1. 引言
近年来,中药材价格呈现普遍上涨的态势,三七作为中药材中的大宗品种,其价格波动更显剧烈。2010年三七120头的年平均单价在310元左右,最高价为510元,2011年相较2010年价格有小幅的上涨但波动不大。而到了2012与2013年120头三七最高价猛长至800元左右,2015年则跌至155元,三七价格波动的剧烈程度可见一斑。
药材价格波动严重干扰了市场的稳定,也给种植三七的药农和以三七为元材料的药企带来巨大的不确定性。因此,运用相关数学模型对药材价格的周期性和波动性进行分析,对未来三七价格走势进行预测,为药农种植和药企采买提供决策支持是很有意义的。
2. 三七价格特性分析
2.1. 三七价格上涨及波动的定性分析
影响三七价格上涨和波动的原因众多,主要因素包括需求、成本以及投机行为三个方面。
2.1.1. 需求拉动三七价格上涨
三七是治疗心血管疾病药物的主要成分,其指向性明显,具有其他药物无法替代的功效 [1] 。随着我国心脑血管疾病的发病群体的不断加大,对以三七为原料的治疗心脑血管疾病药物的需求也呈递增态势,特别是三七总皂苷、三七血栓通、复方丹参片和复方丹参滴丸等药品在市场中日渐走俏。因此,在需求拉动下,三七价格不断地上涨。
2.1.2. 成本推动三七价格上涨
中药材种植成本包括种子种苗价格、劳动力成本、土地成本、交通成本等。自2009年10月以来,三七种苗从过去的0.1元/株,涨到2013年的1元/株;三七种子的价格也从2009年10月之前的几十元/kg,涨到2009~2014年最高时,达到1000元/kg。另外,三七对地理位置、光、热、水、土、气候等条件要求较高,我国适合种植三七的地区有限,随着三七价格上涨,药农跟风种植三七,对土地需求增加,而适宜种植三七的土地有限,导致地租上涨,三七种植成本进一步增加。
2.1.3. 投机因素加剧三七价格的上涨及波动
中药材具有投机品特性,各大药企囤货助推了三七价格的上涨。三七作为治疗心脑血管疾病药物的主要原材料,其市场需求比较稳定,因此,投机资本是导致三七价格波动极大的偏离价值曲线的重要因素。
2.2. 三七价格波动定量分析
2.2.1. 自然因素对价格的影响
1) 种植面积对价格影响分析
从2010年到2013年,三七的价格经历了历史上最大的涨幅,从100元一直涨到800元。图1为2006~2013年云南省三七种植面积变化折线图,图2为2006~2014年云南地区三七产量变化折线图。综合从网上搜集的大量资料可知,此次涨价的诱因主要是云南省2009年到2011年间较低的采挖面积和连年大旱,另外药企和经销商囤积药材也加剧了此番价格增长。
