1. 前言
乳腺癌(breast cancer)是女性常见的恶性肿瘤之一,占女性癌死亡原因的首位 [1]。增殖是控制乳腺癌发展的重要因素,但目前关于乳腺癌的增殖的机制尚未完全清楚。microRNA是一类非编码小分子RNA,长度为18~26个核酸的内源性小RNA,其通过与靶基因3端非编码区域特异性结合的方式调节靶基因的表达 [2],参与多种生物调节途径,如细胞增殖和凋亡、脂肪代谢等 [3]。最近有研究显示,microRNA可以通过调控癌基因或抑癌基因表达,参与肿瘤细胞增殖等生物学过程 [4] [5] [6]。
本研究在前期对乳腺癌组织测序中发现一批新型microRNA。对这一批新型microRNA进行筛选,发现novel-miR-9 (cggggggcuggcggcggc)对乳腺癌的增殖起到抑制作用。本研究运用生物信息学分析,预测novel-miR-9的靶基因,绘制韦恩图得靶基因集合,找出与增殖相关基因,并对其靶基因集合进行蛋白质互作网络分析,乳腺癌组织表达,GO (Gene Ontology)分析和KEGG Pathway (Kyoto encyclopedia of genes and genomes)分析预测结果中的靶基因集合作用机制,并注释其靶基因的生物学功能,为展开novel-miR-9的靶基因鉴定及生物学功能研究提供理论基础。
2. 材料与方法
1.材料:正常细胞系MCF-10A、人乳腺癌细胞系MCF-7和MDA-MB-231 (中国科学院上海生命科学院细胞库);DMEM高糖培养基和澳洲胎牛血清(Gibco公司);转染试剂Lipofectamine 2000 (Thermo Fisher公司);miDETECT A TrackTM miRNA RT-qPCR Starter Kit和microRNA前后引物以及mimic (广州锐博公司);cck-8 (Biosharp公司);EdU (广州锐博公司);Novel-miR-9测序(华大基因)。
2.细胞培养与分组:DMEM高糖培养基 + 质量分数为10%澳洲胎牛血清 + 质量分数为1%的双抗,置于37℃、5% CO2、饱和湿度的细胞培养箱中培养。无菌操作,3 d传代培养1次。取传2代,对数增殖,状态良好的细胞用于后续实验。将对数增殖的MCF-7和MDA-MB-231细胞收集,均匀铺于6孔板上,5 × 105万/孔,放入37℃、5% CO2、饱和湿度的细胞培养箱中培养。待细胞铺满6孔板后,应用Lipofectamine 2000将mimic及mimic-NC瞬时转染MCF-7与MDA-MB-231细胞。放入37℃、5% CO2、饱和湿度的细胞培养箱中培养6~8 h,将6孔板液体换为完全培养基后继续放入37℃、5% CO2、饱和湿度的细胞培养箱中培养。
3.RNA提取与RT-qPCR检测:用Trizol法提取收集MCF-10A、MCF-7、MDA-MB-231细胞RNA,置于−80℃冰箱保存待检。用RNeasy Maxi试剂盒纯化RNA,在NanoDrop 2000c上测定RNA浓度。RNA样品的A值260/280比1.8~2.1之间用于进一步研究。按反转录试剂盒操作说明书进行操作,将提取的每种细胞的总RNA (1 μg)反转录成cDNA。使用罗氏LightCycler 480 Real-Time PCR系统检测novel-miR-9的表达水平。使用U6作为内参,U6上游引物:5’-CTCGCTTCG GCAGCACA-3’;下游引物:5’-ACGCTTCACGAATT TGCGT-3’。RT-qPCR热循环条件为:第一步:95℃ 10 min;第二步:95℃ 2 s,60℃ 20 s,70℃ 10 s,共40个循环;第三步:融解曲线生成。采用相对定量法,以U6为内参,各种细胞中10个microRNA的水平为它与同样本中的U6的比值为相对表达水平,由公式Flods = 2 − ΔΔCt计算获得,ΔΔCt为10个microRNA的相对表达水平。
4.cck-8和EdU检测增殖:cck-8:对照组和实验组乳腺癌细胞以质量分数为10%的胎牛血清高糖培养基配制成2 × 103个细胞/孔的细胞悬液,96孔板培养(37℃、5% CO2、饱和湿度的细胞培养箱),分别于24、48和72 h后,加入cck-8溶液,每孔10 μL,用全波长酶标仪在450 nm处测量各孔的吸光度值。EdU:对照组和实验组乳腺癌细胞以质量分数为10%的胎牛血清高糖培养基配制成2 × 104个细胞/孔的细胞悬液,24孔板培养(37℃、5% CO2、饱和湿度的细胞培养箱),培养24 h,按照protocol加入EdU试剂,并在正置荧光显微镜拍照。
