1. 引言
步态识别是运用步态的普遍唯一性 [1] ,通过提取人走路过程中步态特征,经过分类识别,最终实现辨别人物身份,达到分析行为特征的目的。一般包括轮廓检测、特征分析、分类识别三个主要研究步骤。其中特征提取是步态识别的关键环节,特征提取的好坏对最终识别率影响很大。步态特征的提取方法主要分为基于模型与基于整体两种方式。典型的基于模型的特征处理有钟摆模型 [2] 、棍状模型 [3] 和椭圆模型 [4] 。基于模型的特征提取对于遮挡和噪声有较强的鲁棒性,但计算量大,对序列变化敏感度高,难以实现实时处理。基于整体的特征提取也称为基于全局的特征提取,无需建立先验模型,而是通过分析目标动态变化与轮廓信息提取合适的特征,相对基于模型的特征提取实时性好,但对于视角和服饰变化等鲁棒性较差。N.V.Boulgouris 等人采用随机变换提取单帧特征,并使用LDA对周期累计特征矢量进行降维 [5] 。Dong Xu等人提出一种新的局部分布式特征(PDF),他们将每帧动态能量图(GEI)表示为局部增量Gabor特征组,将其从5个尺度和8个方向组合为新特征矢量 [6] 。Mohan Kumar HP等人提出变式能量图(CEI),将特征值重构为区间值,通过随机变换提取判决特征,实验表明该特征对于穿衣、背包等变化具有良好的识别鲁棒性 [7] 。
当前,步态识别的最新研究在国外各学术期刊和会议上频繁出现,主要聚焦在视角变化、衣帽穿戴、实时处理等问题上,步态识别的研究越来越接近实际,如何提高算法的鲁棒性和实际情况下的识别率成为未来的一个研究难点。现有的算法多采用整体特征或模型特征进行单一特征提取与识别,整体特征反映的是目标的轮廓特点,粒度较粗;而模型特征则反映了目标的局部动态特征,粒度又过细,在实际问题中具有一定局限性。针对这些问题,本文提出一种新的区域面积序列特征(RAS),将人体通过采样划分为若干区域矢量,提取身体不同部位在运动过程中的面积变化特征,并将均值形状PMS、动态能量图GEI、区域面积序列RAS这三类不同粒度的步态特征构建成特征字典,最后采用基于联合多特征字典稀疏表示算法,去除不同特征间冗余信息的同时实现特征融合。在CASIA B标准库上进行的仿真实验表明,该算法在90度视角下能够达到较高识别率,在三种不同视角下鲁棒性均优于对应的单特征识别。
2. 多特征提取
步态是一种连续不断的运动,是一个过程,有别于传统的人脸、指纹等一代生物技术识别方法,而且步态序列中每一个运动目标的身体特征也都是不一样的,如身高体重、形态和服装等。因此我们可以用形状特征来作为它的静态特征,并根据目标的运动情况提取它的动态特征。
2.1. PMS特征提取
Procrustes均值形状(PMS)分析法是方向统计学中一种广泛使用的方法,通常适用于编码二维形状,并提供了一种好的工具来寻找一组图形的均值形状,因此它可以用来描述人体步态特征 [8] 。
采用PMS分析的特征提取方法如下:
Step 1. 采用Canny算子提取图像步态轮廓,将所有轮廓点表示为以人体质心为坐标原点的极坐标值,并以等间隔的角度对轮廓线进行采样,得到轮廓的一维极坐标向量表示。
Step 2. 通过极坐标到直角坐标的逆变换将每个形状表示为一个复数向量
。其中
,
为采样后的轮廓坐标,
为采样频率。为将形状置于坐标空间中心位置,定义中心向量
,其中
,
;
Step 3. 当序列中含有
帧图像时,可得到
个中心配置向量,计算配置矩阵