2) 降水量对价格的影响
三七生长发育期要求比较湿润的环境 [2] ,查看有关三七的新闻和快讯可知每年有关“干旱”的内容集中在每年的第一季度,新栽种三七同时也在三月份左右出苗,因此我们观察一下1到4月的降雨量。图3是文山州附近月值降水量统计。
直观上看旱情较严重的三年的共同特点是2月和3月的降水量偏低,4月份降水量比较充足,一般是旱情解除的月份;而同时尽管2011年2月的降水量极低,但是3月极高的降水量在一定程度上缓解了旱情,所以2011年的三七报道并未关注旱情。综合来看图中红色着重的年份为旱情较为严重的3年,同时也是三七价格飙升的三年,因此降雨量对三七价格具有显著影响。
3) 寒潮对价格的影响
三七产区的地貌复杂,地势起伏大,造成气温的垂直差异显著,冬春季及夏末秋初,受大气环流冷空气侵袭的影响,造成短时性及局部的霜冻和低温灾害,特别是低温冷害,气温骤然降低至作物生长的临界值以下造成的危害较大,这类情况几乎隔数年就可能出现 [2] [3] 。图4在日值价格曲线上用红圈标出了2010~2016年间云南地区5次较大的暴雪和寒潮的发生日期。
观察可知,只有前两次寒潮之后价格曲线上升,而后三次寒潮之后价格并没有改变甚至在第3次寒潮后还下降。另外第一次寒潮过后价格的上升并没有超过其附近的价格波动区间,可以认为寒潮对这次上升的贡献几乎可以忽略不计。进一步观察可知在第一次寒潮过后11天左右价格发生上升,在第二次寒潮过后17天左右价格发生的上升,中间间隔了很长的时间,综上我们认为寒潮并不会抬升三七的价格。
在气候因素中,目前只发现干旱这种长时间大面积气候现象会对三七的价格整体走势产生影响。另外,降雨量和采挖面积是影响价格整体走势的两个重要因素。在整体走势的预测中,降雨量可通过天气预报提前估计,而今年的采挖面积也大概可以通过去年的二年生三七在地面积普查估计。
2.2.2. 三七价格波动规律分析
影响三七价格波动的外界因素众多,并且各自之间存在较强的相关性。然而,各因素之间的相关关系、对波动的影响程度最终都体现在价格上,因此本文从历史价格走势来对未来价格进行预测。
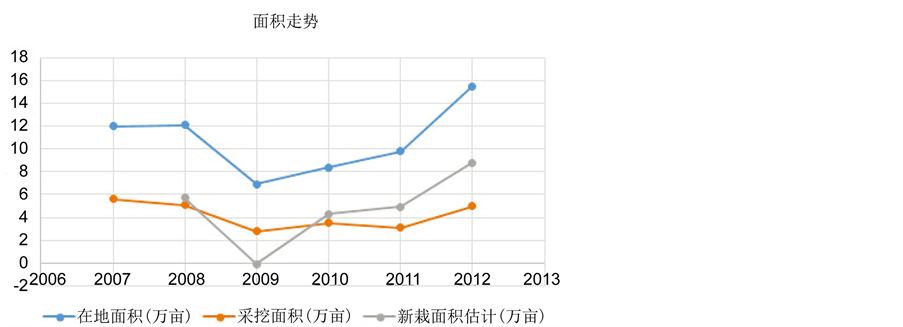
Figure 1. 2006-2013 Yunnan Province pseudo-ginseng planting area change line chart
图1. 2006~2013年云南省三七种植面积变化折线图
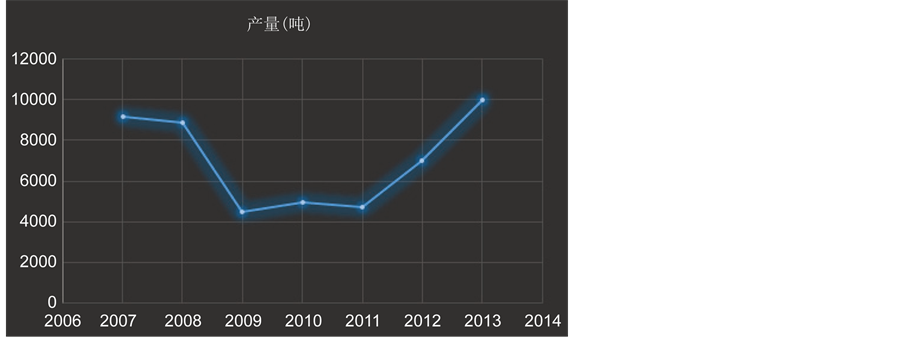
