摘要: 目的:探讨胃癌周围神经侵犯的独立危险因素,同时建立诊断预测模型。方法:收集自2017年01月至2020年12月在延安大学附属医院行胃癌根治术的543例胃癌患者临床病理资料,根据术后病检结果将胃癌患者分为周围神经侵犯阴性组(275例)和周围神经侵犯阳性组(268例)两组。对纳入本研究的临床病理指标进行单因素Logistic回归分析,得到具有统计学意义的指标。将这些指标进行多因素Logistic回归分析,继而得出胃癌周围神经侵犯的独立危险因素,此外应用Hosmer-Lemeshow拟合优度检验拟合效果。建立胃癌周围神经侵犯的诊断预测模型,同时应用ROC曲线和单因素Logistic回归分析验证该模型的诊断价值。结果:单因素Logistic回归分析结果表明体重、BMI、淋巴细胞、单核细胞、红细胞、血红蛋白、血小板、NLR、PLR、LMR、白蛋白、CEA、CA19-9、CA72-4、肿瘤最大直径、肿瘤分化程度、肿瘤组织类型、肿瘤浸润深度、淋巴结转移以及癌结节是具有统计学意义的指标。多因素Logistic回归分析结果提示肿瘤最大直径(P = 0.010, OR: 1.226, 95% CI: 1.049~1.433)、肿瘤分化程度(P < 0.001, OR: 3.000, 95% CI: 1.660~5.423)、肿瘤浸润深度(P < 0.001, OR: 6.323, 95% CI: 2.766~14.453)、淋巴结转移(P < 0.001, OR: 42.884, 95% CI: 11.470~160.338)以及癌结节(P < 0.001, OR: 17.454, 95% CI: 6.759~45.071)是胃癌周围神经侵犯的独立危险因素。此外应用Hosmer-Lemeshow拟合优度检验得知该五项临床病理指标拟合效果较好(χ2 = 7.581, P = 0.475)。根据独立危险因素可以建立胃癌周围神经侵犯的诊断预测模型为:P = ex/(1 + ex),x = −4.118 + 0.204 × 肿瘤最大直径 + 1.099 × 肿瘤分化程度 + 1.844 × 肿瘤浸润深度 + 3.759 × 淋巴结转移 + 2.860 × 癌结节。ROC曲线的曲线下面积为0.944,渐近95% CI为0.926~0.961。预测模型的诊断临界值为0.5122,该临界值对应的敏感度为0.836,特异性为0.935。此外该诊断模型的预测正确总体百分比87.1%以及单因素Logistic回归分析结果均提示该模型预测准确率较高。结论:肿瘤最大直径、肿瘤分化程度、肿瘤浸润深度、淋巴结转移以及癌结节是胃癌周围神经侵犯的独立危险因素。本研究建立的胃癌周围神经侵犯诊断预测模型其敏感度和特异性均较高,且预测准确率较高,提示该预测模型的诊断价值良好,具有较好的实践应用价值。
Abstract: Objective: To explore the independent risk factors of perineural invasion in gastric cancer, and to establish the diagnostic prediction model. Methods: The clinicopathological data of 543 patients with gastric cancer who underwent radical gastrectomy in the Yan’an University Affiliated Hospital from January 2017 to December 2020 were collected. According to the postoperative pathological examination results, the gastric cancer patients were divided into two groups: the perineural invasion negative group (275 cases) and the perineural invasion positive group (268 cases). Univariate Logistic regression analysis was performed on the clinicopathological indicators included in this study, and statistically significant indicators were obtained. Multivariate Logistic regression analysis was performed on these indicators to determine the independent risk factors for perineural invasion in gastric cancer. In addition, Hosmer-Lemeshow goodness of fit was used to test the fitting effect. The diagnostic prediction