1. 背景
类风湿关节炎(Rheumatoid arthritis, RA)是一种以慢性炎症、持续性滑膜炎和滑膜关节破坏为特征的系统性自身免疫性疾病 [1]。它是最常见的炎性关节炎,具有高的发病率和致残率 [2]。RA在女性中的患病率高于男性。RA的病因和发病机制复杂,涉及滑膜细胞增殖和纤维化、血管翳形成,以及软骨和骨侵蚀 [3]。除了遗传和家族性危险因素外,还存在多种环境、饮食和生活方式因素与RA发病相关 [4]。RA是不同发病机制的结果,并表现出不同的临床表现和细胞基础和分子基础。炎症细胞因子,如白细胞介素(IL)-6、IL-1β和肿瘤坏死因子(TNF)-α,已被证实在RA的发病机制中发挥作用。然而,类风湿关节炎的具体病理生理机制尚未被彻底研究。因此,探索RA发生、发展的分子特征和机制,为RA的有效预防、诊断和治疗提供新的策略具有重要意义 [5]。
基于高通量平台的基因芯片技术已被广泛用于基因组水平上探索和识别有希望的疾病诊断和预后生物标志物。大量研究表明,RA发生的病理生理过程与基因突变和异常表达密切相关,包括HLA II类基因、PTPN22、CTLA4、PADI4等 [6] [7] [8] [9]。在非HLA-DRB1基因座中,PTPN22基因是迄今为止与RA易感性最显著相关的基因,与HLA-DRB1等位基因一起,被认为是RA 50%的遗传组成部分 [10]。最近的一项研究表明,病毒和细菌可以通过与TLR结合增加对RA的易感性并加剧RA。促进破骨细胞成熟由toll样受体(TLR)7/8信号介导,该信号由mir-574-5p结合激活 [11]。一项研究表明,RA患者的外周血单核细胞和血清外泌体中lncRNAs Hotair和NEAT1的高表达水平,有助于活性巨噬细胞的迁移 [12]。因此,为了对RA的诊断和治疗做出新突破,有必要探索RA发生和进展中包含的准确分子靶点。
我们分析了美国国立生物技术信息中心(National Center for Biotechnology Information, NCBI)的GEO (Gene Expression Omnibus)数据库的mRNA微阵列数据,以筛选RA和健康样本之间的差异表达基因(DEGs)。然后,通过对DEGs的GO和KEGG的富集分析,进一步探讨了RA的分子机制。IGLJ3、IGHM、IGKC、IGLV1-44、GUSBP11基因是与RA发生发展密切相关的基因。通过R语言CIBERSORT包进行免疫浸润评估。用WGCNA和GSEA分析IGLJ3、IGHM、IGKC、IGLV1-44、GUSBP11可能影响RA发病的机制。R软件(版本3.6.1;https://www.r-proje-ct.org/)和Cytoscape等用于数据处理。这项研究中发现的类风湿关节炎和健康对照之间的新基因和通路有望帮助揭示潜在的分子。
2. 材料和方法
2.1. 获取基因数据集
以类风湿性关节炎为关键词,在GEO数据库(https://www.ncbi.nlm.nih.gov/geo)中搜索符合要求的基因芯片数据集GSE55235,数据集包括正常对照组和类风湿性关节炎组。利用平台的注释信息将探针转化为同源基因符号。
2.2. 数据校正和差异表达基因筛选
基于R软件(版本4.0.3)的limma和impute包,使用稳健多阵列平均算法(RMA) [13] 对从GEO数据库下载的原始文件进行预处理和标准化。使用limma软件包进行样本间差异基因分析,获得p值后进行多重假设检验和校正。筛选标准为log2 (fold change)>1或<−1,校正后的p值(Q value) < 0.05。用ggplot软件包制作火山图显示差异基因的分布情况。
2.3. GO和KEGG富集分析
为了对获得的DEGs进行功能性注释,使用R软件进行基因本体论(Gene Ontology, GO)和京都基因与基因组百科全书(Kyoto Encyclopedia of Genes and Gnomes, KEGG)的注释和可视化。R软件clusterProfler、enrichplot、ggplot2、Circize、RColorBrewer、dplyr、ComplexHeatmap软件包用于分析DEGs的GO和KEGG富集,并使用chord plot软件包以可视化富集结果。
2.4. GSEA富集分析
基因集富集分析(Gene Set Enrichment Analysis, GSEA)用于评估基因集中一组预定义基因的分布趋势,以确定它们对表型的贡献。GSEA 4.1.0版软件用于分析基因功能。我们下载了GSEA_4.1.0和GO基因集(c5.all.v7.5.1.symbols.gmt)、KEGG基因集(c2.cp.KEGG.v7.5.1.symbols.gmt)用于功能富集分析。富集分析采用默认加权富集法。随机组合设定为1000次。使用GSEA分析对DEG进行GO和KEGG途径富集分析。FDR < 0.25,NOM p值 < 0.05和|NES| > 1被认为是显著富集 [14]。
2.5. 构建加权基因共表达网络及蛋白互作网络