Figure 2. 2006-2014 Yunnan region pseudo-ginseng years production change line chart
图2. 2006~2014年云南地区三七产量变化折线图
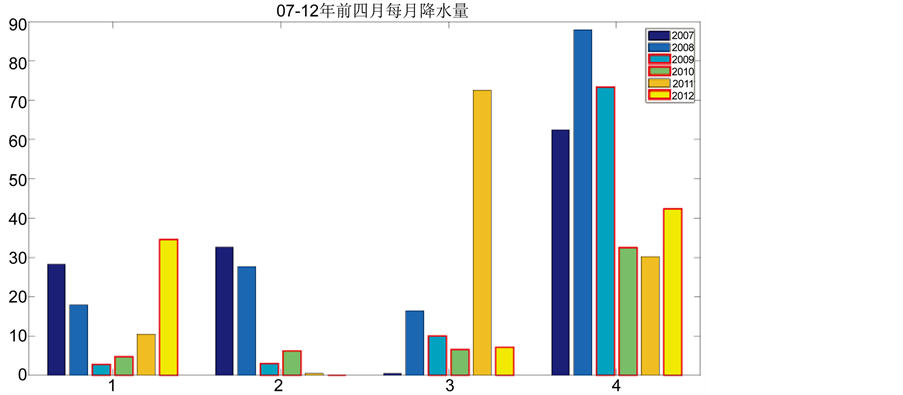
Figure 3. 2007-2012 in Yunnan 4 months before the annual precipitation histogram
图3. 2007~2012年云南每年前4个月降水量柱状图
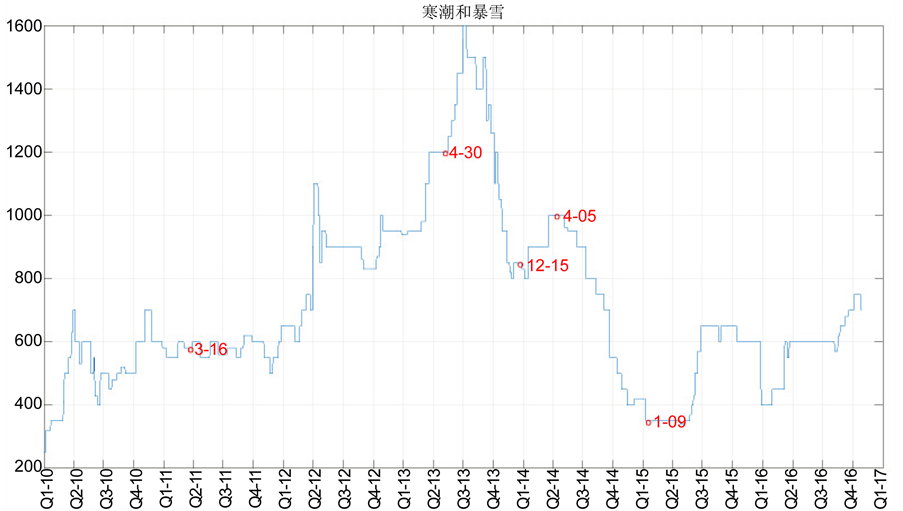
Figure 4. 2010-2016 years of cold wave in Yunnan
图4. 2010~2016年间云南地区寒潮记录
1) 三七价格周期性变化规律
图5为2010年至2016年每年12个月份120头三七的月平均价格。我们将2010年与2014年、2011年与2015年、2012年与2016年的价格曲线图进行对比发现:虽然整体价格高低有差距,但每组中两条曲线的走势大致相同。
为了更直观的观察,本文给出了将每组的价格曲线对比图,分别如图6、图7和图8所示。
2010年与2014年的对比图中可以看出,2010年与2014年的120头三七价格都是在第12周左右开始上涨,在第21周左右出现下跌,又在43周左右小幅回升。
2011年与2015年进行对比发现,这两年三七价格都相对平稳,并且都在年末时出现上扬趋势。
2012年与2016年价格曲线进行对比,这两年的价格走势也是大致相同。
由以上三组价格曲线图的对比情况,我们可以初步确定,三七价格波动周期大概为四年,而这与三七的生长周期正好吻合。三七的生长周期长达3年,当市场上价格出现下跌时,再减少三七的种植面积已经来不及了,当年产的三七3年前就已经种植在地里,三七价格表现在市场上会有一定的滞后,因此确定三七的价格波动周期为四年左右。
2) 三七价格季节性变化规律
图9为2010年至2016年每年四个季度三七平均价格折线图。从图中我们可以清晰的看出:每年第一季度末第二季度初和第三季度末第四季度初,三七的价格会出现大幅度上涨,波动较为剧烈。
在统计学中,方差反映了数据的波动情况。为了说明以上价格波动情况的猜想的正确性,通过绘制价格方差折线图来进行验证。如图10所示,三七价格方差在每年的2、3月份和9、10月份较大,说明三七价格在这四个月波动明显。
