1. 引言
阿尔茨海默病(Alzheimer’s Disease, AD)是一类与认知功能和行为障碍相关的进行性、不可逆的神经系统退行性病变,是65岁以上老年人痴呆的最常见原因 [1] ;有研究显示,截至2021年,我国AD患者已超过1000万名,成为全球AD患者最多的国家。同时,AD的发病率预计在未来几十年,将呈指数增长 [2] 。目前,AD病因尚未明确,缺乏标准诊断方法,相关药物的研发仍处于初步阶段,且难以根治,已演变成为全球主要公共卫生问题之一,严重影响了患者的生存质量,给患者家庭、医院和社会带来了沉重的经济负担,给我国乃至全球医疗保健系统带来了巨大的挑战,其病因、预防和治疗已成为神经科学的重点研究课题。
便秘是一种急性或慢性胃肠道疾病,以排便不频繁、粪便干硬、排便不完全和排便困难为主要特征。相关研究表明,大脑和肠道之间的双向连接,在肠道疾病和神经系统疾病之间的关联中起着至关重要的作用,肠道功能障碍可通过脑–肠轴影响中枢神经系统。研究发现,便秘与许多神经系统疾病密切相关,包括帕金森(PD)、多发性硬化症、脊髓损伤和AD [3] [4] [5] 等等。目前这些相关性研究主要集中在PD上,即使在4.3%~17.2%的AD患者中观察到便秘 [5] [6] ,但目前尚无研究将便秘与AD相关联。尽管随机对照试验作为流行病学研究中评估因果关系最可靠的方法,但受伦理和实验条件的限制,实施起来困难重重。孟德尔随机化(Mendelian Randomization, MR)研究是检验暴露与结果之间因果关系的统计方法。它使用单核苷酸多态性(SNP)作为工具变量(IV),并通过计算IV对暴露和结果的因果效应值间接推断暴露与结果之间的因果关系。与传统的观察性研究相比,它可以减少混杂因素或反向因果关系造成的对因果推断的影响 [7] 。
此次研究采用了两样本MR分析方法来探索阿尔兹海默症与便秘之间的因果关系,通过基因层面的分析揭示阿尔兹海默症发生和进展的机制,为加强防治措施提供新的依据。
2. 材料与方法
2.1. 数据来源
AD的遗传数据来自于Douglas Wightman等 [8] 关于AD的GWAS数据,总样本量1,126,563例,包括90,338例患和1,036,225有欧洲血统的受试者。数据从Complex Trait Genetics lab网站 (https://ctg.cncr.nl/software/summary_statistics)下载。便秘的GWAS汇总统计数据来自FinnGen研究最新第9版生物样本库,总样本量309,154例,包括26,919例病例和有欧洲血统的受试者,数据可从(https://www.finngen.fi/en)下载;由于本研究是基于公开数据,不需要额外的伦理批准或同意。
2.2. 工具变量的选择