,得到矩
阵的特征值及对应特征向量;
Step 4. PMS的均值形状
对应
的最优配置,即最大特征值对应的特征向量,将其作为步态序列的统计特征用于识别。
图1给出了三个图像序列的PMS均值形状,从图中可以看出不同个体在同一视角下的均值形状差别较大。
2.2. GEI特征提取
用加权平均方法将一个周期内步态序列图像合成的一幅能量图像,称之为步态能量图(GEI)。GEI既包含了原有周期图像序列中走路频率、相位变化等动态信息,同时有效的减少了统计步态图像序列包含
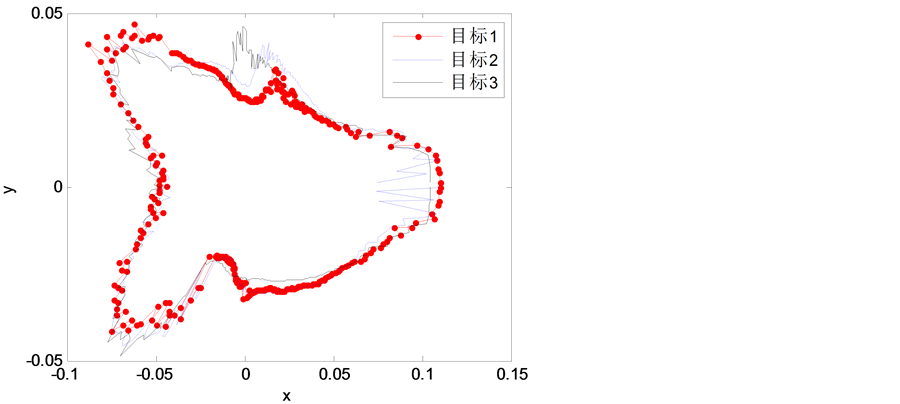
Figure 1. The mean shape of the three goals
图 1. 三个目标的均值形状
信息所需的数据量 [9] 。
对于一个周期内包含
帧图像的步态序列,其GEI的定义见式(1):
(1)
其中
表示第
帧图像在
处的像素值。本文从测试库中选取的2个人11帧的步态序列及其能量图如图2所示。
在生成步态能量图后,采用主成分分析法PCA对GEI进行特征降维 [10] 。针对含有56个步态能量图的训练集,设定PCA降维数为25,图2中两幅步态能量图在特征空间的表示如图3所示。
2.3. RAS特征提取
为了描述运动人体各区域随时间变化状态,挖掘运动人体步态图像各区域所包含的深层信息,本文提出一种新的区域面积序列特征RAS。该特征将二值化图像中人体各区域随时间的面积变化特征转化为特征向量来表示运动过程中的周期性状态变化。
以人体质心点与轮廓采样点连线,将每帧步态图像分为若干区域,各个区域的面积可采取等分角度采样方法计算,如图4所示。若将图像等分为
个区域,则这
个区域可用
条经过人体质心的直线约束划分,直线的斜率
对应角度
,
的正切值,以第一象限为例,
所划分的各区域可表示为:
(2)
式(2)中
为轮廓采样点坐标,
为坐标值。进行等分角度提取后,设各区域面积为


Figure 2. Gait sequences and energy maps
图 2. 步态序列及其能量图
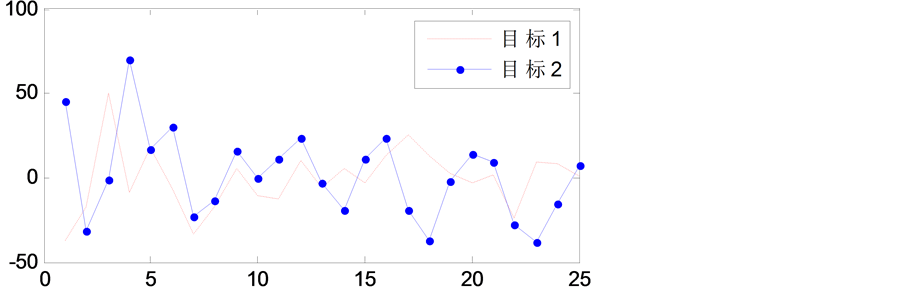
Figure 3. Dimensionality reduction by PCA
图3. PCA降维
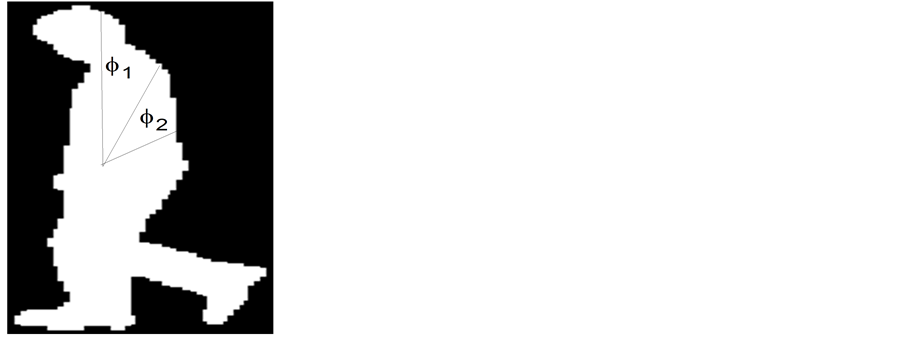
Figure 4. Feature extraction by RAS
图 4. RAS特征提取
,则第
副步态能量图的区域面积特征向量
,对于包含
幅图像的步态序列,其RAS特征向量表示为
。
提取出RAS特征后,采用PCA主成分分析法对RAS特征进行降维处理。
图5给出了按角度24等分采样的一幅步态图像的RAS特征。
图6给出了图2中第一个步态序列对应的RAS特征。
3. 基于联合多字典稀疏表示的步态识别
基于联合多字典稀疏表示的步态识别算法框架如图7所示。算法首先通过计算步态轮廓均值形状得到PMS静态特征,同时分别对步态序列周期图像进行加权平均获得GEI动态特征,提取区域面积序列构成RAS动态特征,并对两种动态特征进行PCA降维以减少信息冗余。其次,基于PMS、GEI、RAS特征针对步态序列分别构建对应特征字典,并在构建的特征字典上进行多任务联合稀疏表示,约束条件为每种特征字典上的稀疏表示系数趋于一致(同样的稀疏表示图形)。最后,通过Accelerated Proximal Gradient (APG)算法求得联合多任务稀疏表示系数的最优解,利用它计算三个特征字典累计残差最小即可得到测试样本类别。