三七价格的季节性波动情况也正好符合三七的采收情况。在交易市场中,三七分为冬三七和春三七。冬三七在每年春节过后进行采挖,3、4月份上市;春三七为每年8、9月采挖。春节过后,随着药企购货计划的开启和新三七的上市,三七的购销热度上升,价格也随之上涨。
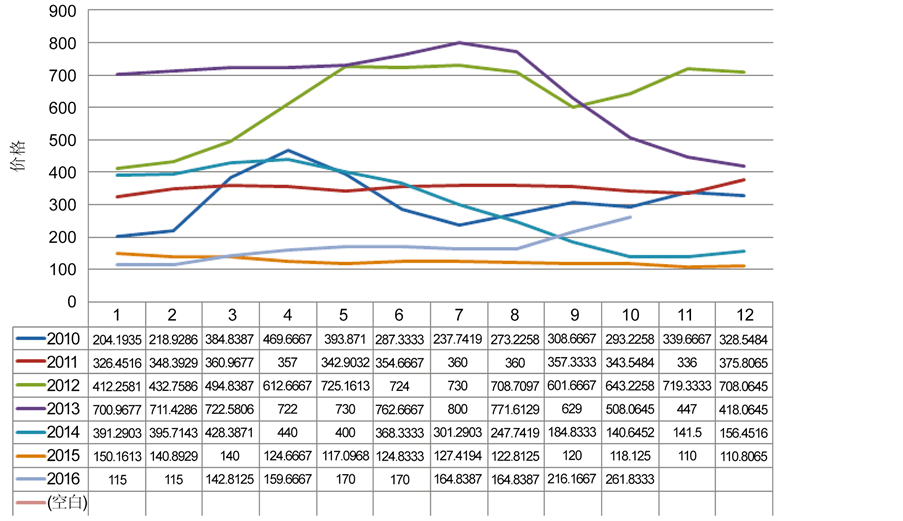
Figure 5. 2010-2016 120 price of pseudo-ginseng (monthly average) trend
图5. 2010年至2016年120头三七价格(月均价)走势
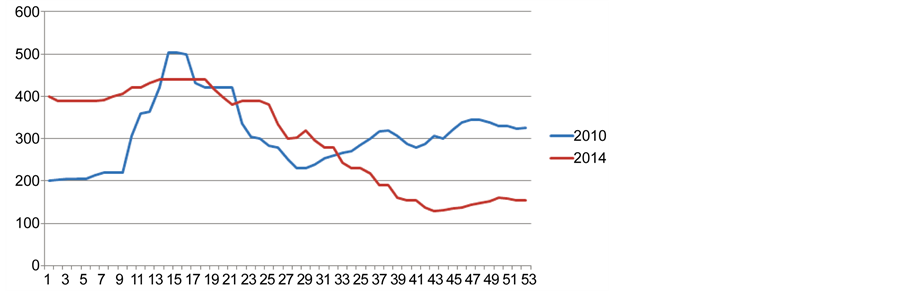
Figure 6. Price comparison between 2010 and 2014
图6. 2010与2014年价格曲线对比
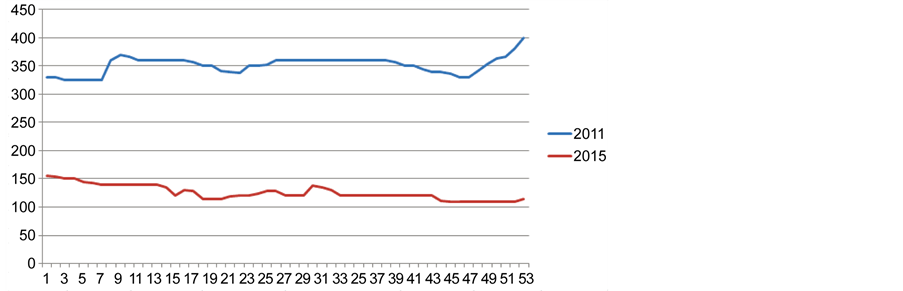
Figure 7. 2011 and 2015 price curve comparison
图7. 2011与2015年价格曲线对比
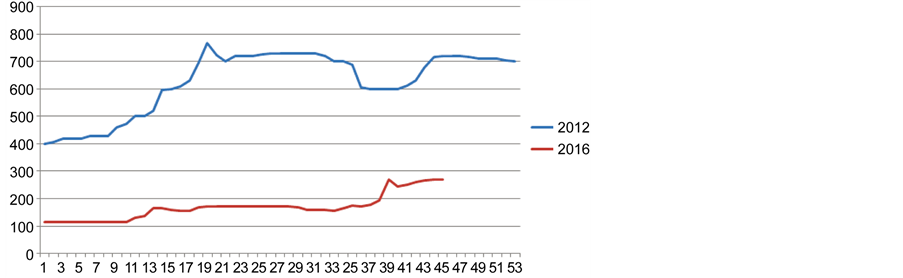
Figure 8. 2012 and 2016 price comparison curve
图8. 2012与2016年价格曲线对比
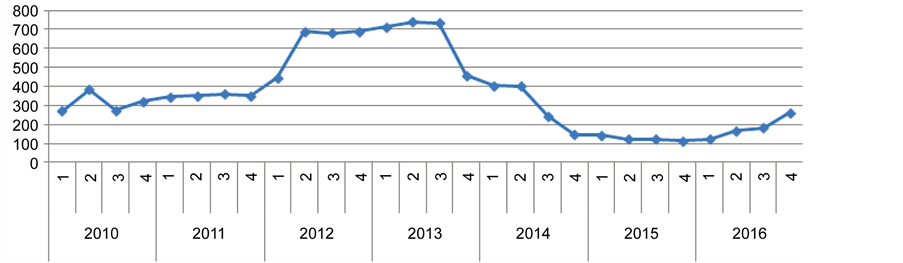
Figure 9. The average price chart of pseudo-ginseng
图9. 120头三七季度平均价格走势图
3. 基于ARIMA的三七价格预测
3.1. ARIMA模型
通过三七价格数据分析,三七价格曲线具有时序性、周期性和季节性的特点,因此三七价格预测属于典型的时间序列分析问题。
自回归移动平均(ARMA)模型是传统的时间序列分析模型,其广泛应用于股票、期货等时间序列分析 [4] 。ARIMA模型以AR模型和MA模型为基础“混合”构成,包含了自回归和移动平均2种成分 [5] 。

模型记作ARMA(p,d,q),其中d代表对原序列进行差分的阶数,p代表自回归成分的阶数,q代表移动平均成分的阶数;
是自回归系数,描述了
对
的影响程度;
为移动平均系数,
是随机干扰误差项 [6] 。
3.2. 数据预处理
1) 时间维度确定
实验分析所用数据为2010年1月至2016年3月每天的三七价格,为了提高计算精度和效率,需要首先确定价格频域(时间维度)。
2010至2016年间,2010年的价格小幅波动最为频繁,因此选取2010年的价格数据作为分析对象,来确定最大的有效时间维度。