MR分析中以SNP作为工具变量应满足三个关键假设 [9] :1) SNP须与暴露因素紧密相关;2) SNP独立于混杂因素;3) SNP仅通过暴露因素与临床结局相关联。根据以上条件,首先我们获得了与暴露显著相关的SNP (P < 5 × 10−6);同时,与暴露显著相关SNP需满足r2 < 0.001、遗传距离为10,000 kb以避免连锁不平衡(linkage disequilibrium, LD)偏倚对分析结果产生影响;然后,我们将选取的SNPs的rs编号逐一在使用Pheno Scanner数据库中进行检验,消除潜在的混杂因素影响(可能会影响AD的高危因素和保护因素),并且应保证所选择的SNPs与AD不直接相关,删除与AD直接高度相关的SNPs,以此来满足二和第三个假设。排除不明确的SNP和具有不可推断的正向链的回文结构SNP。最后,通过计算F统计量以避免弱工具变量偏倚,所有工具变量的F统计量需大于10 [10] (计算公式为F = β2/SE2,β为等位基因效应值,SE为标准误),若SNP的F统计量 < 10,则表明该SNP存在弱工具变量偏倚的可能性,进而将其剔除,避免对结果造成影响 [11] 。
2.3. 统计学方法
采用R4.3.0及R包(Two Sample MR)进行数据统计分析。使用了5种常用的MR分析方法:随机效应逆方差加权法(Inverse-Variance Weighted, IVW)、MR-Egger回归与加权中位数法(Weight Median Estimator, WME),简单模式(simple mode法)和加权模式(weighted mode法)验证便秘与AD之间的因果关联。本研究使用逆方差加权法(IVW)作为主要分析方法确定因果关系 [12] 。该方法在所有SNPs有效的前提下,根据每个研究的样本大小和效应估计的方差进行加权平均,在没有异质性和水平多效性存在的情况下,这种方法可以提供最准确的分析结果 [13] [14] 。使用留一法(Leave-one-out)进行敏感性分析,以评价SNP对于因果关联估计结果的影响大小 [15] 。采用MR-Egger回归方法和MR-多效残差和离群值(Pleiotropy Residual Sum and Outlier, PRESSO)对多效性进行鉴定和校正。在本研究中,我们使用 Cochran’s Q检验评估了每次分析时工具变量之间的潜在异质性,若其P > 0.05则代表不存在显著的异质性问题。
3. 结果
3.1. 便秘对AD的因果效应
以便秘为暴露因素、AD为结局变量,总共筛选出16个与便秘相关的SNP,具有全基因组显著阈值(P < 5 × 10−6)。