model for perineural invasion in gastric cancer was established, and the diagnostic value of the model was verified by ROC curve and univariate Logistic regression analysis. Results: Univariate Logistic regression analysis showed that body weight, BMI, lymphocytes, monocytes, erythrocytes, hemoglobin, platelet, NLR, PLR, LMR, albumin, CEA, CA19-9, CA72-4, maximum tumor diameter, degree of tumor differentiation, tumor tissue type, depth of tumor invasion, lymph node metastasis and tumor deposit were statistically significant indicators. Multivariate Logistic regression analysis showed that maximum tumor diameter (P = 0.010, OR: 1.226, 95% CI: 1.049~1.433), degree of tumor differentiation (P < 0.001, OR: 3.000, 95% CI: 1.660~5.423), depth of tumor invasion (P < 0.001, OR: 6.323, 95% CI: 2.766~14.453), lymph node metastasis (P < 0.001, OR: 42.884, 95% CI: 11.470~160.338) and tumor deposit (P < 0.001, OR: 17.454, 95% CI: 6.759~45.071) were independent risk factors for perineural invasion in gastric cancer. Hosmer-Lemeshow goodness of fit test showed that the five clinicopathological indicators had good fitting effect (χ2 = 7.581, P = 0.475). According to the independent risk factors, the diagnostic prediction model for perineural invasion in gastric cancer could be established as follows: P = ex/(1 + ex), x = −4.118 + 0.204 × maximum tumor diameter + 1.099 × degree of tumor differentiation + 1.844 × depth of tumor invasion + 3.759 × lymph node metastasis + 2.860 × tumor deposit. The area under the ROC curve was 0.944, and the asymptotic 95% CI was 0.926~0.961. The diagnostic cut-off value of the predictive model was 0.5122, which corresponded to a sensitivity of 0.836 and a specificity of 0.935. In addition, the prediction accuracy of this diagnostic model was 87.1% and the results of univariate Logistic regression analysis indicated that the prediction accuracy of this diagnostic model was high. Conclusion: Maximum tumor diameter, degree of tumor differentiation, depth of tumor invasion, lymph node metastasis and tumor deposit are independent risk factors for perineural invasion in gastric cancer. The diagnostic prediction model for perineural invasion in gastric cancer established in this study has high sensitivity and specificity, and high prediction accuracy, suggesting that the prediction model has good diagnostic value and good practical application value.