图11为2010年三七日价格曲线,从中可以看出,一周7天内的价格无明显差异;而一个月中各周间的波动则较为明显,因此选取前1个月的数据来预测下月里每周的平均价格。
2) 数据归一化
不同年代经济水平不同使得价格基数不同,为了消除这种影响需要对数据进行归一化处理。另外,进行归一化处理后的数据可以消除不同量纲之间的影响,同时加快程序运行时的收敛速度。
3.3. 数据集介绍
实验所用数据集为2010年1月至2016年10月每周的平均价格,其中2010年至2015年的数据作为训练集,2016年1月至3月的数据作为测试集。每一样本包括每月五周的周均价,即:
。2010年至2015年共5年,60条样本,因此测试集为
的矩阵。如表1所示为2010年的样本资料阵。
3.4. 模型建立
通过使用EVIEWS软件对云南文山地区2010年1月至2015年12月每周的价格进行分析和预测。
1) 序列平稳性检验
时间序列分析过程中,为防止回归分析中存在伪回归,不能够真实的反映因变量和解释变量之间存在的均衡关系,因此需要对序列进行单位根检验,以确定序列的平稳性。通过对2010年1月至2015年12月每周的三七价格进行ADF检验,图12为ADF检验图。从图中可以看出ADF值为−0.88426均大于显著性水平分别为1%,5%,10%时的临界值,因此该序列不稳定。
2) 差分平稳化
对原始序列进行差分变换可以将不平稳序列转换成平稳序列。实验中对原序列进行一阶差分,再次对差分后的序列进行单位根检验,得到一阶差分序列单位根检验结果如图13所示,其ADF值为−21.12798,均小于显著性水平为1%,5%,10%的临界值,因此可确定差分后的序列为平稳序列,且d = 1。
3) 模型定阶与数据预测
模型定阶过程主要是为了确定p、q两个参数,通过逐步试探方法最终确定模型ARIMA(2,1,2)为最佳模型。利用ARIMA(2,1,2)模型对2010年1月至2015年12月的价格进行拟合预测,从图14可以看出模型拟合偏差为0,方差为0.000024,所以ARIMA对训练数据的拟合效果较好。
使用所得模型对2016年1月至3月的价格进行预测,预测值与实际值对比如图15所示。
从图15中可以看出ARIMA模型对2016年前两个月的三七价格预测比较准确,但3月份数据偏差较大。综上所述,ARIMA模型能较好的预测短期数据的趋势,但对于非线性变化规律预测效果较差。
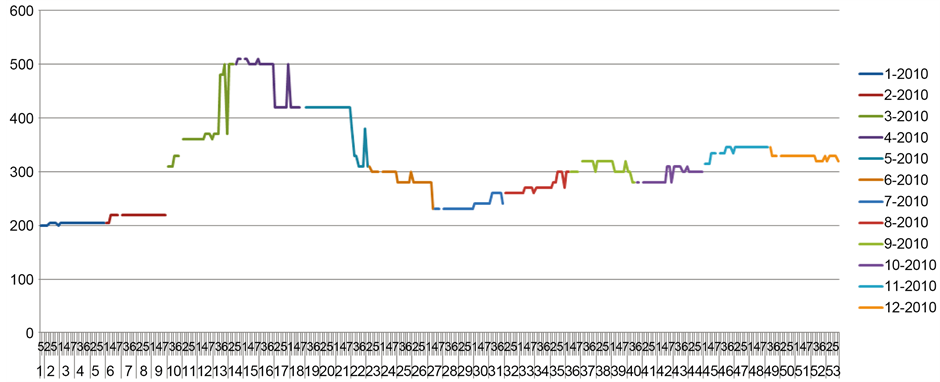
