1. 引言
改革开放以来,我国的批发和零售业得到了较快发展,促使社会消费品零售总额持续较快增长。我国批发和零售业无论从繁荣发展的程度看,还是从对国民经济的贡献率看,都是我国国民经济十分重要的行业,对拉动和扩大我国内需,促进我国经济快速平稳发展起到了越来越大的作用 [1] - [5] 。国外大型批发和零售业全面进入我国,对我国本土批发和零售业带来了强大冲击和市场压力,不仅使我国批发和零售业的国际化程度越来越高,还使我国境内的批发和零售业的市场竞争变得越来越激烈,批发和零售业的演变和发展趋势越来越复杂 [6] [7] 。面对国际上批发和零售业在我国零售市场上的规模化扩张,我国的批发和零售行业能否在“同台竞争”中不断发展壮大自己,还有我国零售和批发业未来的发展趋势,都是我们长久以来十分重视的问题。
本文以山东省批发和零售业为研究对象,以此来研究山东省批发和零售业未来的发展趋势。批发和零售业销售总额数据序列受到许多因素的影响,这些因素之间存在着很多复杂的联系,往往会表现出某种随机性,但是它们彼此之间也存在着统计上的依赖关系。本文把山东省批发和零售业的销售总额作为一个时间序列,根据时间序列的性质 [8] ,再以以前的资料为依托得到它的变化规律,以此来预测批发和零售业未来发展的趋势。为了准确的预测山东省批发和零售业的发展趋势,要求所建模型必须符合实际情况,并且满足统计理论的要求,这就需反复使用自回归单程移动平均模型ARIMA(p,d,q)调整模型 [9] - [12] ,选择出最适合山东省批发和零售业销售总额预测的模型,并应用此模型为山东省批发与零售业销售总额进行预测,预测结果可以为相关政府部门就此行业发展计划的制定提供一定的依据和参考价值。
2. 山东省批发和零售业销售总额模型方法
2.1. 数据查询
首先从山东省统计局网站查询数据,得到山东省从1979年到2014年的批发和零售业的销售总额数值(用
表示)。随着时间的推移,山东省批发和零售业的销售总额是逐年增大的。具体数值如表1所示。
按此表1,山东省批发和零售业销售总额数据作散点图,如图1,从图中可以清晰的看出,批发和零售业销售总额序列
整体呈现一定的上升趋势,即山东省批发和零售业销售总额是逐年递增的。特别是2010年以后,呈现出强劲的增长势头。该序列为非平稳序列 [13] ,有明显的上升趋势。
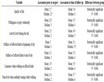
Table 1. Total sales in wholesale and retail industry (unit: one hundred million yuan)
表1. 批发和零售业销售总额(单位:亿元)
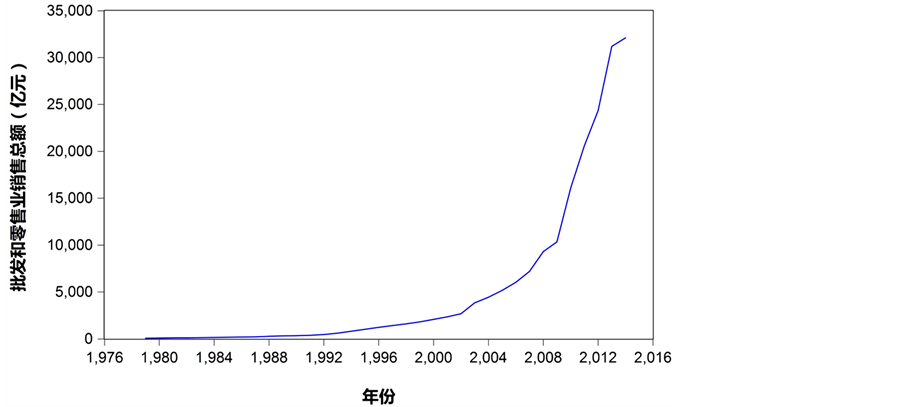
Figure 1. Trend chart of the total wholesale and retail sales
图1. 批发和零售业销售总额趋势
2.2. ARIMA模型原理
ARIMA(p,d,q)模型,即自回归单程移动平均模型具有如下结构
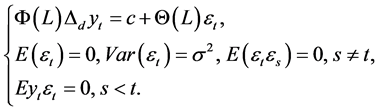
上式中,
为ARMA(p,q)模型的自回归系数多项式,
,
为ARMA(p,q)模型的移动平均系数多项式,并且上式可以简记为
。
式中
是时间序列
的均值,
为零均值的白噪声序列。由此可知,ARIMA1 模型实质上就是差分运算与ARMA 模型的组合。
以时间序列的自相关分析为基础,ARIMA(p,d,q)模型在经济预测的过程中既考虑了经济现象在时间序列上的依存性,又考虑了其随机波动的干扰性,此模型对于经济运行短期趋势的预测准确率较高,是应用比较广泛的时间序列分析方法之一。