1. 引言
胃癌是一个全球性的健康问题,每年全世界新确诊病例数超过100万,尽管在过去的50年里,胃癌的发病率和死亡率均有所下降,但胃癌仍然是癌症相关的第三大死因 [1]。同时我国胃癌发病例数和死亡例数分别约占全球的42.6%和45.0%,疾病负担严重,是癌症防治的重点 [2]。周围神经侵犯是指癌细胞扩散到神经周围空间的过程,它被认为是一种更具有侵袭性的肿瘤表型的重要预测指标,并预示着前列腺癌、膀胱癌以及胰腺癌等许多癌症的不良预后 [3] [4]。周围神经侵犯是胃癌转移的重要途径之一,也是导致胃癌根治术后局部复发和癌痛的重要原因;同时胃癌的不良预后与淋巴结转移和血行转移密切相关,部分是由周围神经侵犯引起的;此外在胃癌的发生发展进程中,神经、血管和淋巴管等因素共同构成肿瘤微环境,对肿瘤细胞的各种生物学特性发挥决定性作用 [5]。虽然目前有关胃癌周围神经侵犯危险因素的研究已有报道,但是很少有学者对其构建诊断预测模型,因此亟待进一步探索。本研究通过回顾性分析延安大学附属医院收治并行手术治疗的543例胃癌患者临床病理资料,探讨影响胃癌周围神经侵犯的独立危险因素,进而建立胃癌周围神经侵犯的诊断预测模型,以期为今后胃癌周围神经侵犯的预防、诊断和治疗策略的研究提供新的参考依据。
2. 资料与方法
2.1. 研究对象
收集自2017年01月至2020年12月在延安大学附属医院行手术治疗的543例胃癌患者临床病理资料,根据术后病检结果将胃癌患者分为周围神经侵犯阴性组(275例)和周围神经侵犯阳性组(268例)两组。纳入标准:① 接受标准的胃癌根治术,同时术后病检确诊为胃癌;② 无术前放化疗史;③ 具有完整的临床病理资料。排除标准:① 既往因胃癌接受过内镜下治疗后追加手术者;② 术前接受过放化疗者;③ 临床病理资料不完全者。本研究已通过延安大学附属医院伦理委员会审批,所有患者及家属均已签署知情同意书。
2.2. 研究指标
本研究列入下述临床病理指标:① 患者一般资料,包括性别、年龄、身高、体重以及体质指数(body mass index, BMI);② 患者术前血常规相关指标,包括白细胞、中性粒细胞、淋巴细胞、单核细胞、红细胞、血红蛋白、血小板、中性粒细胞–淋巴细胞比率(neutrophil-lymphocyte ratio, NLR)、血小板–淋巴细胞比率(platelet-lymphocyte ratio, PLR)以及淋巴细胞–单核细胞比率(lymphocyte-monocyte ratio, LMR);③患者术前肝功能相关指标,包括白蛋白和白球比;④ 患者术前消化道肿瘤系列相关指标,包括癌胚抗原(carcinoembryonic antigen, CEA)、糖类抗原19-9 (carbohydrate antigen 19-9, CA19-9)以及糖类抗原72-4 (carbohydrate antigen 72-4, CA72-4);⑤ 患者ABO血型;⑥ 患者术后病检相关指标,包括肿瘤最大直径、肿瘤位置(贲门胃底区、胃体区、胃窦幽门区)、肿瘤分化程度(高分化 + 中分化、低分化)、肿瘤组织类型(腺癌、非腺癌)、肿瘤浸润深度(T1 + T2、T3 + T4)、有无淋巴结转移以及有无癌结节。
2.3. 统计学方法
采用SPSS 26.0软件对数据进行统计分析。对纳入本研究的临床病理指标进行单因素Logistic回归分析,得到相应的P值、优势比(odds ratio, OR)以及95%置信区间(confidence interval, CI),进而得到具有统计学意义的指标。将这些指标进行多因素Logistic回归分析,继而得出胃癌周围神经侵犯的独立危险因素,此外应用Hosmer-Lemeshow拟合优度检验拟合效果。根据独立危险因素可以建立胃癌周围神经侵犯的诊断预测模型,同时应用受试者工作特征(receiver operator characteristic, ROC)曲线和单因素Logistic回归分析验证该模型的诊断价值。P < 0.05表示差异具有统计学意义。
3. 结果
3.1. 543例胃癌患者周围神经侵犯的单因素Logistic回归分析
543例胃癌患者周围神经侵犯的单因素Logistic回归分析结果见表1。体重、BMI、淋巴细胞、单核细胞、红细胞、血红蛋白、血小板、NLR、PLR、LMR、白蛋白、CEA、CA19-9、CA72-4、肿瘤最大直径、肿瘤分化程度、肿瘤组织类型、肿瘤浸润深度、淋巴结转移以及癌结节是具有统计学意义的临床病理指标,而性别、年龄、身高、白细胞、中性粒细胞、白球比、ABO血型以及肿瘤位置在统计学上无明显差异,与胃癌周围神经侵犯无关。
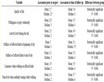
