1. 引言
手指的姿态识别具有重要意义,特别是对于手指康复训练 [1] - [6] 而言。手指康复运动的过程中仍存在几个关键问题亟待攻克,包括高精度移动与高控制分辨率的需求,不同人群的数据多变性,以及在运动过程中的模糊信号识别等问题,因而手指的恢复比上肢的其他部位困难得多,而准确的手指姿态识别可以大大提高手指康复的效果。
截止目前,手势识别已被广泛研究,尤其是基于视觉的手势识别。韩国Inda大学和Korea Polytechnic大学的Jong Shill Lee、Young Joo Lee [7] 等人用熵分析法从背景复杂的视频流中分割出手势区域并进行手势识别。使用链码的方法检测手势区域的轮廓,最后计算出从手势区域的质心到轮廓边界的距离。该系统可识别6种手势,平均识别率超过95%;6个人分别做每个手势的识别率平均达到90%~100%。
印度研究者Meenakshi Panwar [8] 在视觉手势识别的基础上提出了一种基于结构特征的手势识别算法,通过背景去除、方向检测、拇指检测和手指数量检测,来最终识别手势。于成龙 [9] 等人采用基于视觉的组合特征进行手势识别,将手的面积、周长、重心、面积比和长宽比等特征结合,使得识别率得以提高。
上海大学丁友勇 [10] 等人运用改进的LBP算法,利用AdaBoost分类器进行手势识别,建立了多种手势数据集,其中包括一些大角度变化的手势图像。实验表明该方法可较好地对手势特征进行分割和分类。
此外,许多大型电子企业 [11] [12] 也将手势识别研究列入其开发项目中,并且成果显著。2012年,三星推出智能电视新品ES8000,该款电视机将面部识别,手势识别和语言识别结合,无论是通过语音还是简单的手势,用户不仅可完成开关机、调节音量、换台等基本操作,还可实现上网浏览或关键字搜索等复杂功能。微软研发机构的微软研究院与华盛顿大学合作,开发出一种可利用笔记本电脑内置麦克风和扬声器进行手势识别的技术;该项技术被称为Sound Wave,利用了多普勒效应识别接近计算机的任何动作和手势。
本文针对手指康复训练中,提出一种简易、便携式的手指姿态识别系统设计。本文其余部分的内容组织如下:在第2节中,对系统框图和硬件设计做了介绍;在第3节,主要介绍了系统的软件设计;在第4节中,搭建了手部动作识别实验,使用多分类支持向量机进行分析和识别手部运的样本数据。利用训练好的多分类支持向量机完成识别手部运动后,在第5节展示了实验结果,第6节总结全文并对展望未来的工作。
2. 系统框图及硬件设计
一种手指姿态实时识别系统硬件结构框图如图1所示。
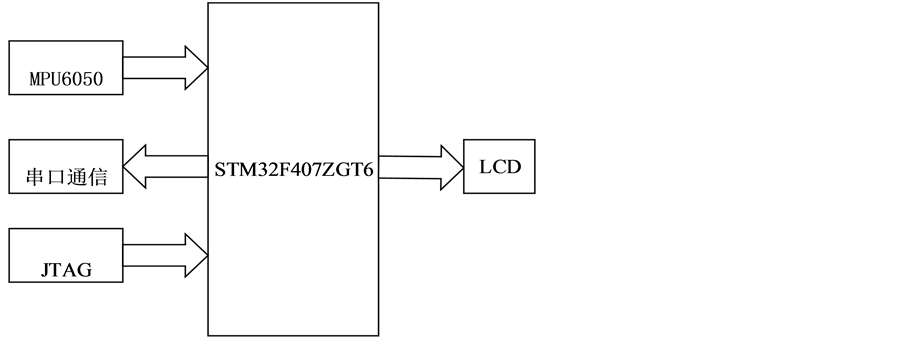
Figure 1. Hardware structure block diagram of finger gesture recognition system
图1. 一种手指姿态识别系统硬件结构框图
LCD显示模块采用ATK-4.3 TFTLCD。采用NT35510驱动,其自带GRAM,这大大节省了MCU内部的RAM使用率。由于本设计涉及到的显示数据较多,利用传统LCD显示器无法保证数据的同时显示,且多色显示有助于区别各个手指的姿态角度,使识别更有效率,所以本设计选用此显示器作为显示设备。
