1. 引言
区间值直觉模糊集的概念由Atanassov和Gargov [1] 在1989年提出,他将每个元素对模糊集的隶属度和非隶属度扩展到一个[0, 1]之间的区间中,使其能够包含更多的不确定性信息。接着,Atanassov [2] 为区间值直觉模糊集定义了一些基础性质和运算法则。至此,区间值直觉模糊集受到了广泛的关注,并吸引了大量的学者对它进行研究。理所当然,存在于直觉模糊集理论中的距离测度,相似度等研究也深入到区间值直觉模糊集环境中。
相似度是模糊集理论中的一个重要测度,负责计算两个模糊集间的相似程度,在决策问题中可以替代距离测度使用,因而具有重要的研究意义。下面是一些学者所做的研究,Xu [3] [4] 在2007年引入了区间值直觉模糊相似度的概念以及一些距离测度。2009年,Xu和Yager [5] 研究了和区间值直觉模糊环境下的偏好关系和相似度。当两个模糊集的隶属度和非隶属度之间差异不大但犹豫度差异很大时,犹豫指数就起着非常重要的作用。Xu和Xia [6] 提出的新的相似度就考虑了犹豫度的问题,克服了之前相似度的缺陷。同样的,Wu等人 [7] 也提出了考虑犹豫度的区间值直觉模糊相似度并应用于专家系统问题之中。
在之后的研究中,各研究者提出了更多的区间值直觉模糊相似度。Singh [8] 为区间值直觉模糊集开发了一种新的余弦相似度,并将其应用于模式识别。Luo和Liang [9] 等人开发了一种新的相似度来解决区间值直觉模糊环境下的多准则决策问题。Jeevaraj [10] 提出了一种新的基于非犹豫得分函数的区间值直觉模糊数的相似度,并将其应用于解决模式重组问题。Verma和Merigo [11] 提出了一种基于加权约简直觉模糊集的区间值直觉模糊余弦相似度,并定义了准有序加权区间值直觉模糊余弦相似度。Tiwari和Gupta [12] 开发了区间值直觉模糊软集的距离、相似度和熵。Rathnasabapathy和Palanisami [13] 为区间值直觉模糊集设计了余弦相似度,应用于现实世界的决策问题,如模式识别、医疗诊断。Suo [14] 建立了一个知识测度函数,将距离与基于理想解的相似度排序偏好技术相结合。Chen和Ke [15] 提出了余弦相似度,利用区间值直觉模糊知识解决多准则决策问题。Nayagam [16] 等人提出了一种基于传统梯形值直觉模糊集准确得分计算的相似度。
从以往的区间值直觉模糊相似度的相关文献和研究来看,已经提出的相似度大多存在着反直觉的情况,无法区分某些模糊集的相似程度。因此,本文从熵测度的角度出发,基于熵测度和相似度之间的相关关系,利用一个双参数熵提出了一个新的区间值直觉模糊相似度,证明其满足区间值直觉模糊相似度的公理化定义。之后,通过一个简单实例,说明其优越性和有效性。最后将其与VIKOR方法相结合,提出了一个解决多属性决策问题的新方法,应用于多属性决策问题中。
2. 预备知识
定义1 [1] 设X是一个非空有限集合,那么
是定义在X上的一个区间值直觉模糊集(Interval-valued intuitionistic fuzzy set, IvIFS),其中
,
分别表示
对于集合A的隶属度和非隶属度,满足对任意
,
。
特别的,当
,
时,区间值直觉模糊集A退化为直觉模糊集。
此外,
表示元素
对于集合A的犹豫度。
为了方便后续研究,称
为区间值直觉模糊数(IvIFN),其中
,
,
。
定义2 [2] 设
