摘要: 针对电站锅炉燃烧过程存在的高度的复杂性和非线性问题,本文采用模糊支持向量机(FSVM)建立含氧量预测模型,预测在不同燃料量、总风量、总给水量等因素的影响下烟气含氧量的含量。选取模糊C均值算法(FCM)作为隶属度函数的设计方法,然后选取径向基核函数(RBF)和ε-SVR模型结构,其中惩罚因子和松弛变量的最佳参数值要用交叉验证法来选取。Matlab仿真实验结果表明,该方法有效地缩短了训练时间,提高了预测精度和模型的抗噪性,其性能优于一般支持向量机预测模型。
Abstract:
For power plant boiler combustion process of a high degree of complexity and nonlinear problem, fuzzy support vector machine (FSVM) is adopted to establish the prediction models of oxygen forecast in different fuel quantity, total air volume and total yield, total steam flow under the in-fluence of factors such as flue gas oxygen content. We select fuzzy c-means algorithm (FCM) as a design method of membership function, then select the radial basis kernel function (RBF) and ε-SVR model structure, and choose the penalty factor and the optimum parameter value of slack variable to use cross validation method. Matlab simulation experiment results show that this method can effectively shorten the training time, improve the prediction precision and the model of noise resistance; and its performance is superior to the general support vector machine forecasting model.
1. 引言
科技飞速发展,人们对能源的需求也日益剧增。在各种一次性能源的消费比例中,煤炭几乎占世界能源总需求的30%,煤炭作为一次性能源重要组成部分的地位在相当一段时间内不会改变。提高锅炉效率降低煤耗节约能源是一极其重要的任务。电厂中锅炉烟气含氧量是一项重要的参数。烟气含氧量的测量成为重点研究过程。有专家运用支持向量机 [1] 建立模型进行预测,然而由于模糊信息的存在,SVM对孤立点和噪声过分敏感,容易出现过学习现象 [2] ,因此提出模糊支持向量机算法,提高抗噪性。因此本文提出FSVM为烟气含氧量测量过程建模,并通过仿真实验验证FSVM预测模型的有效性。
2. 基于模糊支持向量机的预测模型
回归SVM通过核函数将特征向量 [3] 从非线性空间映射到某一高维空间(Hilbert空间),然后在新空间中求解一个典型的二次规划问题,得到全局最优解,从而在特征空间中应用线性方法解决高度非线性回归问题。假设样本集为
,其中
为输入向量且
为系统的输出且
,n为样本数据的个数。一般支持向量机的回归模型可表示为:
(1)
(1) 式中
为输入空间到特征空间
的映射函数;
为权值向量,
;
偏置量。
对于标准SVM来说,优化问题为:
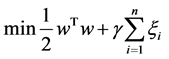