Table 1. The results of univariate Logistic regression analysis of perineural invasion in gastric cancer
表1. 胃癌周围神经侵犯的单因素Logistic回归分析结果
3.2. 543例胃癌患者周围神经侵犯的多因素Logistic回归分析
将单因素Logistic回归分析结果中具有统计学意义的临床病理指标进行多因素Logistic回归分析,结果见表2。结果提示,红细胞、血红蛋白、CA19-9、CA72-4、肿瘤最大直径、肿瘤分化程度、肿瘤浸润深度、淋巴结转移以及癌结节是具有统计学意义的临床病理指标,而体重、BMI、淋巴细胞、单核细胞、血小板、NLR、PLR、LMR、白蛋白、CEA以及肿瘤组织类型在统计学上无明显差异,与胃癌周围神经侵犯无关。经筛选后再次进行多因素Logistic回归分析可知,肿瘤最大直径(P = 0.010, OR: 1.226, 95% CI: 1.049~1.433)、肿瘤分化程度(P < 0.001, OR: 3.000, 95% CI: 1.660~5.423)、肿瘤浸润深度(P < 0.001, OR: 6.323, 95% CI: 2.766~14.453)、淋巴结转移(P < 0.001, OR: 42.884, 95% CI: 11.470~160.338)以及癌结节(P < 0.001, OR: 17.454, 95% CI: 6.759~45.071)是胃癌周围神经侵犯的独立危险因素,结果见表3。此外应用Hosmer-Lemeshow拟合优度检验得知该五项临床病理指标拟合效果较好(χ2 = 7.581, P = 0.475)。
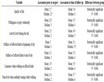
Table 2. The results of multivariate Logistic regression analysis of perineural invasion in gastric cancer
表2. 胃癌周围神经侵犯的多因素Logistic回归分析结果
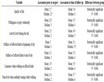
Table 3. The results of multivariate Logistic regression analysis of five clinicopathological indicators
表3. 五项临床病理指标的多因素Logistic回归分析结果
3.3. 诊断预测模型建立及其诊断价值分析
对肿瘤分化程度、肿瘤浸润深度、淋巴结转移以及癌结节四项分类变量进行赋值,结果见表4。依据表3和表4结果,可以建立胃癌周围神经侵犯的诊断预测模型为:P = ex/(1 + ex),x = −4.118 + 0.204 × 肿瘤最大直径 + 1.099 × 肿瘤分化程度 + 1.844 × 肿瘤浸润深度 + 3.759 × 淋巴结转移 + 2.860 × 癌结节。其中e为自然常数,约为2.71828。将543例胃癌患者根据诊断预测模型依次计算出其对应的P值,同时应用ROC曲线验证该模型的诊断价值(见图1)。曲线下面积为0.944,渐近95% CI为0.926~0.961。依据ROC曲线检验结果变量的敏感度和特异性,计算得出约登指数最大值为0.770,其对应的预测模型诊断临界值为0.5122,该临界值对应的敏感度为0.836,特异性为0.935。此外将543例胃癌患者周围神经侵犯预测结果进行二分类并赋值,即预测值大于或等于0.5122判断为周围神经侵犯阳性并赋值为1,小于0.5122判断为周围神经侵犯阴性并赋值为0,同时选用单因素Logistic回归分析检验该诊断模型的预测准确率,结果见表5、表6。该诊断模型的预测正确总体百分比87.1%以及单因素Logistic回归分析结果均提示该模型预测准确率较高。
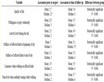
Table 4. The assignment results of four classification variables
表4. 四项分类变量的赋值结果
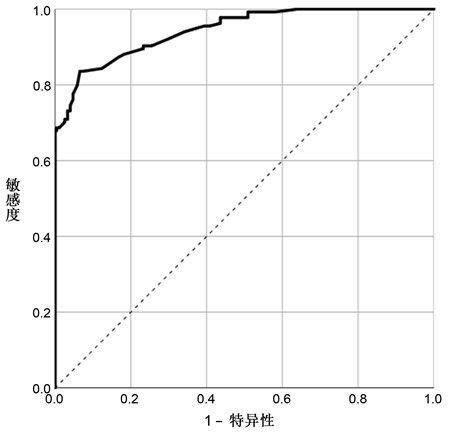
Figure 1. The ROC curve of the diagnostic prediction model for perineural invasion in gastric cancer
图1. 胃癌周围神经侵犯诊断预测模型的ROC曲线
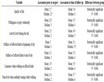
Table 5. The classification table of the diagnostic prediction model for perineural invasion in gastric cancer
表5. 胃癌周围神经侵犯诊断预测模型的分类表
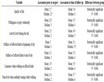