MR分析结果显示:IVW [OR = 1.00, 95% CI (0.977, 1.039), P = 0.629]、MR Egger [OR = 0.991, 95% CI (0.910, 1.081), P = 0.857]、加权中位数法[OR = 1.008, 95% CI (0.964, 1.044), P = 0.870]、简单模式[OR = 0.935, 95% CI (0.435, 2.009), P = 0.866]、加权模式[OR = 0.997, 95% CI (0.941, 1.058), P = 0.934],5种方法均提示便秘与AD发生风险之间没有因果关系(表1)。进行异质性分析IVW检验(Q = 9.288, P = 0.862)和MR-Egger检验(Q = 9.143, P = 0.822),均未观察到明显的异质性。水平多效性分析:MR-Egger回归分析结果显示无水平多态性的证据(截距P = 0.987);离群值分析:使用MR-PRESSO没有发现离群的SNP,确保了结果的准确性。leave-one-out法分析结果表明,没有单个SNP影响到总体MR估计。MR分析结果见表1、图1~3。

Figure 1. Scatter plot of the results of Mendelian randomization analysis of constipation on AD
图1. 便秘对AD孟德尔随机化分析结果散点图

Figure 2. Sensitivity analysis of constipation to AD leave-one-out method
图2. 便秘对AD leave-one-out法敏感性分析结果
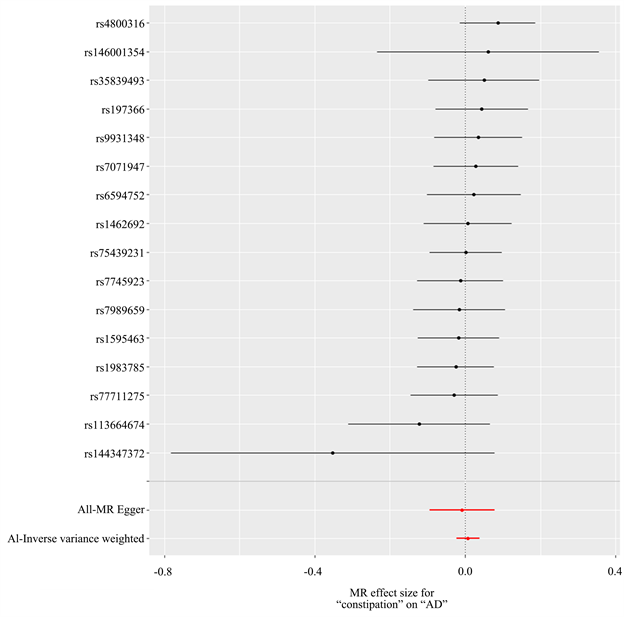
Figure 3. Forest map of Mendelian randomized analysis results of constipation on AD
图3. 便秘对AD孟德尔随机化分析结果森林图
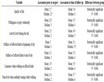
Table 1. Results of constipation on five MR test methods for AD
表1. 便秘对AD五种MR检验方法的结果
3.2. AD对便秘的因果效应
以AD为暴露因素、便秘为结局变量,总共筛选出56个与便秘相关的SNPs,具有全基因组显著阈值(P < 5 × 10−6)。MR分析结果显示:IVW [OR = 1.174, 95% CI (1.068, 1.290), P = 0.001]、MR Egger [OR = 1.173, 95% CI (1.031, 1.334), P = 0.018]、加权中位数法[OR = 1.134, 95% CI (1.004, 1.282), P = 0.043]、简单模式[OR = 1.171, 95% CI (0.835, 1.643), P = 0.364]、加权模式[OR = 1.160, 95% CI (1.030, 1.305), P = 0.017],提示便秘与AD发生风险之间正向因果关系。