利用R软件中的WGCNA软件包构建了基于DEGs的加权基因共表达网络(Weighted gene co-expression network analysis, WGCNA) [15]。在本研究中,我们构建了一个邻接矩阵来描述基因之间的相关强度(软阈值参数)。随后,我们将邻接矩阵转化为拓扑重叠矩阵(Topological overlap matrix, TOM)来测量基因的网络连通性。接下来,我们执行分层聚类来识别模块,模块由分支和聚类树的不同颜色表示。最后,我们计算特征基因,对模块进行层次聚类,构建模块关系,计算基因模块与表型之间的相关性,识别与临床特征相关的模块。构建蛋白互作网络(Protein protein interaction network, PPI) (筛选阈值 = 0.5),然后利用Cytoscape软件(https://www.cytoscape.org/)的Cytohubba插件 [16],综合12种算法排序得出Top5的基因。
2.6. 临床预测模型建立
通过R软件的“limma”软件包获得5个关键基因的表达量,使用“RMS”软件包,建立列线图模型来预测类风湿关节炎的发生。然后利用校准曲线评价诺模图模型的预测能力。最后通过决策曲线分析评价模型的临床价值。
2.7. 免疫细胞浸润分析
我们使用R软件“e1071”和“parallel”软件包来评估免疫浸润的丰度,我们从CIBERSORT下载了22种浸润性免疫细胞的数据(https://cibersort.stanford.edu/).我们使用R软件的“corrplot”软件包绘制相关热图,可视化22种浸润性免疫细胞的相关性,“ggplot2”软件包对免疫细胞浸润矩阵数据进行PCA聚类分析,绘制二维PCA聚类图,并绘制小提琴图,以可视化免疫细胞浸润的差异。
3. 结果
3.1. 数据处理和DEGs筛选结果
基于高通量分析,对原始数据进行校正和标准化处理,如(图1(A)、图1(B))所示。运用“limma”软件包,以log2 (fold change) >1或<−1,校正后的p值(Q value) < 0.05为筛选阈值,在RA与正常对照组间筛选出1071个DEGs,包括599个上调和472个下调基因。结果以火山图和热图可视化(图1(C)、图1(D))。
(A)
(B)
(C)
(D)
Figure 1. (A) Raw unprocessed dataset; (B) Data set after correction and standardization; (C) Differential gene volcano map between RA and control groups; (D) Differential gene heat map between RA and control groups
图1. (A) 原始未处理数据集;(B) 经校正和标准化处理后的数据集;(C) RA和对照组的差异基因火山图;(D) RA和对照组的差异基因热图
3.2. GO和KEGG富集分析
GO富集分析用于从分子功能、生物过程和细胞成分评估上述获得的差异基因的潜在机制。GO富集结果表明,这些基因在生物过程模块上与T淋巴细胞活化、细胞活性的正调控、细胞黏附等有关。细胞组成模块富集表明这些基因发挥作用的位置可能位于质膜外侧、细胞外基质等。分子功能模块富集显示基因产物分子的功能主要集中于活化信号受体激活剂、受体–配体活化、活化细胞因子及其受体等(图2(A)、图2(B))。KEGG途径分析表明,相关基因参与细胞因子–细胞因子受体相互作用、趋化因子信号通路、病毒蛋白与细胞因子及细胞因子受体的相互作用等(图2(C)、图2(D))。
(A)
(B)
(C)
(D)
Figure 2. (A) Bar graph of go enrichment analysis of differential genes; (B) Cycle diagram of go enrichment analysis of differential genes; (C) Bar graph of KEGG enrichment analysis of differential genes; (D) Circle diagram of KEGG enrichment analysis of differential genes
图2. (A) 差异基因GO富集分析条形图;(B) 差异基因GO富集分析圈图;(C) 差异基因KEGG富集分析条形图;(D) 差异基因KEGG富集分析圈图
3.3. GSEA富集分析
我们使用GSEA软件对数据集进行基因富集分析,结果显示富集主要集中于:活化细胞参与免疫反应、B淋巴细胞活化、B淋巴细胞受体信号通路、自然杀伤细胞介导的细胞毒性反应、T淋巴细胞受体信号通路、趋化因子信号通路等(图3(A)~(F))。综合结果表明,B淋巴细胞、T淋巴细胞、自然杀伤细胞水平与RA的生物学途径密切相关。
3.4. WGCNA分析及PPI网络构建
我们选择之前筛选的差异基因(少于5000)进行WGCNA分析。拓扑重叠热图显示蓝色、蓝绿色、灰色模块重叠显著,表明该模块的WGCNA分析效果好结果较准确(图4(A))。模块–临床相关性热图显示灰色、蓝色、蓝绿色模块与RA的发生最为正相关,绿色、红色模块与RA的发生最为负相关(图4(B))。基因–临床相关分析显示出排名前10的差异基因与RA发生的相关性,如表1所示。使用WGCNA分析结果进行基因–基因互作网络预测,将数据导入Cytoscape构建蛋白互作网络(图4(C)),使用cytoHubba插件综合MCC、DMNC、MNC、Degree、EPC等12种算法,获得排名前5的关键基因包括IGLJ3、IGHM、IGKC、IGLV1-44、GUSBP11 (图4(D))。