5.生物信息学网站和软件:利用TargetScan [7] (http://www.targetscan.org/),miRDB [7] (http://mirdb.org/),miRWalk [7] 和miRbase [7] 4个数据库预测novel-miR-9的靶基因;GeneCards (http://www.genecards.org/) 可查询到与乳腺癌相关的致病基因;两者取交集,获得同乳腺癌相关的预测靶基因;运用UCSC [8] (https://xena.ucsc.edu/)在线工具分析靶基因在乳腺癌患者中的差异表达;DAVID6.8数据库 [9] (https://david.ncifcrf.gov/)对预测的靶基因集合进行GO分析和KEGG信号传导通路的富集分析;STRING [10] (http://string-db.org/)在线工具分析靶基因之间的交互作用。
6.统计学分析差异:计量资料用均值±标准差(χ ± s)表示,统计方法采用配对t检验,P < 0.05表明差异具有统计学意义。
3. 结果
1) novel-miR-9在细胞中的表达水平 利用RT-qPCR检测novel-miR-9在正常细胞MCF-10A和人乳腺癌细胞MCF-7、MDA-MB-231中的表达量,发现novel-miR-9在乳腺癌细胞的表达量低于正常细胞(图1)。
***P < 0.001 compared with MCF-10A, MDA-MB-231 NC and MCF-7 NC.
Figure 1. Expression level of novel-miR-9 in cells. (a) Novel-miR-9 in normal cells and in human breast cancer cells; (b) (c) to verify whether novel -miR-9 was introduced into human breast cancer MDA-MB-231 and MCF-7 cells
图1. novel-miR-9在细胞中的表达水平。(a) novel-miR-9中正常细胞MCF-10A和人乳腺癌细胞MCF-7、MDA-MB-231;(b) (c) 验证novel-miR-9是否导入人乳腺癌MDA-MB-231和MCF-7细胞
2) novel-miR-9对乳腺癌细胞增殖影响 将novel-miR-9类似物导入MCF-7和MDA-MB-231细胞,运用cck-8以及EDU检测乳腺癌细胞增殖能力(图2)。
3) 预测novel-miR-9靶基因 通过TargetScan,miRDB,miRWalk和miRanda预测novel-miR-9的靶基因与GeneCard查询到与乳腺癌相关的致病基因取交集得到同乳腺癌相关的1188个预测靶基因(图3)。
(a)
(b)
(c)**P < 0.01, ***P < 0.001 compared with MDA-MB-231 NC and MCF-7 NC
Figure 2. Effects of Novel-miR-9 on the proliferation of breast cancer cells. (a) CCK-8 assayed the effect of Novel -miR-9 on the proliferation of MCF-7 and MDA-MB-231 breast cancer cells; (b) EDU detection of the effect of Novel-miR-9 on the proliferation of MDA-MB-231 breast cancer cells; (c) EDU was used to detect the effect of Novel-miR-9 on the proliferation of breast cancer cells MCF-7
图2. novel-miR-9对乳腺癌细胞增殖影响。(a) cck-8检测novel-miR-9对乳腺癌细胞MCF-7和MDA-MB-231增殖影响;(b) EdU检测novel-miR-9对乳腺癌细胞MDA-MB-231增殖影响;(c) EdU检测novel-miR-9对乳腺癌细胞MCF-7增殖的影响
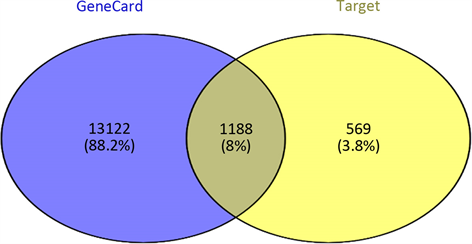
Figure 3. Predicts target genes associated with Novel -miR-9 and breast cancer
图3. 预测novel-miR-9同乳腺癌相关的靶基因
4) 找出与增殖相关靶基因 运用DAVID线上网站找出1188个靶基因中与增殖相关的靶基因。发现BRCA1、CDH1、ERBB2、EGFR等26个基因直接调控细胞增殖(表1)并列出26个基因信息(表2)。