采用JTAG接口。JTAG是一种嵌入式测试技术,它在芯片内嵌入了专用的测试电路 TAP (Test Access Port,测试访问口),通过专用JTAG对内部断电进行测试。
串口通信模块采用CH340G将USB电平信号转换为串口电平信号。CH430G外围电路配置方便,只需将相应引脚的滤波电容与晶体振荡器连接好即可。其与Windows操作系统拥有极好的兼容性,不需做任何修改,即可连接使用。CH430G支持硬件全双工,支持通信频率最高达到2 Mbps,同时还可外加RS232、RS485、RS422等接口来达到电平转换的目的。
角度测量模块采用MPU6050传感器实现数据的采集。MPU6050是9轴运动处理传感器。MPU6050的陀螺仪和加速度计用了三个16位ADC,将其测量的模拟量转化为可输出的数字量。它和外部设备寄存器之间的通信采用400 kHz的I2C接口或1 MHz的SPI接口。在测试过程中发现,MPU6050的内置DMP的运行速率的确不高,很难达到设计目的。因此,本设计中,放弃DMP,而改用姿态解算算法来完成相应任务。
STM32F407ZGT6 [13] [14] 是意法半导体公司STM32F4系列下的一款芯片,因充足的资源,合理的价格和较高的性能而得到了广泛的运用。STM32F407ZGT6的主频可以达到168 MHz,是本设计的理想选择。
本设计采用MPU6050实现姿态信息的获取,数据传输采用IIC总线的形式,使用资源少,并适合有一定距离的传输。由于试验要求对手指多个关节进行角度测量,所以使用了6个MPU6050传感器,并将相应的供电接口和数据传输接口布局在硬件电路一侧,方便连接。
3. 软件设计
一种手指姿态实时识别系统能够正常工作,不但需要可靠的硬件设计,还需要一个优化的软件设计。本设计是基于STM32F407ZGT6的软件设计,利用Keil μVision4集成开发环境对芯片进行编程开发。系统软件的主程序流程如图2所示。
由主程序流程图可以看出,当系统开始工作时,首先对STM32F407ZGT6时钟系统进行初始化操作,随后对外设进行初始化操作,进而判断整体初始化是否成功,如果成功,则进一步进行LCD框架文本的
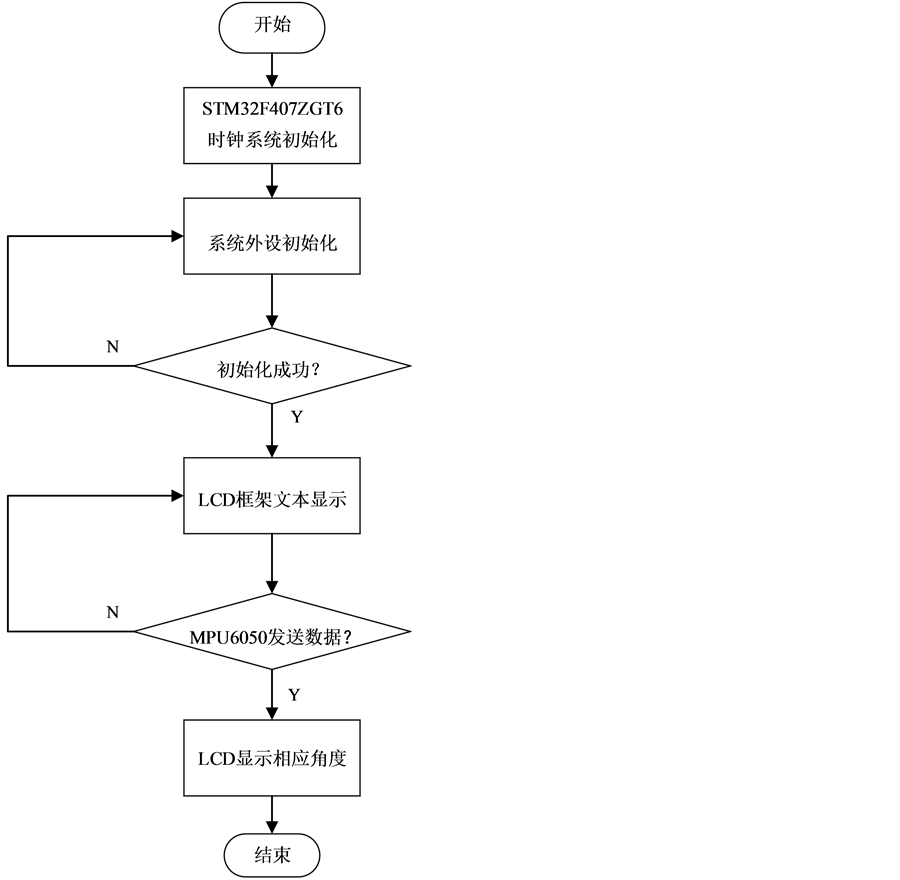
Figure 2. Working flow chart of the system
图2. 系统工作流程图
显示;如果未成功,则返回重新进行初始化操作。下一步继续检测MPU6050模块是否成功发回数据。如果发送成功,则用LCD显示相应角度;如果未成功,则返回文本显示,等待数据的发送。
4. 手部动作识别
1) 硬件模块结构
如图3所示,本文提出一种简易且成本低廉的方法来检测更精细的手部运动。
STM32F407ZGT6作为CMU连接LCD、COM接口、JTAG、和3D传感器组,3D传感器选用集成了三个16位模数转换器以采样模拟数据的MPU6050。
若将六个传感器被放置在一个薄的手套,在手套的不同位置可以检测到的精确地横摇角,偏航角和螺旋角。
2) 手部动作
1, 2, 3, 4, 5, 6, 3D传感器