(A)
(B)
(C)
(D)
Figure 4. (A) The topology overlapping heat map shows that the blue, blue-green and gray modules overlap significantly, indicating that the analysis results of this module are more accurate; (B) Heat map of gene and module correlation for WGCNA enrichment analysis. Each color represents a common expression module. The value in the module is the correlation coefficient, and the value in brackets is the p value; (C) The gene interaction network was constructed by using the differential genes further screened by WGCNA. The color of genes represents that the genes belong to different modules of WGCNA analysis; (D) The main difference genes obtained by using the cytohubba plug-in, and the top 5 key genes are marked in orange
图4. (A) 拓扑重叠热图显示蓝色、蓝绿色、灰色模块重叠显著,表明该模块的分析结果较准确;(B) WGCNA富集分析的基因与模块相关性热图。每种颜色代表一个共表达模块,模块内的数值为相关性系数,括号内的为P值;(C) 利用WGCNA进一步筛选的差异基因构建的基因互作网络,基因的颜色代表基因属于WGCNA分析的不同模块;(D) 使用cytohubba插件获得的主要差异基因,排名前5的关键基因使用橘黄色标注
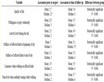
Table 1. Results of correlation analysis between main differential genes and RA
表1. 主要差异基因与RA相关性分析结果
3.5. 临床预测模型建立
我们使用R软件的“RMS”包建立了RA诊断列线图模型(图5(A))。然后,运用校准曲线评估列线图的预测能力。结果表明,实际RA风险与预测风险之间的误差很小,表明列线图模型具有较高预测RA的准确性(图5(B))。决策曲线(Decision Curve Analysis, DCA)显示红色曲线高于灰色曲线,在阈值0.14到0.76处患者净获益最大,且C-index为0.732说明模型准确性高(图5(C))。
(A)
(B)
(C)
Figure 5. (A) Nomogram for predicting RA occurrence based on differential gene construction; (B) Calibration curve to evaluate the accuracy of nomograph model; (C) The decision curve (DCA) shows that the red curve is higher than the gray curve. When the threshold is 0.14 to 0.76, the net benefit of patients is the largest, and the C-index is 0.732, which proves that the model is very accurate
图5. (A) 基于差异基因构建的预测RA发生的列线图;(B) 校准曲线评估列线图模型的准确性;(C) 决策曲线(DCA)表明,红色曲线高于灰色曲线,在阈值为0.14至0.76时,患者的净收益最大,且C-index为0.732证明该模型非常准确
3.6. 免疫细胞浸润分析
免疫细胞浸润的小提琴图显示,与正常对照样品相比,RA患者中的浆细胞(p = 0.001)和CD8阳性T细胞(p = 0.008)浸润更多,活性肥大细胞(p = 0.002)和CD4阳性的静息记忆T细胞(p = 0.001)浸润更少(图6(A))。22种免疫细胞的相关热图显示,单核细胞与调节性T细胞呈正相关,浆细胞与CD4阳性的静息记忆T细胞呈负相关(图6(B))。主成分分析(PCA)显示,RA患者和正常对照组样本中的免疫细胞比例存在个体差异(图6(C))。
(A)
(B)
(C)
