预测结果。实例证明应用本文方法对电力系统短期负荷进行预测取得了较高的预测精度。
2. GBR算法
Boosting算法是基于统计学习理论的一种重要的机器学习方法,近年来在模式识别,回归分析,特征提取等方面得到了广泛的应用[5] ,其中由Freund和Schapire提出的AdaBoost (Adaptive Boost)算法分类在理论分析和实际应用中取得了巨大的成功[6] 。因此一些研究者试图将分类方法引入到回归问题中。Friedman将梯度下降方法应用于回归算法中,提出了Gradient Boosting算法[7] ,该算法利用沿着模型的损失函数的负梯度求解损失函数的最小值,迭代得到最佳模型。
GBR本质上是一种利用
个基函数的加法展开式对目标函数进行逼近的方法.表达式[3] 如下所示:
(1)
其中
为一个简单的参数函数,称为基函数,本文中它是决策树。
为它的参数,表示节点分枝变量、分枝规则、根节点的数目等[8] 。
为展开式系数,
为基函数的个数,
为输入向量,
为输出。
与其他的Boosting算法类似,为了得到最终的模型,GBR建立了分步向前可加模型进行迭代,即
(2)
也就是说,我们要找到使所有样本的损失函数达到最小值时的
即
(3)
其中
为第
个样本,
为样本数,本文选定的损失函数都为平方损失函数,即
(4)
算法伪代码步骤[3] [9] 如下:
给定初始模型
(5)
2) for
to
do
3) 计算负梯度
(6)
4) 通过输入向量
及负梯度
拟合一个回归树模型
,即可得到
5) 通过最小化
(7)
求得
6) 更新模型
(8)
其中
为学习率
7) end for
3. WGBR预测模型
3.1. 预测模型
本文数据来自华北地区某市2004年7月5日至8月22日整点时刻的负荷数据及相应的气象数据。将7月5日至8月15日负荷及气象序列作为训练数据,来预测未来一周(8月16日至22日)各时的电力负荷值。应用WGBR方法的电力系统短期负荷预测的具体过程图如图1所示。
流程图第一步为利用小波进行数据处理:本文将原始负荷序列
及气象序列(温度(
),湿度(
),气压(
),风速(
))都选用具有时频紧支撑和高正则性的Daubechies小波分别进行分解。根据实验经验,采用Daubechies5作为母小波,对负荷序列及气象序列用小波变换和Mallat算法进行3层分解。
和
分别称为负荷序列在分辨率
下的近似序列和细节序列。各层细节序列和近似序列是原
始序列在相邻的不同频率段上的成分。
由于用Mallat算法把序列分解,分解后的序列长度减半,这样各小波系数的序列长度必然不同,故第二步将负荷分解后的序列进行重构增加序列的长度。即对
和
分别进行重构[10] ,得到
和
。它们和原始序列
的长度一样,且有
(9)
同理可得到气象序列各自的重构序列:温度重构序列
,
,
,
,湿度重构序列
,
,
,
,气压重构序列
,
,
,
,风速重构序列
,
,
,
。
第三步为特征提取,此步骤为预测序列构造特征以便GBR建模预测。通过对实时气象序列与负荷序列之间的相关性分析,选取实时温度、实时湿度、实时气压、实时风速作为GBR模型的气象因素输入量。负荷序列本身又具有很强的自相关性及周期性。故选取的样本输入量如表1所示。标号(0),(-
),(0)-
,(-1)-
分别表示预测时刻,预测时刻前
个时刻,预测前
天预测点的同一时刻,预测前
天预测点的上一时刻。即表1所示的特征向量输入由预测时刻前11个时刻的负荷值,前11天每天同一时刻的负荷值,前9天预测时刻的上一时刻的负荷值,预测时刻前5个时刻的温度值,预测时刻前5个时刻的湿度值,预测时刻前2个时刻的气压值及预测时刻前2个时刻的风速值组成。
第四步利用GBR预测重构的负荷序列
,
,
,
,对应的特征见第三步。我们的历史数据实际上包括49天的负荷数据及相应的气象数据,我们把前42天的负荷及气象数据作为GBR模型的训练数据集,后7天的负荷及气象数据作为测试预测数据集。利用训练集建立GBR模型,将此模型应用到测试集进行预测,得到各个预测负荷序列,最后综合得到负荷序列的最终预测结果。
3.2. GBR的实现环境及参数说明
在利用Mallat算法对数据进行小波分解后,在Spyder环境下使用Python的scikit-learn包来实现GBR算法[2] 。

Figure 1. Short-term load forecasting model
图1. 短期电力负荷预测流程图