和
是两个IvIFSs,那么
1)
;
2)
;
3)
;
4)
;
5)
;
6)
;
定义3 [17] 一个实值函数
叫做区间值直觉模糊集的相似度,如果满足以下条件:
S1)
;
S2)
当且仅当
;
S3)
;
S4) 如果
,那么
且
。
定义4 [17] 一个实值函数
叫做区间值直觉模糊集的熵,如果满足以下条件:
E1)
当且仅当A是一个清晰集;
E2) 对任意
,
当且仅当
;
E3)
;
E4) 对任意
,若当
时,
且
或
当
时,
且
,都有
,则称
为IvIFS A的熵。
3. 新的区间值直觉模糊相似度
文献 [18] 中提出了一个区间值直觉模糊熵
。 (1)
其中A是一个IvIFS,且
满足定义4。
Wei等人 [17] 定义了一些区间值直觉模糊熵和相似度之间的相关关系,通过这些相关关系使得熵和相似度可以相互导出,借助该方法,本节通过(1)式构造出一个新的相似度。
3.1. 新的相似度及其证明
首先做如下定义:
定义5设A和B是
中的两个IvIFSs,
;
,由A和B定义的一个新的区间值模糊集
,如下:
,
,
,
。
则
,
。
。
定理1
是区间值直觉模糊集A和B一个相似度,其中
是(1)式中的熵。
证明:为了证明该定理,需验证定义(3)中的四个条件。
S1) 因为对任意
,
。又
,所以
。
S2) 根据熵
和
的定义可得,对任意
,
,
,
,
,
,
,
。
S3) 由定义(5)可得,显然
,于是
。
S4) 设A,B,C是三个区间值直觉模糊集且
,那么
,
。
可得
,
,
,
。
于是有
, (2)
, (3)
, (4)
。 (5)
然后可得
,
,
,
,
因此
。此外,由(2)~(5)式还可得出
,
。再根据定义4的条件(E4),就有
。同理可得,
。
综上所述,
是一个有效的区间值直觉模糊熵。
例1计算集合X中两个区间值直觉模糊集
和
的相似度。其中参数
,结果见表1
,
,
,
。于是
。
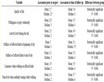
Table 1. Values of the proposed similarity measure
表1. 新的相似度的计算结果
3.2. 与现存相似度的比较分析
3.2.1. 一些现存相似度
1) Xu和Chen [19] 的相似度:
。 (6)
2) Wei等人 [17] 的相似度:
。 (7)
其中,
,
,
,
。
3) Luo和Liang [9] 的相似度:
(8)
3.2.2. 比较分析
例2考虑三个区间值直觉模糊集
,
,
。
,
计算
和
的相似度结果展示在表2;
,
计算
和
的相似度结果展示在表3;
,
计算
和
的相似度结果展示在表4。
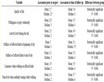
Table 2. Values of ξ ( α , β ) ( ψ ) and S X C
表2.
和
的计算结果
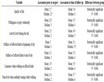
Table 3. Values of ξ ( α , β ) ( ψ ) and S W
表3.
和
的计算结果
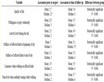
Table 4. Values of ξ ( α , β ) ( ψ ) and S L L
表4.