Figure 11. Price fluctuation curve of pseudo-ginseng int the year of 2010
图11. 2010年三七日价格波动曲线
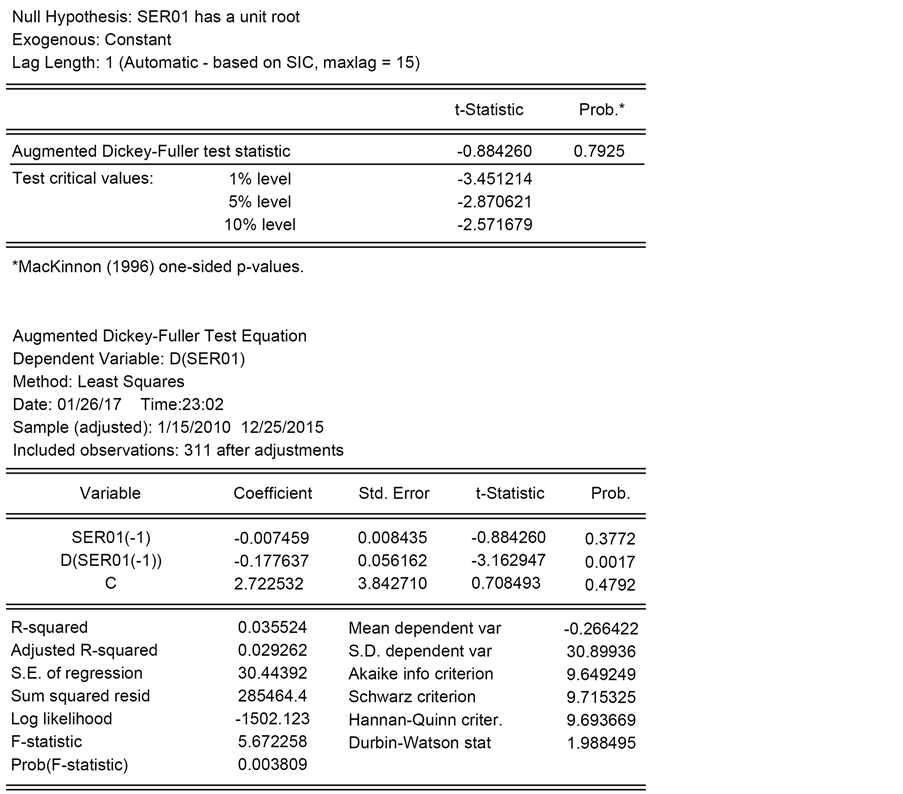
Figure 12. Initial sequence unit root detection results
图12. 初始序列单位根检测结果图
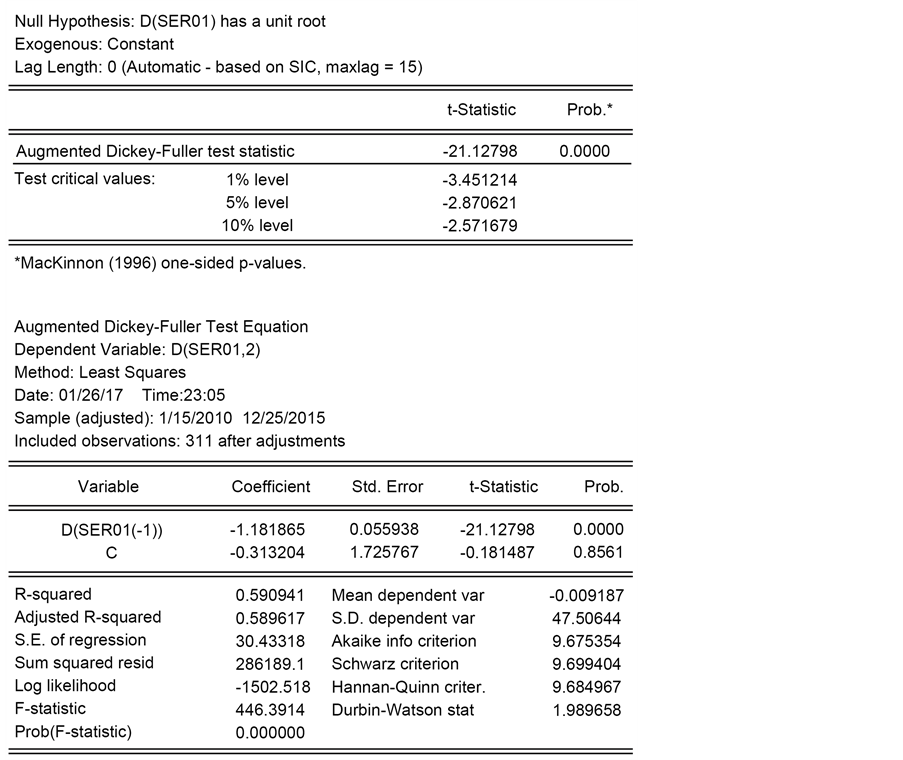
Figure 13. First-order differential unit root test
图13. 一阶差分单位根检验
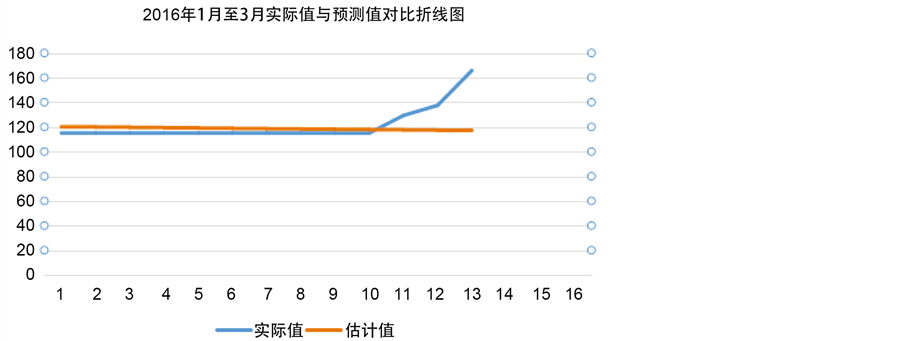
Figure 15. Comparison of the mean and predicted values of the monthly average for January to March 2016
图15. 2016年1月至3月周均值实际值与预测值对比图
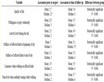
Table 1. Weekly price of pseudo-ginseng sample data matrix in 2010
表1. 2010年120头三七周价格样本资料阵
4. 基于BP神经网络的三七价格预测
ARIMA模型虽然对短期数据变化趋势具有较好的预测效果,但其对非线性变化因素的感知能力较差 [7] ,对价格波动曲线中的离群点预测效果不佳。而BP神经网络属于多层感知器,适用于求解非线性可分问题。为解决这个问题,引入BP神经网络算法。
4.1. BP网络预测模型
要解决非线性可分问题,需要使用具有多层功能神经元的神经网络模型。本实验选用“多层前馈神经网络”作为预测模型,使用误差反向传播算法进行参数调整。
如图16所示为“M-P神经元模型”,神经元接收来自n个其他神经元传递过来的输入信号,并通过带权重的连接进行传递,通过“激活函数”处理以产生神经元的输出。多隐层前馈神经网络模型,输入层神经元接收外界输入,隐层与输入层神经元对信号进行加工,最终结果由输出层神经元输出。
实验所用数据集为2010年1月至2016年10月每周的平均价格,训练集、测试集、检验集按70%、15%、15%进行随机划分。由于周数据的数据量较少(360组),根据Haykin提出的经验性公式 [8] 决定使用10周的数据来预测下一周的数据。因此,BP网络输入层包含10个节点,输出层为一个节点。