(2)
(2) 式中,
为松弛变量,
为预算误差的惩罚系数用于调节误差所取的作用,它能够使训练误差和模型复杂度之间取一个折衷,以便使所求的函数具有较好的泛化能力,并且
值越大,模型的回归误差 [4] 越小。FSVM是近几年提出的一种新方法,是对传统SVM的改进和完善。为了提高SVM的抗噪能力,对每一个样本引入模糊隶属度
且
则优化问题改进为:
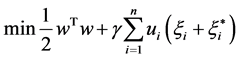
(3)
其中
同样是惩罚系数,
和
是允许的回归误差。在(3)式中引入拉格朗日乘子,同时根据KKT条件引入核函数
,得到FSVM求解问题的对偶形式:

(4)
其中,
为拉格朗日乘子,利用最优化理论中的二次规划方法可以得到。模糊支持向量回归机的估计式为:
(5)
其中
为偏移量 [5] ,可通过下式求得:
(6)
在FSVM模型的计算过程中,使用隶属度ui将惩罚系数模糊化,不同样本具有不同的隶属度 [6] ,这样在计算的过程中也考虑到了孤立样本,可有效地提高模型的抗噪性能,进而达到较高的预测精度。
3. FSVM模型建立
3.1. 模糊隶属度函数的计算
模糊隶属度函数的计算采用模糊C均值聚类(FCM)算法,是目前较为常用的聚类分析方法。该方法采用了在欧几里得空间确定数据点的几何贴近度的概念,将这些数据分配到不同的聚类,然后确定聚类之间的距离,每一个数据点按照一定的隶属度隶属于某一聚类中心。
3.2. 核函数和初级参数的选取
使用FSVM模型进行预测需要使用核函数,由于RBF核函数计算简单,同时它将训练样本点非线性地映射到无穷维的特征空间 [7] ,可有效地处理非线性关系,因此本文采用RBF核函数。
根据从DCS历史数据中得到的300组训练样本来计算模型中的最佳参数c和g,其寻优的方法采用函数SVMcg For Regress的交叉验证方法 [8] [9] 进行寻优,最终寻得参数bestc = 4,bectg = 1.31950791077289。
3.3. 基于FSVM软测量模型的流程图
基于FSVM软测量模型的流程图见下图1。
3.4. 仿真实验结果及分析
根据上述模型的建立步骤,从DCS历史数据中选取300历史数据做样本。本文使用FSVM方法为烟气含氧量过程建立预测模型,对决定烟气含氧量和它的影响因素之间的高度非线性函数关系进行较为精确的拟合,根据当前的条件预测下一时刻烟气含氧量的含量。根据输入量与输出量的相关度 [10] ,利用相关系数法选取该模型的输入为燃料量、总风量、总给水量、总蒸汽流量、炉膛压力、主汽压力、主汽温度、锅炉负荷,输出为烟气含氧量。考虑到模型的平衡性,300组数据中使用150组数据用于训练FSVM模型,其余150组用于测试(图2)。
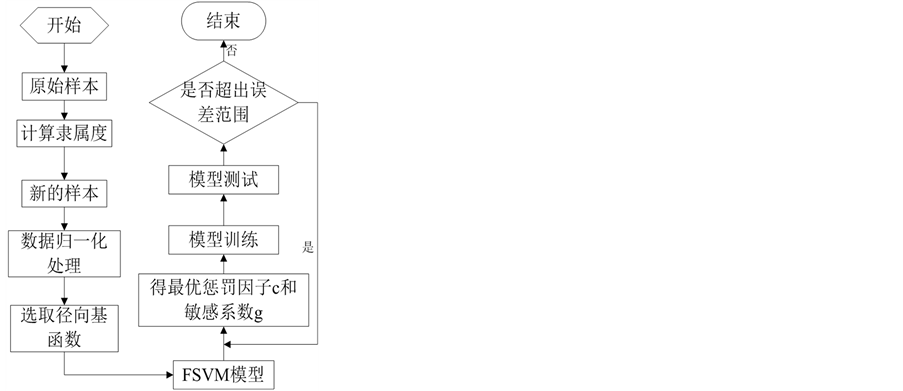
Figure 1. Flow chart of FSVM soft meas- urement model
图1. FSVM软测量模型的流程图
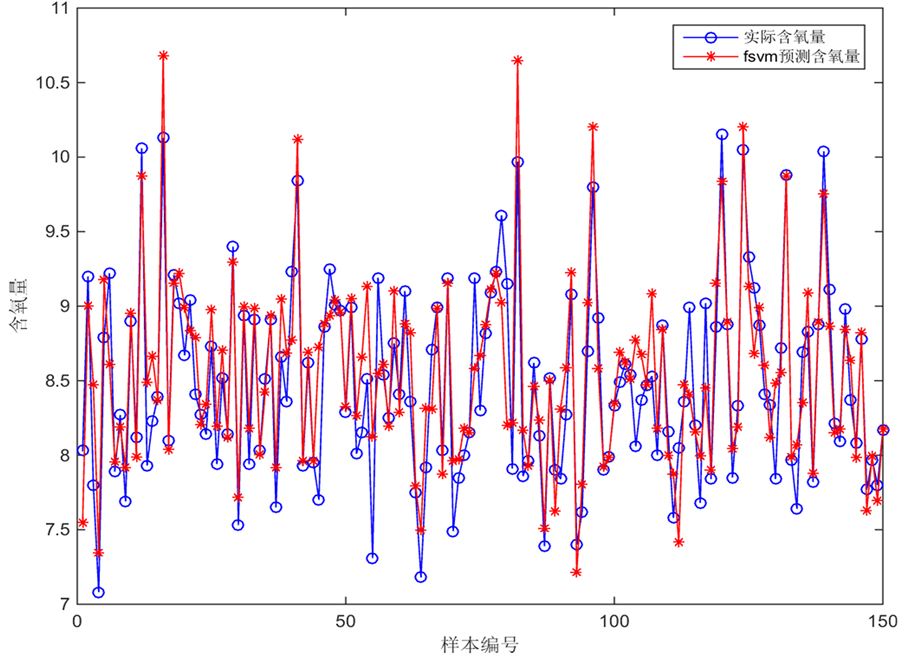
Figure 2. FSVM model predicted results compared with the actual oxygen
图2. FSVM模型预测结果与实际含氧量比较
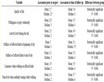
Table 1. FSVM compared with SVM experimental results
表1. FSVM与SVM实验结果对比
从表1中不难看出,FSVM与SVM相比误差小,相关度高,运行时间少。
4. 结束语
针对标准SVM预测模型对孤立点过分敏感,模型的容错性差的问题,提出一种基于FSVM的烟气含氧量的预测模型建立方法。FSVM模型方法引入了模糊隶属,具有较强的抗噪性能,可大大地提高预测模型的预测精度,仿真实验表明该方法的有效性。