1. 引言
1973年,Bismut [1] 首次引入了线性倒向随机微分方程的概念,并研究了该类方程解的存在唯一性。基于如下的BSDE,
Pardoux和Peng [2] 得到了非线性倒向随机微分方程解的存在唯一性。这一重要研究成果奠定了正倒向随机微分方程组的理论基础。从那时起,BSDE得到了许多研究者的广泛研究。在之后的研究中,通过非线性Feynman-Kac公式,Ma,Protter和Yong [3] 提出了研究正倒向随机微分方程组的四步法,该方法证明了在任意时间区间上的解的存在唯一性。1991年,Peng [4] 得到了正倒向随机微分方程(FBSDEs)和偏微分方程之间的直接关系,然后在 [5] 中,他还找到了随机控制问题的最大值原理。
虽然BSDE的解析解往往难以获得,但BSDE的近似数值解相对容易计算,因此在实际应用中,求解近似数值解成为一种非常理想的方法。求解BSDE的数值方法主要有两类。一种是基于BSDE与其相应的抛物型偏微分方程之间的关系,Ma [3] 等利用这种关系,首次提出了一种典型的研究FBSDEs的四步方法(而不是数值方法)。基于在四步法中提出的类似思想,后续又有学者提出了一些数值求解的算法。另一种方法是直接基于BSDE设计的,当函数f不依赖于变量
时,Chevance [6] 提出了一种有效的时空离散化方案。Bender [7] 等引入了一种求解BSDEs的正演方案。Peng [8] 提出了一种在合理假设下收敛的迭代线性近似算法。Memin等在 [9] 中提出了一些求解BSDEs的随机游走方法,并证明了这些方法的收敛性。在某些较弱的正则性假设下,Zhang提出了一种改进的求解BSDEs的数值方案,并在 [10] 中研究了其收敛速度,然后Cvitanic等在 [11] 中提出了一些求解FBSDEs的Monte Carlo方法。Zhao等在 [12] 中提出了一种高精度求解BSDEs的数值方法,Zhao等在 [13] 中研究了
格式的误差估计。2014年,Zhao等在 [14] 中从BSDEs中推导出两个参考ODE,然后逼近参考ODE中的条件期望和导数,得到了带导数的数值格式。同年,Chassagneux在 [15] 中,提出了BSDEs的线性多步方法。Chassagneux等在 [16] 中,提出了BSDEs的Runge-Kutta方法。2017年,Tang等在 [17] 中提出了BSDEs中的延迟修正方法,其关键特点之一是迭代使用低阶数值方法,从而得到高阶数值格式。2019年,Zhang等在 [18] 中导出了一类单步多导数方法。通过几个数值例子说明了该方法的计算效率和高阶精度。为了说明该方法的优点,并与
方法进行了比较。2021年,Zhou等在 [19] 中结合Feynman-Kac公式配合Itô-Taylor展开,在数值求解
时使用有限差分,提出了一类BSDEs的高阶一步格式。
目前国内外学者一直致力于对BSDEs数值解的研究,想要寻求具有较高收敛阶的数值方法。2010年,Zhao等在 [20] 中利用时空网格提出了一种稳定的多步方案。Zhao等证明了所提出的半离散格式的收敛性可以是高阶的,而精确的阶数取决于所使用的时间网格点。本文拟在Zhao的基础上采用Chebyshev网格进行空间离散,使用重心Lagrange插值 [21] 近似非网格点的插值,最后,我们在证明过程中考虑了Newton-Cotes系数的代数精度,并给出了多步格式收敛阶的证明。
2. 多步半离散格式
令
是一个完整的、滤波的概率空间,在这上面定义了一个标准的d维布朗运动
。如果一个过程
它是
-适应,平方可积而且满足(1),
(1)
我们称这个过程为(1)的
-适应解。其中
是关于每一个
适应的随机过程,右边的第三项是一个Itô型积分。
Pardoux和Peng证明了(1)的解的存在唯一性,我们假设初值
形式为
,然后 的解
能够表示为如下形式,
其中,
表示
相对于空间变量x的梯度,而
是以下抛物型偏微分方程的解:
考虑情况
,对方程(1)的区间
进行均匀网格划分,N为一个正常数,有
,
和时间步长
,对于两个给定的正常数k和
且满足
,考虑
(2)
在(2)的等式两端同取期望
,我们得到
(3)
在等式(3)两边同乘以
,再取条件数学期望,根据Itô等距公式,
注意到
是关于s的确定性函数,因此我们选择用Lagrange插值基于插值点
去近似这个确定函数,我们有
其中
当时间为等距划分时,我们有
(4)
其中
即
(5)
通过上面的推导我们得到关于
的多步半离散格式为
(6)
类似可以得到关于
的多步半离散格式为
(7)
其中
这里的推导过程我们用到了等式(8)
(8)
3. 最优收敛阶证明
在本小节中,我们将对多步半离散方案进行误差分析,即生成器函数f独立于随机变量
,对于方程,
(9)
为了简单的分析,我们只考虑
的情况,但下面得到的所有结果也适用于一般的情况。考虑如下的简化形式,
(10)
相应的我们的多步方案为:
(11)
其中
。
对于后续的证明,这里我们用到了Zhao [20] 中的假设1,假设2,引理1,引理3。对于Zhao [20] 中的引理2,我们考虑Newton-Cotes系数的代数精度,进行了修改如下。
引理2 设
和
为参考方程(10)中定义的局部截断误差。然后在假设2下,我们有以下的局部估计:
,
为奇数时;
,
为偶数时;
。
其中
是一个一般常数,只依赖于T,
的上界和f及其它们的导数。
上述引理的证明与 [13] 中的引理3.2的证明相似,因此我们在这里省略了它。但考虑到多步格式(11)中y的求解方程系数为Newton-Cotes系数,结合Lagrange插值的局部截断误差和Newton-Cotes系数代数精度我们可以得到以上