网络层次结构确定之后,需要设置层与层之间信息传递所用传递函数。根据实际交易经验,将初始学习率设为0.1,期望达到的最小错误率为4 × 10−5;另外,具体实验表明当迭代次数达到10,000次之后,模型错误率趋于稳定,因此迭代次数设为10,000次。网络结构参数设定如表2所示。
在其他条件不变的情况下,分别用logsig和tansig作为激活函数对整理后的数据进行测试,选取预测结果误差较小的tansig函数为输入层与隐含层的传递函数;通过控制变量法在保证其他条件不变只改变隐层神经元个数的情况下发现隐含层节点为9个时预测误差较小。输出层传递函数一般选用purelin函数,以保持输出的范围 [9] 。
实验结果如图17所示,神经网络的预测结果相较于ARIMA有了较大提升,价格在跌势时下降的更快,对跌势预测地更精确,嗅觉更灵敏。
4.2. BP网络结构优化
神经网络的关键问题为确定网络结构,包括输入节点个数,隐层数,隐层节点个数和输出层节点个数 [10] [11] 。实际问题中,根据经验直观判断网络输入输出节点个数往往无法取得好的预测结果,既费时又费力。因此,不能仅根据经验值来确定网络的结构,为了解决此问题,引入相空间重构 [12] [13] 对BP神经网络结构进行优化。
1999年,H.S. Kim等人基于嵌入窗法的思想提出了C-C方法,该方法使用关联积分同时估计出时延与嵌入窗,是估计相空间重构参数延迟时间
和延迟时间窗
的有效方法 [16] 。
估计延迟时间
和延迟时间窗
的C-C方法通过计算以下3个量:
(1)
(2)
(3)
其中,
,σ为给定时间序列的标准差,
。由
的第一个极小值所对应的时滞t计算
,由
的最小值所对应的时滞
计算时间窗
,
为
采样间隔。
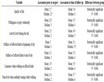
Table 2. BP network structure related parameters table
表2. BP网络结构相关参数表
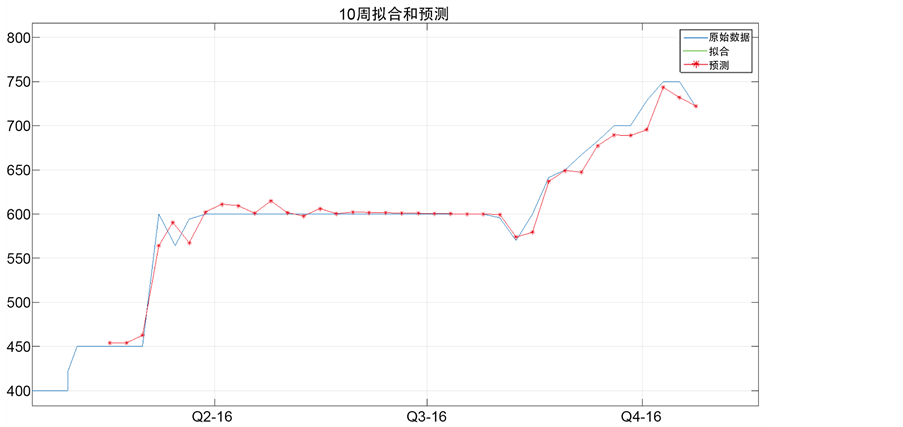
Figure 17. Comparison of predicted and true values of preliminary neural networks
图17. 初步神经网络预测值与真实值对比图
C-C方法中关键的量
定义为
(4)
其中,m = 2,3,…,关联积分为
(5)
价格曲线具有一定的混沌性 [14] ,借助相空间重构确定BP网络的输入维数。首先使用C-C方法计算相空间的嵌入维和时间延迟 [15] 。结果图18所示。