Figure 3. Hardware module design of normal hand
图3. 正常手的硬件模块设计
试验中的一组运动包括握钢笔、握手柄、握水瓶、做“OK”手势、做“V”手势,通过多组三维角度传感器测量得到三个手指的弯曲数据如表1所示。
显然,不同的人戴上手套后会产生不同的数据,甚至有时候一个人戴手套两次得到的数据都不是完全相同的,这种数据模糊性的问题会给识别带来困难,而多分类支持向量机可以解决这类问题 [15] [16] [17] [18] [19] 。
手指姿态识别主要分为两部分:首先是离线学习训练阶段,指派多人穿戴好数据手套分别做多个不同动作,其一部分用做训练样本,一部分用于测试样本,针对不同动作的角度变化数据,采用交叉验证确定多分类支持向量机的参数。然后是在线预测阶段,实验者穿戴数据手套做动作时,将得到的多分类支持向量机实时检测动作时产生的角度变化数据,达到识别手指姿态的目的。
5. 实验分析
10名志愿者被选中参与实验,他们被分别安排握一支钢笔,握手柄,握一个瓶子,做“OK”手势,做“V”手势,如图4~图8所示,每个动作重复八次。所有的数据均被分组记录下,共400组数据,其中随机抽取200组作为训练集和剩下的200组用于识别数据作为测试集。
由于多分类支持向量机的研究与应用已经很成熟 [15] [16] [17] [18] [19] ,这里不过多阐述理论,只介绍多分类支持向量机的应用。多分类支持向量机在这里作为一个学习机。我们选择的特征值组成特征向量,包括:梯度平方和(S),平均值(M),方差(V)。
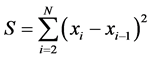
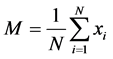
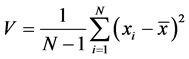
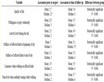
Table 1. Sampled data of different motion
表1. 不同运动的采样数据
向量[S, M, V]作为特征向量,用径向基函数计算高维空间中的内积。
在多分类支持向量机对初始数据的处理过程中,经过大量训练逐渐接近最优参数。经过多次计算,确定了径向基函数的惩罚参数和宽度参数作为最优参数:
,
。
剩下的200组数据用于识别测试已经训练好的多分类支持向量机。最终,多分类支持向量机在识别“握水瓶”和“握笔”时仅仅产生了1次交叉性错误,“握手柄”和“握笔”时仅仅产生了3次交叉性错误,结果表明多分类支持向量机可以准确识别手部三跟手指的运动姿态,误差率仅有2%。
6. 结论
为提高手指康复的康复效果,本文提出了一种实时手指姿态识别系统设计,以简单廉价的方式检测正常手的手势动作。在4次的识别错误中,可以分析手指姿态识别的识别错误原因,一方面是由于交叉性错误的两种运动比较相似,另一方面是由于传感器组测量的数据可能存在一定误差。在未来,传感器的精度将愈发被重视,结合手指的外骨骼可穿戴式机械手的手指结构,对系统的测量误差进行补偿后,本系统可以开发用于家庭手指瘫痪病人的日常康复训练。
基金项目
国家自然科学基金(61663027)。
NOTES
*通讯作者。