Figure 6. (A) The violin diagram shows the proportion of 22 immune cells in the data set. Plasma cells and CD8 positive T cells were more infiltrated in the data set, while active mast cells and CD4 positive resting memory T cells were less infiltrated; (B) Heat maps of 22 immune cells. The number of colored squares indicates the strength of the correlation. Red represents positive correlation and blue represents negative correlation. The darker the color, the stronger the correlation; (C) Principal component analysis of all samples in the data set showed that there were differences between immunophenotypes
图6. (A) 小提琴图显示了数据集22种免疫细胞的比例。数据集中的浆细胞和CD8阳性T细胞浸润较多,活性肥大细胞和CD4阳性的静息记忆T细胞浸润较少;(B) 22种免疫细胞的相关热图。彩色方块的数量表示相关性的强度。红色代表正相关,蓝色代表负相关。颜色越深,相关性越强;(C) 对数据集的所有样本进行主成分分析,结果表明免疫表型之间存在差异
4. 讨论
RA是最常见的慢性炎性关节疾病之一,常常由于炎症失控而导致残疾 [17]。因此,迫切需要更好地掌握RA诊断和治疗新策略的详细机制 [18] [19]。在这项研究中,基于从GEO数据库数据集获得的基因表达谱,通过一系列生物信息学分析确定了5种最具差异的基因(IGLJ3、IGHM、IGKC、IGLV1-44、GUSBP11)。随后,我们对这些差异表达基因的生物学功能进行了进一步的研究 ,GO分析显示这些DEGs与免疫功能的变化显著相关。GO、KEGG富集分析和GSEA富集分析均表明,B淋巴细胞通路、T淋巴细胞通路、趋化因子信号通路是RA的关键通路,这与之前的研究一致 [20] [21] [22] [23]。这些DEGs有助于促进RA的诊断和治疗,为了解RA的研究指明了新的方向。为了更好地了解RA的进展,使用WGCNA来识别RA的候选生物标志物。最后,通过WGCNA分析构建了一些RA相关模块。综合各个模块分析及与患者诊断的相关性,我们确定了IGLJ3、IGHM、IGKC、IGLV1-44、GUSBP11基因在RA疾病发生发展中具有重要作用。
免疫球蛋白在类风湿关节炎的发生发展及诊断治疗中一直有着重要作用,类风湿因子(RF)是一种结合IgG Fc段的自身抗体,是自身免疫在RA种发挥作用最早的直接证据。与血清RF阴性的RA患者相比,血清RF阳性的RA患者有更严重的临床表现和并发症 [24]。之前的研究发现,RA中的RF主要由IgG重链可变区3 (IGVH3)基因和一组轻链可变区(VL)基因编码,而具有RF活性的天然抗体则由IGVH1、IGVH4和IGKV3基因编码 [25]。最近的一项研究发现位于RA患者上经4-羟基-2-壬烯醛修饰免疫球蛋白KappaC (IGKC)轻链构成的IgG和IgM较正常对照组增多,结果表明高水平的IGKC可能会增加患RA的风险 [26]。我们的研究新发现IGLJ3、IGHM、IGKC、IGLV1-44四种免疫球蛋白基因在RA的发生发展中起到了重要作用,为进一步探究RA的发生机制及诊断治疗提供了新的靶点。
此外,由研究表明,免疫细胞浸润在RA的发病过程中起重要作用。因此,寻找特异性诊断标志物,分析RA的免疫细胞浸润,对改善RA患者的临床症状和预后有深远意义。我们使用R软件的CIBERSORT包对RA的免疫浸润进行了综合分析。我们发现CD8阳性T淋巴细胞和浆细胞浸润增加。CD4阳性的静息记忆T淋巴细胞和活性肥大细胞浸润减少可能与RA的严重程度显著相关。亚索等人发现记忆性CXCR4阳性CD4阳性的T淋巴细胞与RA的严重程度显著相关 [27]。单核细胞与调节性T细胞呈正相关,提示二者在RA的发生发展中具有正向协同作用。浆细胞与CD4阳性的静息记忆T细胞呈负相关,提示二者在RA疾病过程中具有负向协同作用。在RA患者中,B淋巴细胞分化成浆细胞,产生类风湿因子和抗环瓜氨酸肽抗体 [28]。临床上利妥昔单抗通过消耗B淋巴细胞来减轻使用肿瘤坏死因子抑制剂不能控制病情的RA患者。可见,这些免疫细胞浸润机制与RA发病相关,为进一步探索RA的免疫浸润及临床诊断治疗提供了新的研究思路。
然而本研究尚有不足之处。一是纳入的基因芯片样本量有限,容易造成误差,需进行一定规模的体外实验以验证所得的关键基因;二是CIBERSORT算法是基于有限的遗传数据,导致免疫浸润评估时出现计算偏倚,仍需进一步实验验证。今后课题组的相关工作将基于生物样本进行实验研究,以验证本研究结果的准确性,并进一步探讨关键基因和免疫浸润之间的调控关系。
5. 结论
综上所述,根据我们的分析结果及文献证据表明,IGLJ3、IGHM、IGKC、IGLV1-44、GUSBP11基因在RA的发生发展中具有重要作用,应成为进一步研究的重点。这将有助于确定RA的新型诊断标志物和治疗靶点,也为后续的发病机制研究提供可靠的依据和全新的视角。
NOTES
*通讯作者Email: qyfuchb@163.com