若时间序列
通过
次差分后可转换成平稳时间序列,即
,则
,
为平稳序列,即
,于是可建立ARMA(p,q) 模型
,
经
阶差分后的ARMA(p,q)模型称为ARIMA(p,d,q)模型,即自回归单程移动平均模型,其中
为自回归模型的阶数,
为移动平均的阶数,
为白噪声过程。模型参数估计可采用条件最小二乘估计(CLS)或最大似然估计(ML)。
而本文对山东省批发和零售业销售总额的研究,通过往年的历史数据随着时间的变化是如何变化的,进而建立一个模型,使得此模型有效的反映出销售总额随时间变化规律。从而进行未来发展趋势以及销售总额的预测。ARIMA模型法完全符合我们的研究对象,所以我们本文选用ARIMA模型法进行建模分析与预测。
3. 山东省批发和零售业销售总额ARIMA模型
3.1. 数据处理
山东省批发和零售业的销售总额数据变动幅度比较大,是非平稳序列。为了消除原始数据序列的非平稳性,使数据更为平稳,本文采用对山东省批发和零售业销售总额取对数形式(记为
),并利用EXCEL对
序列作一阶差分(记为
),对
序列作二阶差分(记为
),所得数据对一阶差分后的数据
作序列趋势图,见图2 ,从图中可以看出,时间趋势基本消除,可认为是平稳性序列。但序列的趋势图只能粗略地判断序列具有平稳性,理论上应用单位根检验法检验。
序列的趋势图只能粗略地判断序列是否具平稳性不能准确地判断该序列是否为平稳性序列,为了更加准确的判断批发和零售业销售总额数据是否为平稳序列,我们对
进行平稳性检验(ADF 检验),
序列检验
,分别小于三个检验水平不同的临界值
,拒绝了存在单位根的零假设,
是平稳时间序列。
在建模过程中,没有必要进行二阶差分。取对数的山东省批发和零售业销售总额序列为1阶单整序列,即
。
3.2. 模型建立与检验
由以上对序列
的ADF检验,可确定ARIMA(p,d,q) 模型中d的取值为1。同时序列
的
,说明序列
不存在自相关。为了确定ARIMA模型中的p和q的值,我们作序列
的自相关(ACP) 和偏自相关(PACF) 图,见图3。
由图3
序列的自相关与偏自相关图可以看出,ACF表现截尾,但PACF表现拖尾,故选用模ARIMA(0,1,q)型。自相关系数在滞后阶数为时6显著不为零,在滞后阶数大于6时基本都处于置信带内,所以可取
。我们对时间序列
反复拟合,进而得到一个较好的时间序列模型。回归结果如表2。
故估计的ARIMA(0,1,6)模型如下:
.
山东省批发和零售业销售总额随时间演化的模型即得到。对模型的Q统计量进行白噪声检验,即模型检验如图4。
对残差序列进行白噪声检验,可以看出ACF和PACF都没有显著异于零,Q统计量的P值都远远大于0.05,因此可以认为残差序列为白噪声序列,模型信息提取比较充分。常数和滞后六阶参数的P值都很小,参数显著;因此整个模型比较精简,山东省批发和零售业销售总额的ARIMA模型较优。能有效地反应批发与零售业销售总额随时间的变化趋势。
山东省批发和零售业销售总额随时间演化的模型即得到。对模型的Q统计量进行白噪声检验,即模型检验如图5和图6。
对残差序列进行白噪声检验,可以看出ACF和PACF都没有显著异于零,Q统计量的P值都远远大于0.05,因此可以认为残差序列为白噪声序列,模型信息提取比较充分。常数和滞后六阶参数的P值都很小,参数显著;因此整个模型比较精简,山东省批发和零售业销售总额的ARIMA模型较优。能有效地反应批发与零售业销售总额随时间的变化趋势。
表2. 参数估计
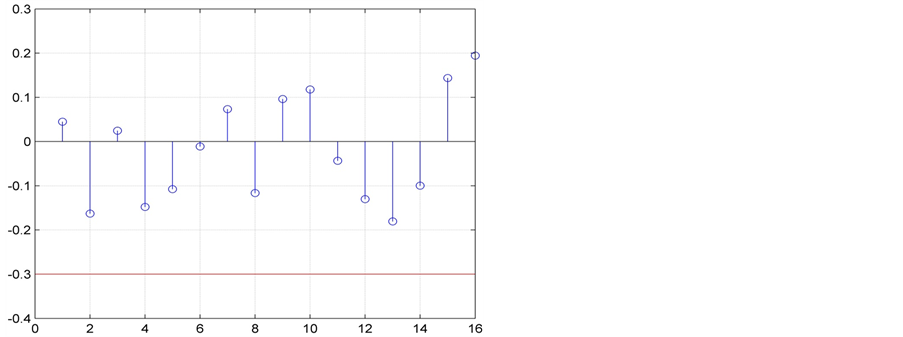
Figure 5. White noise autocorrelation plot