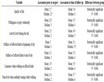
Table 1. List of input features for different series
表1. 各序列预测值选取的输入量
需要设置的参数有:基函数的个数(“n_estimators”),每棵树的深度(“max_depth”),学习率(“learning_rate”),损失函数(“loss”)等。树的最大深深度控制特征相互作用的程度。基函数越多,树越深,得到的方差就越大,模型复杂度就越高。学习率是最优正则化过程中的参数,最优正则化可以简化模型,使预测结果更稳定。学习率是位于(0,1]的超参数,它是衡量梯度下降过程的步长。经验表明,小的学习率有利于得到更好的测试误差。推荐设置学习率为一个小的常数(如学习率
) [11] 。scikit-learn包通过及早停止树的增长来避免决策树学习中的过度拟合问题,比如发现树的深度过高,叶节点过多,或者节点内数据过少。本文利用网格搜索法 [12] 解决参数设定问题,再进行适当的调整,找到最佳参数值。网格搜索法的优点是它能同时搜索多个参数值,最终获取的是使训练误差达到最小时的最优参数组合。同时计算过程中各组参数相互解耦,便于并行计算,提高运行效率。损失函数用来衡量当前模型的拟合程度,不同的损失函数将得到的不同的模型及结果。各预测序列所选则的参数值如表2所示。
4. 实例结果分析
为验证本文方法的有效性,笔者将本文方法与单独使用GBR预测方法进行比较,如图2,图3,图4,表3,表4所示。选取的误差如下:
绝对百分误差:
(10)
平均绝对百分误差:
(11)
式中:
为预测时刻数;
为
时刻的实际负荷值;
为
时刻的预测负荷。
图2是一周的WGBR预测值、GBR预测值及真实值的比较,可以看到WGBR方法的预测值更接近真实值,预测效果比GBR的预测效果更好。图3是两种方法各时预测值的绝对百分误差的对比,明显看出WGBR预测误差较小。表3是一周每天的平均绝对百分误差统计,可以看出WGBR误差基本小于
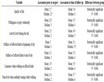
Table 2. Parameter values of WGBR and GBR models
表2. WGBR及GBR模型参数选择值
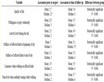
Table 3. Statistics of average relative error for a week
表3. 一周的平均绝对百分误差统计
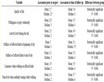
Table 4. Load forecasting result on the Nineteenth day by the WGBR and the GBR methods
表4. 19日各时WGBR和GBR方法对负荷预测的结果
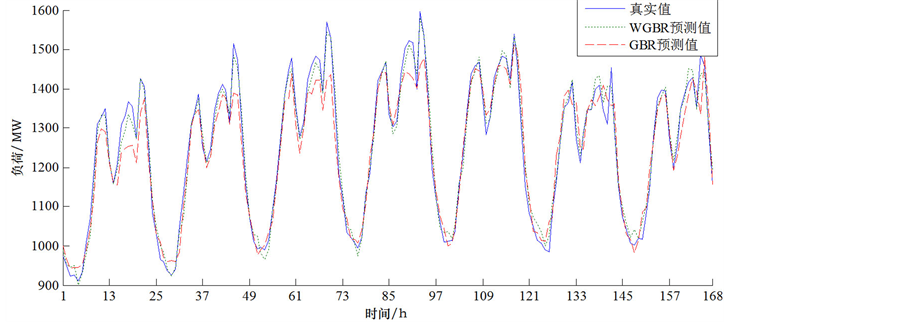
Figure 2. Actual load and forecasting load curve of a week
图2. 一周的负荷真实曲线与预测曲线
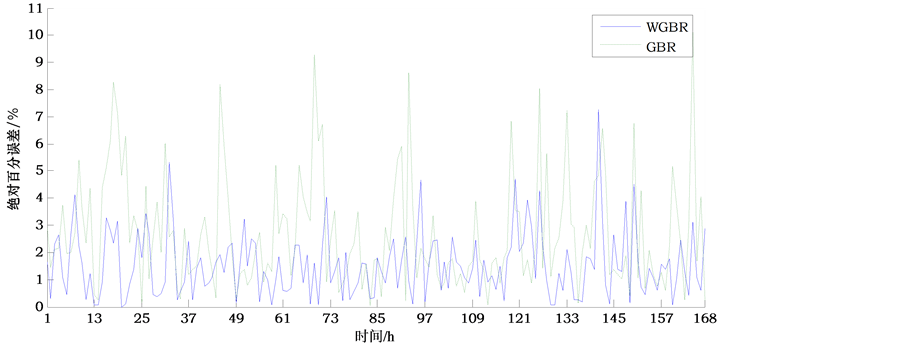
Figure 3. Comparison of forecasting load errors for a week
图3. 一周的负荷预测误差比较
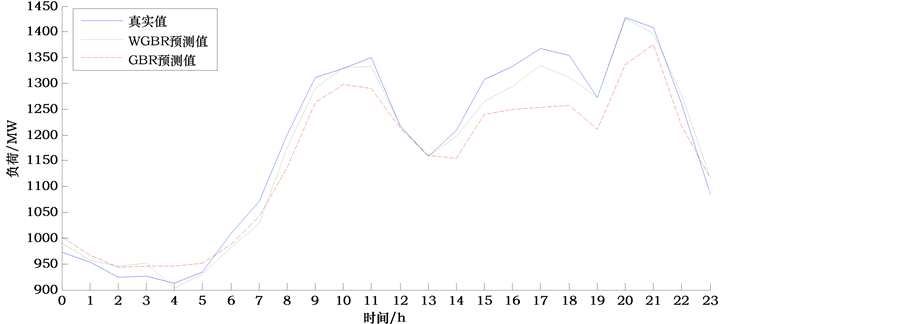
Figure 4. Actual load and forecasted load curve of a Monday
图4. 周一各时的负荷真实曲线与预测曲线
1.5%,取得了较好的预测精度。表4是8月19日(周四)各时的预测值与真实负荷值。图4是周一各时的预测值与真实值的曲线图,能够较清晰地看出WGBR的预测效果。
5. 结论
本文提出了一种基于小波变换的梯度Boosting回归预测电力系统短期负荷的方法,对不同频段的小波分量采用不同参数模型的WGBR方法进行预测更具针对性。通过对比GBR方法的预测效果,结果表明,本文的预测得到了更好的预测精度,其误差基本小于1.5%。小波分析强劲的数据处理能力及GBR算法高效的预测能力显而易见。
基金项目
本文工作由国家自然科学基金(11401208)项目资助完成。