和
的计算结果
从表2至表4可得,
,
,
无法区分区间值直觉模糊集之间的相似度,而我们提出的相似度能够有效区分。
4. 基于新的相似度的VIKOR方法
4.1. 方法步骤
第一步:建立决策矩阵
。假设
是需要解决决策问题的备选方案的集合,
是备选方案的属性的集合,那么该问题的决策矩阵为
其中
是第i个方案的第j个属性的评价值
,
。
第二步:计算标准决策矩阵
。
第三步:计算正理想解
和负理想解
。
,
。其中
,
,
,
,
,
,
,
,
,
。
第四步:基于(1)式的熵确定客观属性权重。
首先计算决策矩阵每一个属性的熵值。
,
。
然后计算每个属性的散度。
,
最后进行归一化的到属性权重。
,
第五步:基于新提出的相似度
计算效用指标
和遗憾指标
:
。
第六步:计算VIKOR指数
:
。
其中
,
,
,
。
为“大多数准则”策略的权重或最大群体效用权重。
时,表示根据大多数人的意见制定决策:
时,表示根据赞同的情况制定决策;
时,表示根据拒绝的情况制定决策。
第七步:对方案排序。根据
,
,
的值对方案排序,值越小越好,共得到三种排序结果。
第八步:找出折衷方案。当下面两个条件满足时,可以根据
的值对方案排序。假设
是按照
的值排序第一的方案,它的值为
。
条件1:可接受优势。
。
其中
为排序第二的方案
的值,m为备选方案个数。
条件2:决策过程中可接受的稳定性。根据
值的第一排序方案
必须是
和
的值的第一排序方案。
如果以上条件有一个不满足,将得到一个折衷解集。折衷解包含两个部分:
(a) 如果不满足条件2,则
,
均为折衷解。
(b) 如果不满足条件1,通过
得到最大的k,
均为折衷解。
4.2. 实例分析
考虑一个Hu [20] 提出的数值例子。一家制造电子产品的高科技公司计划评估和选择一个USB连接器供应商。有四家供应商
和
被选中。我们用四个属性来对它们进行评估:
是财务,
是绩效,
是技术,
是组织文化。决策者使用区间值直觉模糊数对四个方案
的四种属性
进行评价。
第一步:建立决策矩阵
如表5所示。
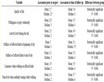
Table 5. The decision matrix R z ˜ i j *
表5. 决策矩阵
第二步:计算标准决策矩阵
。因为所有属性都是效益属性,所以
。
第三步:计算正理想解
和负理想解
,结果见表6。
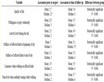
Table 6. The positive and negative idea solutions
表6. 正负理想解
第四步:确定客观属性权重。结果见表7。
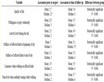
Table 7. Values of ξ , W and ω
表7.
,W and
的值
第五步:效用指标
和遗憾指标
的计算结果展示在表8。
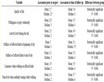
Table 8. Values of S i and R i
表8.
和
的计算结果
第六步:VIKOR指数
计算结果展示在表9。
第七步:根据表8和表9可以清楚得到通过
、
对方案的排序结果都为
。
第八步:找出折衷方案。
首先计算
,不满足条件1,满足条件2,于是计算
得到折衷解
和
。
将我们使用的方法和以下几个方法进行比较:
1) Zhang [21] 提出的基于余弦交叉熵的拓展的TOPSIS方法。
2) Narayanamoorthy [22] 提出的区间直觉犹豫模糊VIKOR方法。
3) Hu [20] 提出的基于IVIFGWHM算子的决策方法。
结果展示在表10。
从表10中可以看出Zhang和Narayanamoorthy的方法和我们提出的方法得到的结果相同,与Hu的结果相反。方法中的不同之处在于Zhang和Narayanamoorthy选择的正负理想解为
和
,不会随评价值的改变而改变。Zhang使用一个线性规划模型计算属性权重,Narayanamoorthy和我们基于熵确定属性权重但Hu得属性权重是由专家直接给出。在计算正负理想解和备选方案之间的差异程度时,Narayanamoorthy使用的是归一化Hamming距离测度,而我们使用的是新提出的相似度,更加有效。总的来说,我们提出的新方法是有效的且具有一定的优越性。
5. 结论
本文考虑了区间值直觉模糊环境下的相似度,针对现存的相似度存在反直觉,无法准确计算模糊集合相似程度的问题,基于区间值直觉模糊熵导出了一个新的区间值直觉模糊相似度,去克服现存相似度的缺陷,并证明了新的相似度满足相应的公理化定义。其次,运用一个实例将新的相似度与几个现存相似度进行比较分析,凸显其优越性。最后,将新的相似度与VIKOR方法相结合,提出了解决多属性决策问题的新方法,并将其应用于一个现实例子中,说明其有效性。未来的研究可以考虑将新的相似度应用于其它决策方法,并在其中考虑加入犹豫度的问题。