Table 6. The results of univariate Logistic regression analysis of the diagnostic prediction model for perineural invasion in gastric cancer
表6. 胃癌周围神经侵犯诊断预测模型的单因素Logistic回归分析结果
4. 讨论
周围神经侵犯是肿瘤侵犯神经并伴有肿瘤微环境改变的过程,已在不同类型的肿瘤中检测到,既往研究报道胃癌周围神经侵犯的发生率在6.8%至75.6%之间 [3] [6]。本研究纳入的543例胃癌患者其周围神经侵犯发生率为49.36% (268/543)。随着近年来国内外研究的不断深入,越来越多的证据揭示了周围神经侵犯是癌细胞局部扩散的一个重要途径,代表着肿瘤具有侵袭性的生物学行为 [7] [8]。Deng等 [9] 的研究指出,周围神经侵犯是影响胃癌患者根治性切除术后总生存期和无病生存期的独立预后因素。此外Chen等 [10] 的研究也阐述了类似的观点。因此建立诊断预测模型对胃癌周围神经侵犯的发生风险进行评估,从而提早采取及时有效的干预措施以改善患者预后,就显得尤为必要。
Del Rio等 [11] 的研究提出,在胃癌患者中,肿瘤大小可以提示肿瘤的恶性程度;同时肿瘤大小是一个重要的生存预测指标,与总生存期显著相关;此外肿瘤大小与浸润深度和淋巴结转移有关,应作为独立的预后因素。同时Zhao等 [12] 的研究表明,肿瘤大小是淋巴结阴性胃癌患者的独立预后因素,考虑肿瘤大小的影响可以提高淋巴结阴性胃癌患者预后预测的准确性。本研究的结果与上述一致,多因素Logistic回归分析结果提示肿瘤最大直径(P = 0.010, OR: 1.226, 95% CI: 1.049~1.433)是胃癌周围神经侵犯的独立危险因素,反映出肿瘤大小在胃癌周围神经侵犯的发生发展进程中发挥着重要作用。Feng等 [13] 的研究发现胃癌的分化程度与年龄、性别、肿瘤位置、肿瘤大小、肿瘤深度、淋巴结转移以及肿瘤分期显著相关。此外崔伟豪等 [14] 的研究显示,肿瘤分化程度是发生胃癌脉管癌栓的独立影响因素。本研究的结果表明肿瘤分化程度(P < 0.001, OR: 3.000, 95% CI: 1.660~5.423)是胃癌周围神经侵犯的独立危险因素,低分化的胃癌患者发生周围神经侵犯的可能性显著高于高分化和中分化的胃癌患者。术前判断肿瘤浸润深度对于确定胃癌患者最佳治疗方法至关重要,对于黏膜内癌和黏膜下浸润较浅的胃癌,内镜黏膜下剥离术通常可以达到根治性切除,而对于浸润较深的胃癌则需要手术切除 [15]。本研究的结果说明肿瘤浸润深度(P < 0.001, OR: 6.323, 95% CI: 2.766~14.453)是胃癌周围神经侵犯的独立危险因素,肿瘤浆膜浸润(T3 + T4)的胃癌患者发生周围神经侵犯的可能性显著高于肿瘤浸润深度为T1 + T2的胃癌患者。除了肿瘤浸润深度,可见于胃癌病程各个阶段的淋巴结转移也是影响患者预后的重要因素之一 [16] [17]。本研究的结果显示胃癌患者存在淋巴结转移时,可以导致周围神经侵犯的发生率显著上升(P < 0.001, OR: 42.884, 95% CI: 11.470~160.338),这提示临床医生在面对胃癌患者时,要关注淋巴结转移对其发生周围神经侵犯的影响。癌结节来源于肿瘤的直接播散、淋巴结转移、脉管侵犯或神经侵犯等,随着肿瘤进展,原有结构被破坏,继而形成癌结节 [18]。Tan等 [19] 的研究指出,癌结节的存在显著降低了胃癌患者的总生存期和肿瘤特异性生存期。本研究的结果证实了有癌结节的胃癌患者发生周围神经侵犯的可能性显著高于无癌结节的胃癌患者(P < 0.001, OR: 17.454, 95% CI: 6.759~45.071)。此外鉴于TNM分期系统对胃癌患者的生存结局以及后续治疗具有重要的指导意义,Aurello等 [20] 建议胃癌传统的TNM分期系统应当纳入周围神经侵犯这项指标,以更准确地对胃癌患者进行病情评估和预后预测,有助于患者选择更广泛的手术方式和进一步的辅助治疗,从而在一定程度上提高患者生存率。本研究的结果也支持这项见解。
5. 结论
综上所述,本研究的结果表明,肿瘤最大直径、肿瘤分化程度、肿瘤浸润深度、淋巴结转移以及癌结节是胃癌周围神经侵犯的独立危险因素。根据独立危险因素可以建立胃癌周围神经侵犯的诊断预测模型为:P = ex/(1 + ex),x = −4.118 + 0.204 × 肿瘤最大直径 + 1.099 × 肿瘤分化程度 + 1.844 × 肿瘤浸润深度 + 3.759 × 淋巴结转移 + 2.860 × 癌结节。本研究建立的胃癌周围神经侵犯诊断预测模型其敏感度(0.836)和特异性(0.935)均较高,且预测准确率(87.1%)也较高,提示该预测模型的诊断价值良好,具有较好的实践应用价值,可以为胃癌患者个体化和综合治疗方案的制定提供基础,有助于延长胃癌患者的生存时间同时改善其生活质量。本研究采用回顾性研究方法统计分析相关数据,样本范围仅为延安大学附属医院收治的胃癌患者,这些因素可能会对研究结果产生一定的影响。
基金项目
陕西省重点研发计划项目(项目编号:2020SF-237);延安大学研究生教育创新计划项目(项目编号:YCX2020020)。
参考文献
NOTES
*第一作者。
#通讯作者。