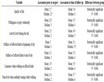
Table 1. GO analysis of DEGs in Breast Cancer
表1. GO分析乳腺癌的差异基因
GO,基因数据库;DEGs,差异表达基因。
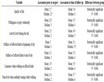
Table 2. Functional roles of 26 genes
表2. 26个基因的功能
5) 增殖相关基因相互作用及乳腺癌组织表达 运用STRING线上网站分析相关基因的交互作用;运用UCSC线上网站分析26个基因在乳腺癌组织中的表达量(图4)。
(a)
(b)
Figure 4. Interaction of 26 genes and their expression levels in breast cancer tissues. (a) Analysis of the interactions of 26 genes by String; (b) the expression levels of 26 genes in breast cancer tissues were analyzed by UCSC
图4. 26个基因相互作用及在乳腺癌组织的表达量。(a)用STRING分析26个基因的相互作用;(b)用UCSC而分析26个基因在乳腺癌组织的表达量
6) 相关基因的GO功能注释及KEGG信号传导通路的富集分析 运用DAVID线上网站分析26个基因的GO功能注释以及KEGG信号传导通路的富集分析(表3)。
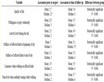
Table 3. GO and KEGG pathway enrichment analysis of 26 Genes
表3. GO和KEGG富集化分析26个基因
GO,基因库;KEGG,基因富集化。
4. 讨论
乳腺癌为临床常见的恶性肿瘤之一,其发病率在不断的升高且有向年轻化发展的趋势,这对乳腺癌的临床防治造成极大的困难。近年来,miRNA在癌症中的作用机制已经成为研究热点,基因芯片有助于探究miRNA在多种癌症中的表达模式 [11]。多项研究表明,miRNA参与细胞生长、增殖、分化和凋亡等多个生理过程 [12] [13],在癌症发生和发展中有重要作用,甚至能够预测肿瘤分类 [14]。
miRNA主要是通过结合mRNA的3'UTR区,转录后调控靶基因 [15],其体现在一方面可与沉默核糖蛋白复合物结合,通过序列完全互补的方式降解目的蛋白,另一方面可通过非完全互补结合发挥转录后调控。因此明确microRNA作用的靶基因尤为重要。
运用生物信息学软件预测miRNA靶基因,已经成为生物学研究的一种重要手段。现已开发出多种miRNA靶基因预测软件,但不同软件的算法不同,优缺点也有所侧重。本研究使用TargetScan,miRDB,miRWalk和miRbase在线分析软件进行靶基因的预测,由于结合了多个靶基因预测软件的结果,大大排除了假阳性的影响,准确性增高。近年来将Genecards数据库查询到的疾病相关因子的调控网络与生物信息学软件所获基因进行联合,为后续实验验证提供大量非常有价值的线索 [16]。本研究将Genecards数据库中与乳腺癌相关的基因同预测出的潜在靶基因交集,共获得1188个基因;通过DAVID数据库GO功能分析所获得的1188个基因,得到与增殖相关基因26个,包括EGFR、IGF1R、IGFBP2等。其中可以看出在乳腺癌明显高表达的有LTBP4、SCN5A、HAP1、EGFR、NTRK2、NGFR和ENG;明显低表达的有RHBDF2、IGFALS、COL5A1、COL1A1、ERBB2。通过STING分析26个蛋白相互作用,发现ERBB2、EGFR、COL1A1、COL5A1在26个基因中起着关键作用。
综上所述,生物信息学在线分析软件所获得的信息能够在miRNA与疾病发生相关的研究中发挥很好的前瞻性作用。本研究对Novel-miR-9进行增殖实验、靶基因预测、GO富集分析和KEGG通路富集分析,结果表明在乳腺癌发病过程中Novel-miR-9可能通过调控多个与乳腺癌发病相关的靶基因,并可能参与多条信号通路的调节,从而在乳腺癌中发挥抑癌基因的作用。通过对Novel-miR-9的靶基因预测,我们有机会在这些与增殖相关的26个靶基因中,找到能被Novel-miR-9靶向调节的基因。从而可以进一步对该microRNA对人乳腺癌细胞增殖影响的机制研究,为将来逆转乳腺腺癌的化疗耐受提供了新的靶点。