式(3)为联合多字典稀疏表示计算公式:
(3)
其中,
为测试步态序列,
为特征字典数目,本文选用了3种特征,因此设定
,
为训练步态序列,
为求解的联合多任务稀疏表示系数。这是一个基于
混合范数约束的多任务最小二乘回归问题,可以通过APG算法求解得到。
通过APG算法求得联合多任务稀疏表示系数的最优解
后,算法的决策条件由3个特征字典的累计重建残差最小得出:
(4)
累计重建残差
最小类则为识别结果。其中
为每个特征字典赋予的权重,权重越大表示该特征对识别结果的影响越大。本文设置
均为1,表示赋予每个特征字典同样权值,对算法识别率的影响保持等量。
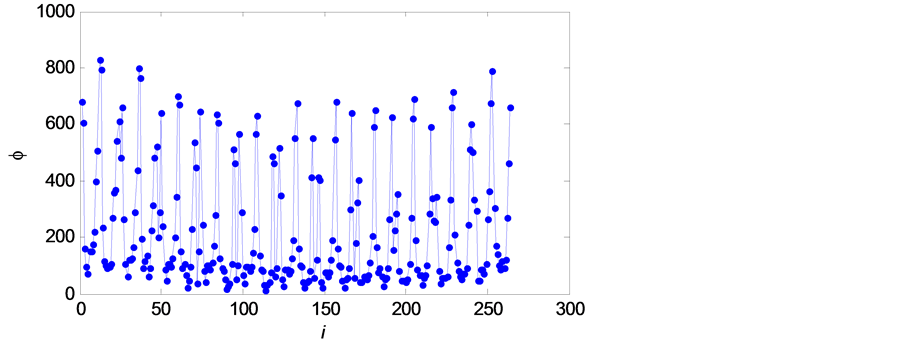
Figure 6. The RAS feature corresponding to gait sequence
图6. 步态序列对应的RAS特征
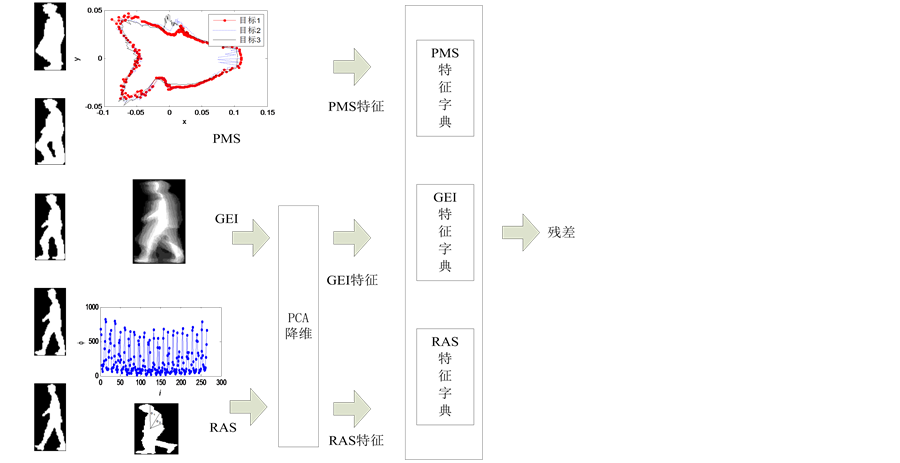
Figure 7. The framework of gait recognition algorithm based on joint multi- dictionary sparse representation
图7. 基于联合多字典稀疏表示的步态识别算法框架
4. 实验步骤与结果分析
4.1. 实验步骤
当前各国为便于研究建立了多个步态数据库用于公开研究测试,但相对其它生物特征数据库数量偏少,样本规模和复杂度有待提升。CASIA (Chinese Academy of Sciences Institute of Automation)步态数据库由中科院自动化研究所建立,分为小规模库(Dataset A)、多视角库(Dataset B)、红外库(Dataset C)3个子数据库 [11] 。其中Dataset B标准库于2005年建立,由124人从0˚至180˚以11种不同视角分别拍摄,每个视角包含6个正常行走序列、2个穿大衣行走序列、2个背包行走序列,总计13640个图像序列,序列规格统一为25 fps,每帧320 * 240像素,使用较为广泛。
本文选用CASIA B库进行仿真实验。从CASIA B库的124人中随机选取56人作为训练样本,每个样本分别包含180˚、90˚、54˚三种视角下的1个正常行走序列(11帧步态图,归一化为150 * 90像素,周期由宽高比变化确定),训练库共计1848帧步态图像。
测试库由标准库中余下的68个样本组成,测试时从对应视角下正常行走序列中随机选取步态图像,测试步骤如下:
Step 1. 将测试样本图像规整到150 * 90大小,提取RMS、GEI与RAS特征;
Step 2. 采用PCA方法对GEI与RAS特征进行降维处理,对每种特征采用最近邻分类器计算单一特征下的识别率;
Step 3. 采用联合多特征字典稀疏表示方法,计算三个特征融合下的识别率,与单一特征下的识别结果进行比较;
Step 4. 调整PCA降维数,测试维数对识别结果的影响;
Step 5. 改变稀疏度,测试稀疏度对识别结果的影响;
Step 6. 对比三个视角下的识别性能。
4.2. 实验结果与分析