进行异质性分析IVW检验(Q = 61.009, P = 0.269)和MR-Egger检验(Q = 61.008, P = 0.239),未观察到明显的异质性。水平多效性分析:MR-Egger回归分析结果显示无水平多态性的证据(P = 0.708);离群值分析:使用MR-PRESSO没有发现离群的SNP,确保了结果的准确性。leave-one-out法分析结果表明,没有单个SNP影响到总体MR估计。MR分析结果见表2、图4~7。
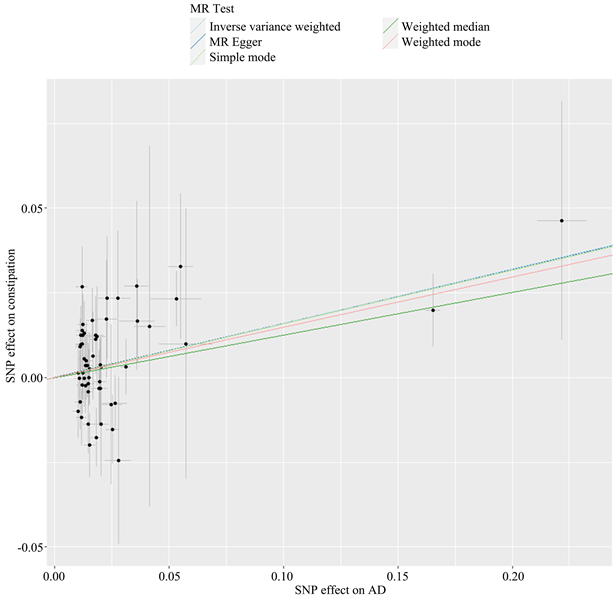
Figure 4. Scatter plot of Mendelian randomization analysis results of constipation by AD
图4. AD对便秘孟德尔随机化分析结果散点图
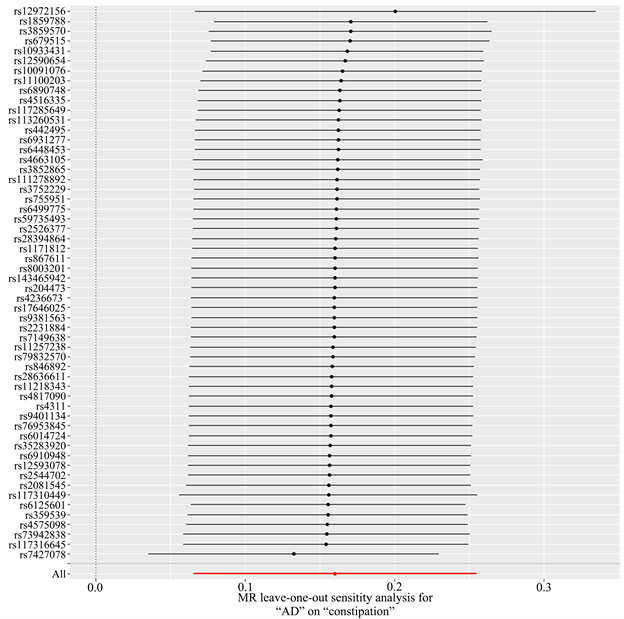
Figure 5. Sensitivity analysis of AD to constipation by leave-one-out method
图5. AD对便秘leave-one-out法敏感性分析结果
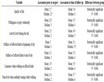
Table 2. Results of five MR test methods for constipation by AD
表2. AD对便秘五种MR检验方法的结果
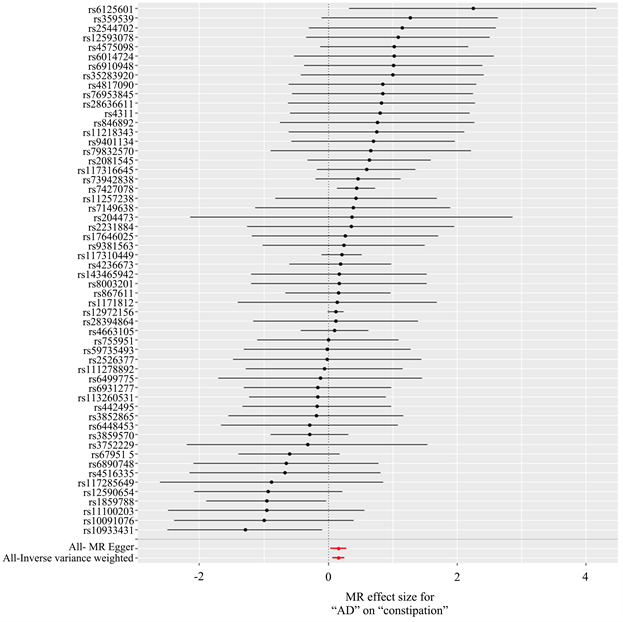
Figure 6. Forest map of results of Mendelian randomization analysis of constipation by AD
图6. AD对便秘孟德尔随机化分析结果森林图
4. 讨论
本研究基于GWAS数据,应用两样本孟德尔随机化方法揭示了便秘与AD之间的因果关系。结果发现,AD与便秘增加存在正向因果关系(IVW: OR = 1.174, 95% CI: 1.068~1.290, P = 0.001),便秘与AD风险增加不存在因果关系(IVW: OR = 1.008, 95% CI: 0.977~1.039, P = 0.629)。在使用不同MR模型进行的敏感性分析中,结果仍然稳健。
目前,AD患者便秘风险增加的原因尚不清楚,其潜在机制仍有待阐明,但有研究推测这可能是多种因素共同作用的结果。其中脑–肠–微生物轴假说可能是其中一种机制;相关研究表明,大脑和肠道之间的双向连接,在肠道疾病和神经系统疾病之间的关联中起着至关重要的作用,肠道功能障碍可通过脑–肠轴影响中枢神经系统,涉及神经、内分泌、免疫等多个方面 [16] 。另外,研究发现肠道菌群可参与