的结论。
现在我们在下面的定理中给出了
的误差估计:
定理1 设
和
分别为BSDE和多步格式的解。在假设2成立的基础上,并且初始值满足
。然后对于足够小的时间步长
,我们有
,
为奇数时;
,
为偶数时;
其中
是一个一般常数,依赖于T,
的上界和f及其它们的导数。
证明:令
对于
。从(10)和(11)我们得到
(12)
然后我们有
(13)
其中L是函数
相对于
的Lipschitz常数。对不等式(13)两边同取数学期望
,我们得到
(14)
令
。对于满足
的整数s,我们也有同样的估计
(15)
然后我们将上面不等式(15)按照
相加可以得到
(16)
整理有
(17)
这里我们令
,
,以及
。
对于足够小的时间步长
,D和
明显是正数,并由一个正的常数为界。然后通过引理3和上式,得到下面的不等式:
通过引理2,我们可以知道
,
为奇数时;
,
为偶数时,
即
,
为奇数时;
,
为偶数时。
在定理的初始条件
下,我们可以得到结论
,
为奇数时;
,
为偶数时。
综上,得证。
我们在下面的定理中给出
的误差估计。
定理2 设
和
分别为BSDE和多步格式的解。在假设2成立的基础上,并且初始值满足
。然后对于足够小的时间步长
,我们有
其中
是一个一般常数,依赖于T,
的上界和f及其它们的导数。
对于
的误差估计证明见Zhao [20] 中定理2的证明。
4. 数值实验
为数值求解
,我们也需要空间离散,这里我们选择Chebyshev网格点。为此,我们在时间层
上引进
空间上的一个剖分
。用
表示剖分
中网格点的密度:
同理,推导全离散下
的格式为
期望的近似采用Gauss-Hermite求积公式,有如下等式
我们进行了一些数值实验,用来说明方案的准确性和有效性。为了方便起见,我们将在时间网格上使用均匀划分,在空间离散上使用Chebyshev非均匀网格进行空间离散化。Chebyshev非均匀网格可以有效地避免Runge现象使空间离散插值近似的更加准确。在我们的数值实验中,我们设定
。误差
和
通常来自三个方面:时间离散化,空间插值和用Gauss-Hermite求积公式对条件期望进行近似。我们的主要目标是测试对于时间离散的收敛速度,所以需要尽量平衡其它误差。我们设定Gauss-Hermite正交点的数量为
用来尽可能减小Gauss-Hermite求积公式带来的误差影响。我们采用重心Lagrange插值来尽可能减小空间离散带来的误差。实验中,我们选用高阶的单步方法或者直接选取精确值作为启动值,利用重心Lagrange插值来获得启动值对应的空间层的非网格点的近似值,应用到多步全离散格式中,循环以上步骤,从而获得数值解
。通过对数值误差进行线性最小二乘拟合,得到了对时间步长
的收敛速度(CR)。
例1考虑如下的线性BSDE
其解析解可以表示为
我们使用多步全离散格式来解决例1中的线性BSDE。在表1和表2中,我们分别列出了
和
的数值误差,以及其收敛速度。
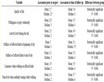
Table 1. Error and convergence rate of | y 0 − y 0 | in Example 1
表1. 例1中
的误差和收敛速度
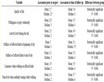
Table 2. Error and convergence rate of | z 0 − z 0 | in Example 1
表2. 例1中
的误差和收敛速度
我们设定终端时间为
。那么精确解
在
时,
是
。此外,我们将时间层划分设定为
。从表1和表2的数值结果中,我们可以得出结论,多步全离散格式对于求解BSDE是稳定和准确的。此外,实验数据表明,当
为奇数时,y的收敛阶可以达到
,当
为偶数时,y的收敛阶可以达到
,对于z,收敛阶可以达到
。
例2考虑如下的线性BSDE
其解析解可以表示为
此例子中,我们设定终端时间为
。精确解在
时为
。误差
和
分别在表3和表4中给出,并且给出了相应的收敛速率。表中数据表明,y的收敛阶与我们定理1的理论推导一致,z的收敛阶符合定理2给出的结论。
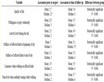
Table 3. Error and convergence rate of | y 0 − y 0 | in Example 2
表3. 例2中
的误差和收敛速度
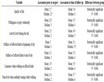
Table 4. Error and convergence rate of | z 0 − z 0 | in Example 2
表4. 例2中
的误差和收敛速度
5. 结论
本文介绍了一类求解倒向随机微分方程的时空网格上的多步格式。我们在时间上采用Lagrange插值离散积分,在空间上采用Chebyshev网格和重心Lagrange插值,此外,我们还采用了Gauss-Hermite求积公式来求解近似条件数学期望。我们在生成元函数f与随机变量
无关时,给出了多步半离散格式的最优收敛阶估计。最后,我们通过一些数值实验验证了该多步格式的最优收敛阶:当
为奇数时,y的收敛阶为
,当
为偶数时,y的收敛阶为
,z的收敛可以达到
。
基金项目
国家自然科学基金项目(11901133)。
NOTES
*通讯作者Email: 954982638@qq.com