表1给出了90˚视角下采用步骤2中单一特征识别与本文多特征联合识别的结果对比。其中PCA降维数为35,稀疏度为9,PMS特征提取采样间隔为1˚,RAS特征提取采样间隔为15˚。
可以看出,在单一特征识别方面,GEI + PCA特征识别率最高,PMS特征次之,RAS + PCA特征识别率最低。而本文所采取的静态特征PMS与动态特征GEI、RAS进行多特征融合方式能够得到最高识别率,实现了各个特征之间的信息互补。
表2给出了PCA降维数对识别率的影响,PCA降维数分别取40~15,间隔为5,稀疏度为9,PMS特征提取采样间隔为1˚,RAS特征提取采样间隔为15˚。从变化趋势来看,本文的多特征联合识别方法识别率随着PCA维数的降低而降低,降维数为35时可以在兼顾算法效率的同时取得最优识别率。
表3给出了不同稀疏度对识别率的影响,稀疏度分别取1~11,PCA降维数为35,PMS特征提取采样间隔为1˚,RAS特征提取采样间隔为15˚。可见,识别率随着稀疏的增大趋势性增大,在稀疏度等于9时识别率达到最大,当稀疏度继续增大时,识别率有所波动。
表4给出了180˚、90˚、54˚不同视角下几种特征提取的识别率。本文所采用的多特征联合识别方法在几种视角下均取得了较高的识别率。对比不同视角的识别率,90˚视角较54˚视角与180˚视角的识别率高,这是因为在视角为90˚时,人体步态的大多数动态特征(如腿部、手臂的摆动等)均能从步态图像序列中有效提取,然而在54˚、180˚视角下,腿部、手臂等部位的动态信息不够明显,不能被很好的提取,或者人体图像的一部分被分割成背景,导致其识别率较低。
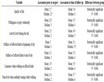
Table 1. Comparison of single feature recognition and multi-feature joint recognition results at 90 degree perspective
表 1. 90˚视角单一特征识别与多特征联合识别结果比较
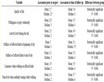
Table 2. The influence of PCA dimension reduction on recognition results at 90 degree single view
表 2. 90˚单一视角PCA降维数对识别结果的影响
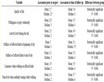
Table 3. Influence of different sparse degree on multi-feature joint recognition
表 3. 不同稀疏度对多特征联合识别结果的影响
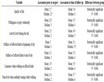
Table 4. Recognition results at different perspectives
表 4. 不同视角下的识别结果
5. 小结
针对步态识别中单一特征提取与分类在实际场景分析中识别率较低的问题,本文选择三种不同粒度的特征PMS、GEI与RAS,通过联合多字典稀疏表达构建特征字典,实现特征层融合。在CASIAB标准库上进行的仿真实验结果表明,本文所采用的多特征联合识别算法在不同视角下均优于单特征识别,降低冗余度的同时实现了特征之间的信息互补。