从图18观察得到时延和嵌入窗口的变化趋势,时延在7 s时取得最小值。根据Haykin et al.提出的经验性公式确定BP网络的输入维数为7,即使用7周的数据来预测下一周的数据。另外,为了让网络学习到周期性额外增加一维ID,用来标志目标周在一年内的位置,如一年52周中的第3周,其ID为3。网络只设置一层隐含层,隐含层有8个节点,包括上一时刻的输出结果和本时刻的输入。最终所得优化后的BP神经网络结构如图19所示。
2) 网络训练与预测
优化后的BP神经网络的三七价格预测模型所用数据集仍为2010年1月1日~2015年12月31日的三七周价格,训练集、测试集、检验集按70%、15%、15%进行随机划分。优化后BP神经网络对2016年的预测效果如图20所示。从图20中可以相较于输入节点为10个的神经网络,优化后的BP神经网络在涨势预测地更精准一些,在跌势时预测价格下降缓慢,预测值曲线更贴近于真实值曲线。
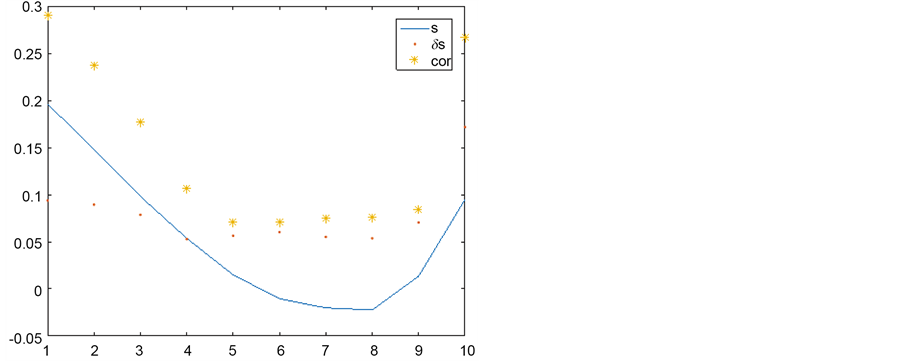
Figure 18. Phase space embedded dimension and time delay analysis results
图18. 相空间嵌入维与时间延迟分析结果图
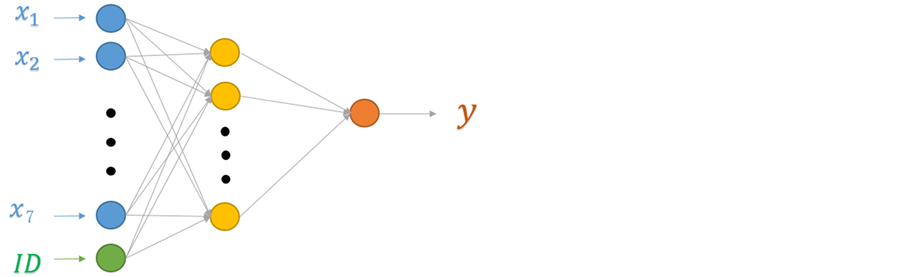
Figure 19. Optimized BP neural network structure
图19. 优化后BP神经网络结构图
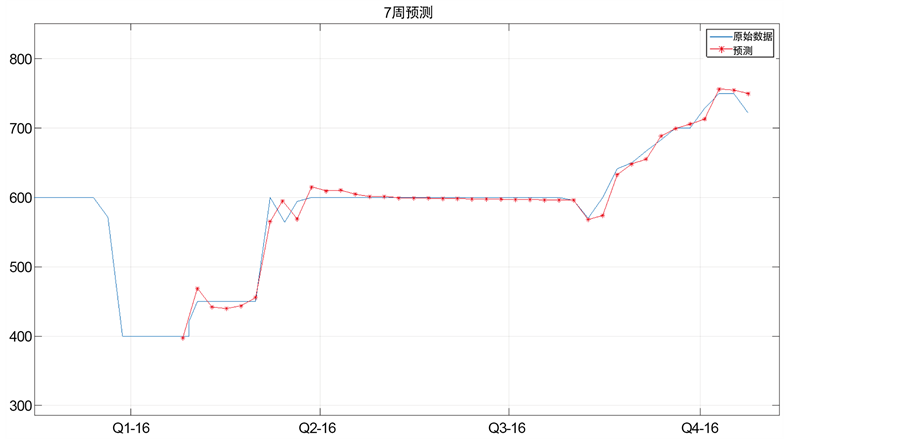
Figure 20. BP neural network 2016 price evaluation
图20. BP神经网络2016年三七价格预测
5. 结论
此次实验中共使用了ARIMA、BP神经网络对2010年1月至2015年12月的周价格进行学习,利用2016年1月至3月的周价格对模型进行预测,实验结构表明BP神经网络对非线性价格波动曲线具有更好的预测效果。另外,为了更客观的确定神经网络结构,提出了相空间重构的方法来确定神经网络输入节点的个数,进一步提高了神经网络的预测精度。
未来的研究方向可以考虑使用集成算法将ARIMA算法和BP神经网络进行结合,使用组合模型同时提取价格曲线的线性和非线性规律,达到更好的预测效果。
基金项目
天津市重大科技专项(14ZXDZGX00867, 15ZXDSGX00090);天津市互联网先进制造专项(15ZXHLGX00360, 15ZXHLGX00380)。