图5. 白噪声的自相关图
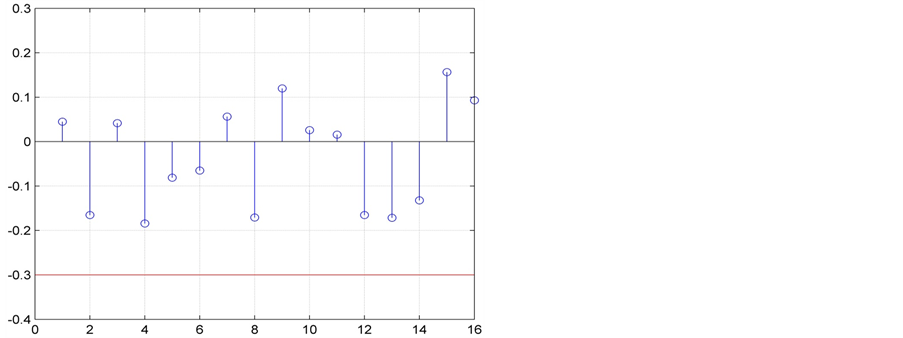
Figure 6. White noise partial autocorrelation plot
图6. 白噪声的偏自相关图
3.3. 模型预测
根据检验和比较的结果,选择最后的方程模型,使用EVIEWS软件中的预测功能对模型进行预测时,如果预测误差较小,就可以用这个模型预测,即可得到原时间序列的将来走势。但是如果预测误差较大,我们就需要重新调整模型,直到选择出合理的预测模型。EVIEWS软件中,预测2007年至2012年的
序列值,预测情况如图7所示。
图中左边是预测值与置信区间,右边是预测的误差。Root mean squared error代表均方误差方,MAE表示平均绝对误差,MAPE表示平均绝对误差百分比。Theil inequality coefficient表示不等系数,bias proportion表示偏误,即预测均值与真实均值的偏离程度;variance proportion表示方差误,用来反映预测波动与真实波动之间的差异;covariance proportion表示协方差误,反映残存非系统性预测误差,该误差占比越大,预测效果越好。本例中的协方差误(0.552111)远远大于方差误(0.367295),因此预测效果较好。
利用ARIMA(0,1,6)模型对山东省2011~2014年批发和零售业的销售总额作预测,并与真实值比较,进一步检验模型的适应性。具体结果见表3。
由表3可知,实际值和预测值相差较小,预测的相对误差都不超过5% ,说明模型非常适合短期预测。通过模型还预测出山东省2015年至2017年批发和零售业销售总额,具体结果见表4。
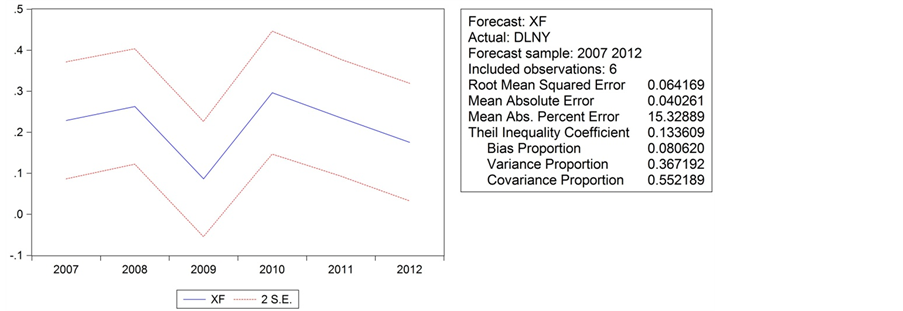
Figure 7. Predictive diagram and related statistics of
sequence
图7.
序列的预测图及相关统计量
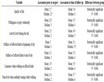
Table 3. Comparison between real and predictive values of total sales in the wholesale and retail industry of Shandong province
表3. 山东省批发和零售业销售总额真实值和预测值的对照
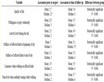
Table 4. 2015-2017 predictive value of the total amount of wholesale and retail sales of Shandong province (unit: one hundred million yuan)
表4. 2015年~2017年山东省批发和零售业销售总额的预测值(单位:亿元)
4. 结束语
ARIMA(0,1,6)模型对于山东省批发与零售业销售总额的预测结果显示其预测值与真实值相对误差小于5% ,从而可以判断此模型对原序列有较好的预测效果。同时预测结果表明,山东省批发和零售业年度销售总额保持较快增长;其中批发和零售业销售总额是社会消费品零售总额的主要组成部分,故社会消费品零售总额也保持较快增长。社会消费品零售总额可以很好的反映人民物质文化生活水平,还能够反映出商品购买力的实现程度和市场规模,批发和零售业销售总额是社会消费品零售总额的主要组成部分,故山东省未来几年的经济将处于快速发展的阶段。