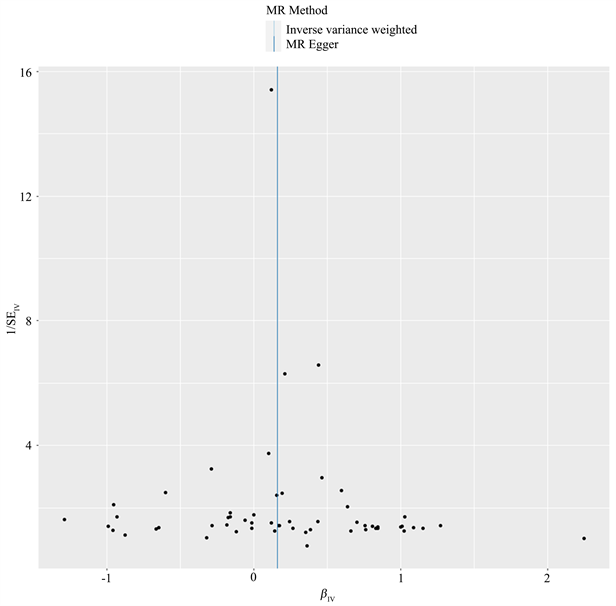
Figure 7. Funnel diagram of the results of Mendelian randomization analysis of constipation by AD
图7. AD对便秘孟德尔随机化分析结果漏斗图
肠道与脑部之间的信号传递,表明肠道微生物组可通过肠–脑轴影响中枢神经系统功能,中枢神经系统也可以同样的方式影响肠道微生物的组成和部分功能,构成“微生物–肠–脑轴”,肠道微生物群在健康和各种疾病模型中肠道和神经系统之间的双向相互作用中起着重要作用 [17] ,其中重要机制包括神经递质(乙酰胆碱、儿茶酚胺、γ-氨基丁酸、组胺等)的合成、免疫系统活性调节、特定代谢物的合成、对色氨酸代谢的影响、微生物群组成的改变以及神经连接等 [18] 。大量动物实验和临床研究发现,阿尔兹海默症患者肠道菌群多样性及菌群丰富度存在改变,提示肠道菌群失调与阿尔兹海默症病理学进程存在一定的相关性 [19] [20] [21] ,比如阿尔茨海默病患者表现出拟杆菌门的丰度增加,厚壁菌门和放线菌门的丰度降低 [22] 。同时,相关研究表明微生物组的改变会诱发便秘 [23] [24] 。另外一个假设是大脑顶叶功能障碍。Aβ学说是阿尔兹海默症发生的主流学说,Aβ过度堆积是AD重要病理标志,是AD发生的最主要病变特征之一 [25] 。而顶叶则被认为与儿童便秘有关 [26] ,并且已知与短期记忆有关 [27] ;相关研究表明,Aβ在内侧顶叶皮层的沉积是AD发展的第一阶段,这也是AD患者最容易发生代谢减退和萎缩的部位 [28] 。
尽管此次研究结果为AD与便秘之间的正相关提供了有力的证据,但也要承认此次研究存在一些限制。第一、在探究AD与便秘的关系时,尽管我们使用了尽可能大的与便秘有关的GWAS数据,但仍只有少数SNP符合条件。为了解决这个问题,我们放宽了统计阈值(P < 5 × 10−6)以满足我们的研究,之后仍然需要通过更大样本的MR研究和随机对照试验来验证我们的结果,当有更大的GWAS数字可用时,我们将需要进一步的研究来证实我们的发现。第二、研究仅考虑了AD与便秘之间的因果关系,而未探究其他潜在因素的影响。AD是一个复杂的病理过程,可能受到多种因素的影响,包括年龄、性别等,进一步的研究可以考虑这些因素,并综合分析它们与便秘的相互作用;第三、本研究只是统计学结果,无法进一步探讨AD与便秘之间的生物学机制。第四、由于我们研究中使用的GWAS数据仅来自于欧洲人群,研究结果不能推广到其他人群。因此,AD对与便秘在其他人群中的效应还有待进一步验证。
综上所述,该研究采用双向两样本孟德尔随机化方法,结果表明AD与便秘风险增加存在正向因果关系,便秘与AD风险增加不存在因果关系。
基金项目
四川省中医药管理局面上项目(2023MS241)。
NOTES
*通讯作者。